from scipy.cluster.hierarchy import dendrogram, linkage from matplotlib import pyplot as plt X = [[i] for i in [2, 8, 0, 4, 1, 9, 9, 0]] Z = linkage(X, 'average') fig = plt.figure(figsize=(6,3)) # 控制图的大小 dn = dendrogram(Z) plt.rcParams["font.sans-serif"]=["SimHei"] plt.title('实验一') plt.xlabel('聚类谱系图') plt.ylabel('聚类水平') plt.show()
时间: 2024-04-02 20:37:04 浏览: 17
这段代码使用了Python的SciPy库来进行层次聚类,并使用matplotlib库进行可视化。具体来说,代码的功能如下:
1. 定义一个长度为8的一维数组X,数组中的元素分别为2, 8, 0, 4, 1, 9, 9, 0。
2. 调用linkage()函数进行层次聚类,其中'average'表示使用平均链接合并策略。聚类得到的结果保存在变量Z中。
3. 创建一个大小为(6,3)的图形对象fig。
4. 对聚类树进行可视化,使用dendrogram()函数绘制聚类树,并将聚类树绘制在变量dn中。
5. 设置字体为中文显示,设置图像标题为'实验一',x轴标签为'聚类谱系图',y轴标签为'聚类水平'。
6. 显示图像。
总的来说,这段代码实现了对一维数据进行层次聚类,并将聚类结果以树状图的形式可视化。可以根据需要修改代码中的数据、聚类合并策略和图像参数等。
相关问题
import numpy as np from scipy.cluster.hierarchy import dendrogram, linkage import matplotlib.pyplot as plt # 生成一个距离矩阵 X = np.array([[0, 1], [0.4, 1], [0.7, 3], [1, 1], [1.5, 2]]) # 使用Ward方法进行层次聚类 Z = linkage(X, 'ward') # 绘制树状图 fig = plt.figure(figsize=(5, 3)) dn = dendrogram(Z) plt.show()这段代码中的ward方法是什么
在这段代码中,`ward` 是 `scipy.cluster.hierarchy.linkage` 函数的一个参数,它代表层次聚类算法的链接方法之一。Ward方法是一种凝聚式聚类算法,它的特点是将两个群集合并时,合并后的群集的平方误差和最小。在实际应用中,Ward方法常用于处理凸形的数据集,它能够较好地保持群集的凸性。
scipy.cluster.hierarchy.dendrogram函数实现鸢尾花数据集分裂聚类并可视化
好的,下面是一个使用`scipy.cluster.hierarchy.dendrogram`函数实现鸢尾花数据集分裂聚类并可视化的示例代码:
```python
import numpy as np
import matplotlib.pyplot as plt
from scipy.cluster.hierarchy import dendrogram, linkage
from sklearn.datasets import load_iris
# 载入鸢尾花数据集
iris = load_iris()
X = iris.data
y = iris.target
# 计算样本间的距离矩阵
Z = linkage(X, method='ward')
# 绘制聚类树
plt.figure(figsize=(10, 7))
plt.title('Hierarchical Clustering Dendrogram')
plt.xlabel('Sample Index')
plt.ylabel('Distance')
dendrogram(
Z,
leaf_rotation=90., # 旋转x轴标签
leaf_font_size=8., # x轴标签字体大小
)
plt.show()
```
上述代码首先使用`load_iris`函数载入鸢尾花数据集,并计算样本间的距离矩阵。然后,使用`dendrogram`函数绘制聚类树,并设置x轴标签旋转角度和字体大小。最后,使用`plt.show()`函数显示可视化结果。
注:上述代码使用了`method='ward'`来进行层次聚类,也可以使用其它距离度量和聚类方法进行分裂聚类。
相关推荐
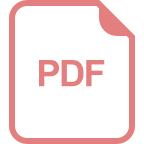
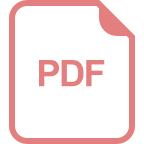
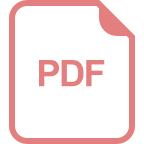












