random_state在xgboost中的取值范围是多少
时间: 2024-04-10 15:33:50 浏览: 430
在XGBoost中,`random_state`参数用于设置随机种子。随机种子控制着每次运行模型时随机性的产生,从而使结果可以重现。
`random_state`的取值可以是任意整数,或者可以为`None`。当指定一个整数时,它将作为随机种子的固定值,以确保每次运行时都获得相同的结果。如果设置为`None`,则使用系统时间作为随机种子,每次运行时都会有不同的结果。
例如,你可以将`random_state`设置为整数1来固定随机种子:
```python
import xgboost as xgb
model = xgb.XGBRegressor(random_state=1)
```
这样,每次运行模型时都会得到相同的结果。请注意,不同的参数组合和数据集可能需要不同的随机种子来获得最佳结果。
相关问题
params = { 'eval_metric': 'rmse', 'max_depth': max_depth, 'learning_rate': learning_rate, 'n_estimators': n_estimators, 'gamma': gamma, 'min_child_weight': min_child_weight, 'subsample': subsample, 'colsample_bytree':colsample_bytree, 'n_jobs': -1, 'random_state': 42 }
这段代码是在定义 XGBoost 模型的参数。其中,'colsample_bytree' 参数指定了每棵树在进行拟合时随机选择的特征占比。这个参数的取值范围是 (0, 1],表示每棵树在进行拟合时最多使用训练集中的多少特征。如果这个参数的值太小,可能会导致模型欠拟合;如果太大,可能会导致模型过拟合。
在定义参数时,你需要将 'colsample_bytree' 参数设置为一个浮点数值,例如:
```
colsample_bytree = 0.8
params = {
'eval_metric': 'rmse',
'max_depth': max_depth,
'learning_rate': learning_rate,
'n_estimators': n_estimators,
'gamma': gamma,
'min_child_weight': min_child_weight,
'subsample': subsample,
'colsample_bytree': colsample_bytree,
'n_jobs': -1,
'random_state': 42
}
```
这将使用 colsample_bytree 参数为 0.8 的 XGBoost 模型进行训练。
colsample_bytree = 0.8 gammma=0.1 params = { 'eval_metric': 'rmse', 'max_depth': max_depth, 'learning_rate': learning_rate, 'n_estimators': n_estimators, 'gamma': gamma, 'min_child_weight': min_child_weight, 'subsample': subsample, 'colsample_bytree':colsample_bytree, 'n_jobs': -1, 'random_state': 42 }
在这段代码中,你定义了 XGBoost 模型的参数。其中,'colsample_bytree' 参数指定了每棵树在进行拟合时随机选择的特征占比,取值为 0.8;'gamma' 参数指定了每个叶子节点分裂所需的最小损失减少值,取值为 0.1。
同时,你还定义了其他的参数,例如最大深度、学习率、子采样率等等,这些参数都可以影响到模型的性能。
最后,你将所有的参数都存储在一个字典中,以便在调用 XGBoost 模型时使用,例如:
```
xgb.train(params, dtrain, num_boost_round=10, evals=[(dtest, "Test")], early_stopping_rounds=3)
```
这将使用上述定义的参数来训练 XGBoost 模型。
阅读全文
相关推荐
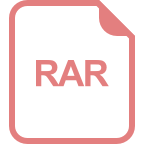
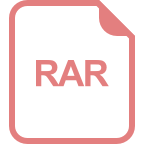
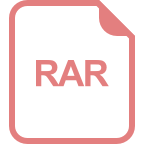
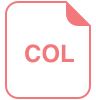
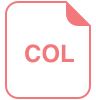
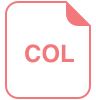
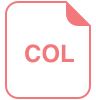
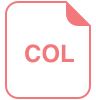
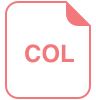
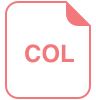
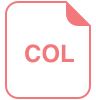





