帮我看看这段代码报错原因: Traceback (most recent call last): File "/home/bder73002/hpy/ConvNextV2_Demo/train+.py", line 274, in <module> train_loss, train_acc = train(model_ft, DEVICE, train_loader, optimizer, epoch,model_ema) File "/home/bder73002/hpy/ConvNextV2_Demo/train+.py", line 48, in train loss = torch.nan_to_num(criterion_train(output, targets)) # 计算loss File "/home/bder73002/anaconda3/envs/python3.9.2/lib/python3.9/site-packages/torch/nn/modules/module.py", line 889, in _call_impl result = self.forward(*input, **kwargs) File "/home/bder73002/hpy/ConvNextV2_Demo/models/losses.py", line 38, in forward index.scatter_(1, target.data.view(-1, 1).type(torch.LongTensor), 1) RuntimeError: Expected index [128, 1] to be smaller than self [16, 8] apart from dimension 1 部分代码如下:cls_num_list = np.zeros(classes) for , label in train_loader.dataset: cls_num_list[label] += 1 criterion_train = LDAMLoss(cls_num_list=cls_num_list, max_m=0.5, s=30) class LDAMLoss(nn.Module): def __init__(self, cls_num_list, max_m=0.5, weight=None, s=30): super(LDAMLoss, self).__init__() m_list = 1.0 / np.sqrt(np.sqrt(cls_num_list)) m_list = m_list * (max_m / np.max(m_list)) m_list = torch.cuda.FloatTensor(m_list) self.m_list = m_list assert s > 0 self.s = s self.weight = weight def forward(self, x, target): index = torch.zeros_like(x, dtype=torch.uint8) # index.scatter_(1, target.data.view(-1, 1), 1) index.scatter_(1, target.data.view(-1, 1).type(torch.LongTensor), 1) index_float = index.type(torch.cuda.FloatTensor) batch_m = torch.matmul(self.m_list[None, :], index_float.transpose(0,1)) batch_m = batch_m.view((-1, 1)) x_m = x - batch_m output = torch.where(index, x_m, x) return F.cross_entropy(self.s*output, target, weight=self.weight)
时间: 2023-12-01 11:04:12 浏览: 108
这个错误是由于 `index` 张量的大小与 `output` 张量的大小不匹配导致的。在你的代码中,`index` 张量的大小是 `[batch_size, classes]`,即每个样本的预测标签的 one-hot 编码,而 `output` 张量的大小是 `[batch_size, features]`,即每个样本的特征向量的大小。因此,如果在 `index.scatter_()` 操作中使用了一个大小为 `[batch_size, 1]` 的张量,则会导致上述错误。
要解决这个问题,你可以将 `index` 的大小更改为 `[batch_size, num_classes]`,其中 `num_classes` 是分类数量。你可以在 `LDAMLoss` 的 `__init__` 方法中将 `num_classes` 作为参数并存储在实例变量中,然后在 `forward` 方法中使用它来创建 `index` 张量。例如:
```
class LDAMLoss(nn.Module):
def __init__(self, cls_num_list, max_m=0.5, weight=None, s=30, num_classes=10):
super(LDAMLoss, self).__init__()
self.num_classes = num_classes
m_list = 1.0 / np.sqrt(np.sqrt(cls_num_list))
m_list = m_list * (max_m / np.max(m_list))
m_list = torch.cuda.FloatTensor(m_list)
self.m_list = m_list
assert s > 0
self.s = s
self.weight = weight
def forward(self, x, target):
index = torch.zeros_like(x, dtype=torch.uint8)
index.scatter_(1, target.data.view(-1, 1).type(torch.LongTensor), 1)
index = index[:, :self.num_classes] # 取前 num_classes 列
index_float = index.type(torch.cuda.FloatTensor)
batch_m = torch.matmul(self.m_list[None, :], index_float.transpose(0,1))
batch_m = batch_m.view((-1, 1))
x_m = x - batch_m
output = torch.where(index, x_m, x)
return F.cross_entropy(self.s*output, target, weight=self.weight)
```
然后在使用 `LDAMLoss` 时,你需要将 `num_classes` 参数传递给它。例如:
```
num_classes = len(train_loader.dataset.classes)
criterion_train = LDAMLoss(cls_num_list=cls_num_list, max_m=0.5, s=30, num_classes=num_classes)
```
阅读全文
相关推荐
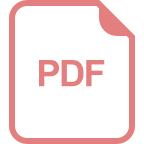
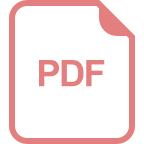

















