Expected index [8192, 1] to be smaller than self [32, 31] apart from dimension 1 and to be smaller size than src [32, 31]
时间: 2024-04-19 22:24:01 浏览: 14
这个错误通常发生在使用 `scatter_` 方法时,目标索引超出了张量的维度范围。根据错误信息,它表明目标索引的形状为 `[8192, 1]`,而张量的形状为 `[32, 31]`。
这可能是因为目标索引的范围超出了张量的维度范围,或者目标索引的维度与张量的维度不匹配。
要解决这个问题,可以检查以下几个方面:
1. 确保目标索引的大小不超过张量的维度大小。在这种情况下,目标索引的值应该在 `0` 到 `31`(或者维度大小减去 `1`)之间。
2. 检查目标索引的形状是否与张量的形状兼容。在这种情况下,目标索引应该具有与张量匹配的维度大小。
3. 如果目标索引是从其他地方计算得出的,请确保计算目标索引的方式正确,并且产生了符合预期的形状和范围。
请仔细检查你的代码,并确保目标索引的正确性和一致性,以解决这个问题。如果有需要,请提供更多关于你使用 `scatter_` 方法的代码,以便我可以帮助进一步排查问题。
相关问题
RuntimeError: Size does not match at dimension 2 expected index [1, 1, 4694272] to be smaller than src [1, 704, 1667] apart from dimension 1
这个错误通常是由于尝试在一个形状不匹配的张量上执行操作导致的。根据错误信息,您正在尝试使用一个形状为[1, 1, 4694272]的张量,但是您的输入张量大小为[1, 704, 1667],这两个张量的形状不同,因此您需要检查您的代码并确保在执行操作之前,两个张量的形状是匹配的。
您可以检查代码中的数据加载和处理部分是否有错误,或者检查模型的输入和输出是否正确。同时,推荐使用PyTorch的调试工具,例如 `torch.set_grad_enabled(True)` 和 `torch.autograd.set_detect_anomaly(True)` 来帮助您发现代码中的错误。
pytorch部分代码如下:train_loss, train_acc = train(model_ft, DEVICE, train_loader, optimizer, epoch,model_ema) for batch_idx, (data, target) in enumerate(train_loader): data, target = data.to(device, non_blocking=True), Variable(target).to(device,non_blocking=True) # 3、将数据输入mixup_fn生成mixup数据 samples, targets = mixup_fn(data, target) # 4、将上一步生成的数据输入model,输出预测结果,再计算loss output = model(samples) # 5、梯度清零(将loss关于weight的导数变成0) optimizer.zero_grad() # 6、若使用混合精度 if use_amp: with torch.cuda.amp.autocast(): # 开启混合精度 loss = torch.nan_to_num(criterion_train(output, targets)) # 计算loss scaler.scale(loss).backward() # 梯度放大 torch.nn.utils.clip_grad_norm_(model.parameters(), CLIP_GRAD) if not (self._backward_hooks or self._forward_hooks or self._forward_pre_hooks or _global_backward_hooks or global_forward_hooks or global_forward_pre_hooks): return forward_call(*input, **kwargs) class LDAMLoss(nn.Module): def init(self, cls_num_list, max_m=0.5, weight=None, s=30): super(LDAMLoss, self).init() m_list = 1.0 / np.sqrt(np.sqrt(cls_num_list)) m_list = m_list * (max_m / np.max(m_list)) m_list = torch.cuda.FloatTensor(m_list) self.m_list = m_list assert s > 0 self.s = s self.weight = weight def forward(self, x, target): index = torch.zeros_like(x, dtype=torch.uint8) target = torch.clamp(target, 0, index.size(1) - 1) index.scatter(1, target.data.view(-1, 1).type(torch.int64), 1) index = index[:, :x.size(1)] index_float = index.type(torch.cuda.FloatTensor) batch_m = torch.matmul(self.m_list[None, :], index_float.transpose(0,1)) batch_m = batch_m.view((-1, 1)) x_m = x - batch_m output = torch.where(index, x_m, x) return F.cross_entropy(self.s*output, target, weight=self.weight) 报错:RuntimeError: Expected index [112, 1] to be smaller than self [16, 7] apart from dimension 1 帮我看看如何修改源代码
根据您提供的代码,出现报错的地方是:
```
index.scatter(1, target.data.view(-1, 1).type(torch.int64), 1)
```
报错的内容是:`Expected index [112, 1] to be smaller than self [16, 7] apart from dimension 1`,这说明了 `index` 和 `target` 的形状不匹配,导致了错误。
可以尝试以下修改:
1. 查看数据集的类别数是否匹配,即 `cls_num_list` 的长度是否等于类别数。如果不匹配,需要重新计算 `cls_num_list`。
2. 修改 `target` 的形状,使其与 `index` 的形状匹配。可以使用 `target.unsqueeze(1)` 将 `target` 的形状改为 `(batch_size, 1)`。修改后的代码如下:
```
index.scatter(1, target.unsqueeze(1).type(torch.int64), 1)
```
如果您的问题仍然没有解决,可以提供更多的信息,我会尽力帮助您解决问题。
相关推荐
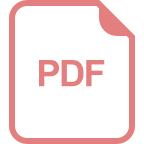
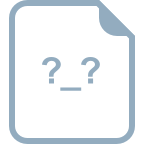
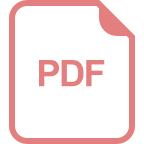







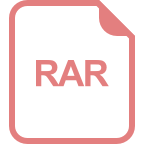
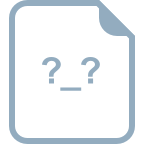
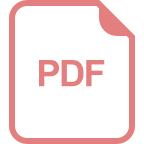
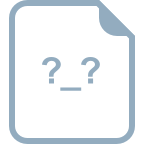
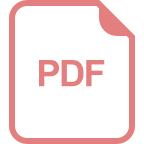
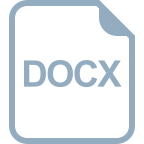