pytorch 残差网络
时间: 2023-09-04 16:14:55 浏览: 56
PyTorch中的残差网络(Residual Networks)是一种深度学习网络架构,旨在解决深度神经网络训练过程中的梯度消失和梯度爆炸问题。它通过引入残差连接来解决这些问题。
在PyTorch中,可以使用torchvision.models模块中的ResNet类来实现残差网络。ResNet类提供了多个预定义的深度和宽度不同的残差网络模型,如ResNet-18、ResNet-34、ResNet-50等。
以下是一个使用PyTorch构建和训练ResNet网络的示例代码:
```python
import torch
import torch.nn as nn
import torchvision.models as models
# 构建ResNet模型
model = models.resnet50(pretrained=False)
# 替换最后一层全连接层
num_features = model.fc.in_features
model.fc = nn.Linear(num_features, num_classes)
# 定义损失函数和优化器
criterion = nn.CrossEntropyLoss()
optimizer = torch.optim.SGD(model.parameters(), lr=l
相关问题
pytorch残差网络
PyTorch中的残差网络(Residual Networks)是一种深度学习模型架构,旨在解决深层神经网络的梯度消失和梯度爆炸问题。它通过引入残差连接,允许信息在网络中直接跨层传播。
在PyTorch中,可以使用torchvision模块中的预定义模型来构建和训练残差网络。torchvision提供了许多经典的深度学习模型,包括ResNet。
以下是使用PyTorch构建和训练ResNet的一般步骤:
1. 导入必要的库:
```python
import torch
import torch.nn as nn
import torchvision.models as models
```
2. 定义ResNet模型:
```python
resnet = models.resnet50(pretrained=True)
```
在上述代码中,我们使用预训练的ResNet-50模型。如果想要使用其他版本的ResNet,可以将`resnet50`替换为`resnet18`、`resnet34`、`resnet101`等。
3. 修改最后一层:
```python
num_classes = 10 # 根据具体任务设置类别数
resnet.fc = nn.Linear(resnet.fc.in_features, num_classes)
```
上述代码将ResNet模型的最后一层全连接层替换为一个具有`num_classes`个输出节点的线性层。`resnet.fc.in_features`表示原始模型最后一层的输入特征数。
4. 训练模型:
```python
# 定义损失函数和优化器
criterion = nn.CrossEntropyLoss()
optimizer = torch.optim.SGD(resnet.parameters(), lr=0.001, momentum=0.9)
# 迭代训练模型
for epoch in range(num_epochs):
# 前向传播
outputs = resnet(inputs)
loss = criterion(outputs, labels)
# 反向传播和优化
optimizer.zero_grad()
loss.backward()
optimizer.step()
```
在上述代码中,您需要根据具体的任务定义适当的损失函数和优化器。
这只是一个简单的示例,您可以根据自己的需求进行修改和扩展。希望对您有所帮助!
pytorch残差网络构建
要构建一个PyTorch残差网络,你可以按照以下步骤进行操作:
1. 首先,导入所需的PyTorch库:
```python
import torch
import torch.nn as nn
```
2. 定义一个基本的残差块(Residual Block):
```python
class ResidualBlock(nn.Module):
def __init__(self, in_channels, out_channels, stride=1):
super(ResidualBlock, self).__init__()
self.conv1 = nn.Conv2d(in_channels, out_channels, kernel_size=3, stride=stride, padding=1, bias=False)
self.bn1 = nn.BatchNorm2d(out_channels)
self.relu = nn.ReLU(inplace=True)
self.conv2 = nn.Conv2d(out_channels, out_channels, kernel_size=3, stride=1, padding=1, bias=False)
self.bn2 = nn.BatchNorm2d(out_channels)
# 如果输入和输出通道数不相同,则使用 1x1 卷积进行维度匹配
if stride != 1 or in_channels != out_channels:
self.shortcut = nn.Sequential(
nn.Conv2d(in_channels, out_channels, kernel_size=1, stride=stride, bias=False),
nn.BatchNorm2d(out_channels)
)
else:
self.shortcut = nn.Identity()
def forward(self, x):
residual = x
out = self.conv1(x)
out = self.bn1(out)
out = self.relu(out)
out = self.conv2(out)
out = self.bn2(out)
out += self.shortcut(residual)
out = self.relu(out)
return out
```
3. 构建整个残差网络(ResNet):
```python
class ResNet(nn.Module):
def __init__(self, num_classes=10):
super(ResNet, self).__init__()
self.in_channels = 64
self.conv1 = nn.Conv2d(3, 64, kernel_size=7, stride=2, padding=3, bias=False)
self.bn1 = nn.BatchNorm2d(64)
self.relu = nn.ReLU(inplace=True)
self.maxpool = nn.MaxPool2d(kernel_size=3, stride=2, padding=1)
self.layer1 = self._make_layer(64, 3, stride=1)
self.layer2 = self._make_layer(128, 4, stride=2)
self.layer3 = self._make_layer(256, 6, stride=2)
self.layer4 = self._make_layer(512, 3, stride=2)
self.avgpool = nn.AdaptiveAvgPool2d((1, 1))
self.fc = nn.Linear(512, num_classes)
def _make_layer(self, out_channels, num_blocks, stride):
layers = []
layers.append(ResidualBlock(self.in_channels, out_channels, stride))
self.in_channels = out_channels
for _ in range(1, num_blocks):
layers.append(ResidualBlock(self.in_channels, out_channels))
return nn.Sequential(*layers)
def forward(self, x):
out = self.conv1(x)
out = self.bn1(out)
out = self.relu(out)
out = self.maxpool(out)
out = self.layer1(out)
out = self.layer2(out)
out = self.layer3(out)
out = self.layer4(out)
out = self.avgpool(out)
out = out.view(out.size(0), -1)
out = self.fc(out)
return out
```
4. 使用定义好的残差网络进行训练和测试:
```python
model = ResNet(num_classes=10)
criterion = nn.CrossEntropyLoss()
optimizer = torch.optim.SGD(model.parameters(), lr=0.1, momentum=0.9, weight_decay=5e-4)
# 在训练集上进行训练...
# 在测试集上进行测试...
```
这是一个简化的ResNet实现示例,你可以根据自己的需求进行修改和扩展。希望对你有帮助!
相关推荐
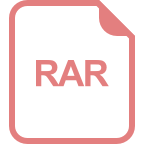
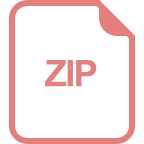












