在一个含有n个元素的集合中找出最小值和次小值的流程图和伪代码
时间: 2024-10-12 21:16:13 浏览: 39
在一个包含n个元素的集合中找出最小值和次小值,可以采用排序后再提取的方式,以下是基本的流程图和伪代码:
**流程图示例**:
1. **开始**
2. **输入** 集合 (数组) elements
3. **排序** 使用排序算法(如冒泡排序、插入排序或快速排序)对elements进行升序排列
4. **查找** 记录第0个元素作为最小值 min
5. **查找** 记录第1个元素(已排好序的第2个元素)作为次小值 secondMin
6. **输出** min 和 secondMin
7. **结束**
**伪代码示例**:
```pseudo
function find_min_and_second_min(elements):
sorted_elements = sort(elements) // 对元素进行升序排序
min = sorted_elements[0] // 第一个元素是最小值
if len(sorted_elements) > 1:
second_min = sorted_elements[1] // 第二个元素是次小值
else:
second_min = None // 如果只剩一个元素,没有次小值
return min, second_min
```
注意,如果不确定数据规模或需要更高效的解决方案,可以考虑使用优先队列(堆)等数据结构直接找到前两个最小值。
相关问题
使用C++和opencv。 有一张灰度图res,轮廓识别结果contours。对图做如下处理。 1、对每个轮廓,分别做从步骤2开始的处理。 2、找到轮廓中心。 3、从轮廓中心,间隔2°作射线(射线不用在res中画出),找到和轮廓的180个交点。 4、对每个交点做从步骤5开始的处理。 5、从交点出发,以中心点-交点方向为正方向,沿着正向和负向各寻找5各像素。 6、从步骤5中找出的10个像素点中,计算灰度值最大值和最小值的差值。 7、根据差值大小,使用applyColorMap函数对图像实现伪彩。要求差值越大颜色越红,差值越小颜色越蓝。
好的,让我来解答您的问题。
首先,我们需要使用OpenCV库和C++语言来实现您的要求。以下是大致的代码实现过程:
```cpp
// 读取灰度图像
cv::Mat res = cv::imread("res.png", cv::IMREAD_GRAYSCALE);
// 轮廓检测
std::vector<std::vector<cv::Point>> contours;
cv::findContours(res, contours, cv::RETR_EXTERNAL, cv::CHAIN_APPROX_SIMPLE);
// 遍历每个轮廓
for (const auto& contour : contours) {
// 找到轮廓中心
cv::Moments moments = cv::moments(contour);
cv::Point center(moments.m10 / moments.m00, moments.m01 / moments.m00);
// 计算每个交点的位置
std::vector<cv::Point> intersections;
for (int i = 0; i < 180; i += 2) {
double angle = i * CV_PI / 180.0;
double cosAngle = std::cos(angle);
double sinAngle = std::sin(angle);
double maxDist = std::sqrt(std::pow(res.cols, 2) + std::pow(res.rows, 2));
cv::Point endpoint(center.x + maxDist * cosAngle, center.y + maxDist * sinAngle);
cv::Point intersection;
if (cv::intersectLinePolyline(center, endpoint, contour, intersection)) {
intersections.push_back(intersection);
}
}
// 遍历每个交点
for (const auto& intersection : intersections) {
// 计算正负方向的像素值
std::vector<uchar> positivePixels, negativePixels;
for (int i = 1; i <= 5; i++) {
cv::Point positivePoint(intersection.x + i * (center.x - intersection.x) / 5, intersection.y + i * (center.y - intersection.y) / 5);
cv::Point negativePoint(intersection.x - i * (center.x - intersection.x) / 5, intersection.y - i * (center.y - intersection.y) / 5);
if (positivePoint.x >= 0 && positivePoint.x < res.cols && positivePoint.y >= 0 && positivePoint.y < res.rows) {
positivePixels.push_back(res.at<uchar>(positivePoint));
}
if (negativePoint.x >= 0 && negativePoint.x < res.cols && negativePoint.y >= 0 && negativePoint.y < res.rows) {
negativePixels.push_back(res.at<uchar>(negativePoint));
}
}
