'KMeans' object has no attribute 'preidct'
时间: 2024-06-15 21:01:14 浏览: 150
这个错误通常是因为在代码中使用了错误的方法名,正确的方法名应该是"predict"而不是"preidct"。 "KMeans"是一种聚类算法,用于将数据分成多个组(即簇),其中每个组包含具有相似特征的数据点。在使用KMeans算法进行聚类时,需要先使用fit方法将数据拟合到模型中,然后再使用predict方法对新数据进行预测,并将其分配到相应的簇中。
如果您使用的是"preidct"方法而不是"predict"方法,那么就会报出"KMeans object has no attribute 'preidct'"的错误。请检查您的代码,确保正确拼写了方法名称,并且正确地使用了KMeans算法。如果您仍然遇到问题,请提供更多的信息,以便我能够更好地帮助您。
相关问题
kmeans object has no attribute labels
The error message "kmeans object has no attribute labels" typically occurs when you try to access the "labels" attribute of a KMeans object in scikit-learn, but the attribute does not exist.
In scikit-learn, the KMeans algorithm does not have a "labels" attribute by default. Instead, you can use the "predict" method to assign labels to new data points based on a trained KMeans model. For example:
```
from sklearn.cluster import KMeans
# create KMeans object and fit it to data
kmeans = KMeans(n_clusters=3)
kmeans.fit(X)
# assign labels to new data points
labels = kmeans.predict(new_data)
```
If you need to access the cluster labels assigned to the training data by KMeans, you can use the "labels_" attribute instead:
```
# get cluster labels assigned to training data
train_labels = kmeans.labels_
```
Note that the "labels_" attribute only exists after the KMeans object has been fit to data.
KMeans object has no attribute 'labels
这个错误通常发生在使用KMeans聚类算法时,可能是因为你的代码中没有对KMeans对象进行训练或者训练不成功,导致没有生成聚类标签。你可以检查以下代码中是否有对KMeans对象进行拟合的语句,例如使用fit()函数对数据进行训练。
如果有,你可以尝试检查训练数据的格式是否正确,或者尝试调整KMeans算法的超参数以获得更好的聚类效果。
如果没有,你需要在使用KMeans对象之前对其进行拟合,例如使用fit()函数对数据进行训练,然后才能使用labels属性获取聚类标签。
阅读全文
相关推荐
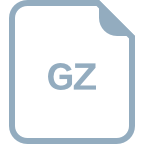