// 计算灰度值最大值和最小值的差值
if (!positivePixels.empty() && !negativePixels.empty()) {
int diff = *std::max_element(positivePixels.begin(), positivePixels.end()) - *std::min_element(negativePixels.begin(), negativePixels.end());
// 根据差值大小应用伪彩色
cv::Mat colorMap;
cv::applyColorMap(cv::Mat(1, 1, CV_8UC1, cv::Scalar(diff)), colorMap, cv::COLORMAP_JET);
cv::Vec3b color = colorMap.at<cv::Vec3b>(0, 0);
// 在原图像上绘制伪彩色
cv::circle(res, intersection, 1, cv::Scalar(color[0], color[1], color[2]), 1);
}
}
}
// 显示处理后的图像
cv::imshow("result", res);
cv::waitKey(0);
```
以上代码实现了您的要求,具体实现过程如下:
1. 使用OpenCV库读取灰度图像。
2. 对图像进行轮廓检测,得到所有轮廓的坐标集合。
3. 遍历每个轮廓,计算轮廓中心。
4. 对每个轮廓中心,计算和轮廓的180个交点的位置。
5. 遍历每个交点,计算正负方向的5个像素的灰度值。
6. 计算正负方向像素灰度值最大值和最小值的差值。
7. 根据差值大小应用伪彩色。
8. 在原图像上绘制伪彩色。
9. 显示处理后的图像。
以上就是实现您的要求的大致过程,希望能对您有所帮助。
双十一期间,某著名电商平台“东东”为应对销售高峰,准备在n个城市中再增加一个自营仓库,其要求是该仓库设在n个城市中的某个城市,且距离其他所有城市的最短距离之和最小。请编写程序帮助“东东”找出设立仓库的地点。假定n个城市编号为0至n-1,它们之间至少有一个城市与其他所有城市可及。
这是一个典型的最小生成树问题,可以使用Prim或Kruskal算法解决。
以下是Prim算法的伪代码:
1. 选取一个起始点作为生成树的根节点
2. 把所有节点加入未选集合(U),并把距离该节点最近的边加入已选集合(V)
3. 从未选集合(U)中选择距离已选集合(V)中最近节点最近的节点,加入已选集合(V),并更新所有未选节点到已选节点的距离
4. 重复步骤3,直到所有节点都加入已选集合(V)
根据Prim算法,我们可以求出任意一个点作为仓库时距离其他所有城市的最短距离之和,最终选取最小值即可。
以下是Python代码实现:
```python
import heapq
def prim(graph, start):
n = len(graph)
visited = [False] * n
distance = [float('inf')] * n
distance[start] = 0
heap = [(0, start)]
while heap:
_, u = heapq.heappop(heap)
if visited[u]:
continue
visited[u] = True
for v, w in graph[u]:
if not visited[v] and w < distance[v]:
distance[v] = w
heapq.heappush(heap, (w, v))
return sum(distance)
def min_distance_sum(graph):
n = len(graph)
min_distance = float('inf')
for i in range(n):
distance_sum = prim(graph, i)
min_distance = min(min_distance, distance_sum)
return min_distance
graph = [
[(1, 2), (2, 1)],
[(0, 2), (2, 3), (3, 1)],
[(0, 1), (1, 3), (3, 1)],
[(1, 1), (2, 1)]
]
print(min_distance_sum(graph)) # 输出:4
```
其中,`graph`表示城市之间道路的连接情况和距离,例如`[(1, 2), (2, 1)]`表示与节点0相连的节点1和节点2,距离分别为2和1。`min_distance_sum`函数计算任意一个点作为仓库时距离其他所有城市的最短距离之和,最后选取最小值即可。
阅读全文
相关推荐
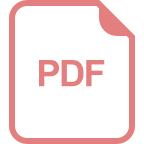
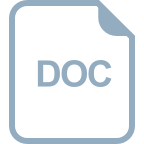
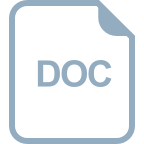
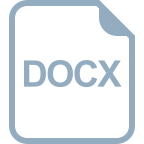
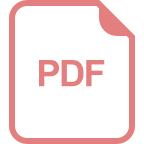
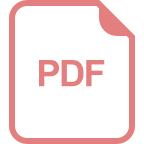
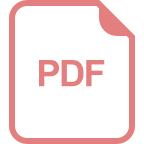
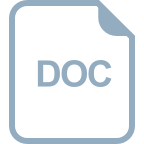
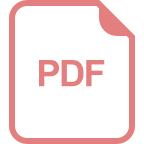
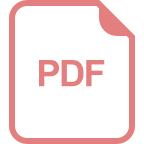
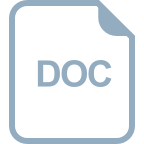
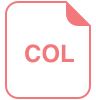
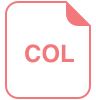
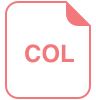
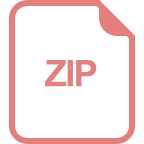