GAT python
时间: 2023-08-25 17:17:54 浏览: 63
在Python中,你可以使用深度学习框架例如PyTorch或TensorFlow来实现GAT模型。这里给出一个使用PyTorch实现GAT的简单示例代码:
```python
import torch
import torch.nn as nn
import torch.nn.functional as F
class GraphAttentionLayer(nn.Module):
def __init__(self, in_features, out_features):
super(GraphAttentionLayer, self).__init__()
self.W = nn.Linear(in_features, out_features)
self.a = nn.Linear(2*out_features, 1)
def forward(self, x, adj):
h = self.W(x)
N = h.size(0)
a_input = torch.cat([h.repeat(1, N).view(N*N, -1), h.repeat(N, 1)], dim=1).view(N, -1, 2*h.size(1))
e = F.leaky_relu(self.a(a_input).squeeze(2))
zero_vec = -9e15*torch.ones_like(e)
attention = torch.where(adj > 0, e, zero_vec)
attention = F.softmax(attention, dim=1)
h_prime = torch.bmm(attention.unsqueeze(0), h.unsqueeze(0))
return F.elu(h_prime)
class GAT(nn.Module):
def __init__(self, in_features, hidden_features, out_features, num_heads):
super(GAT, self).__init__()
self.attentions = [GraphAttentionLayer(in_features, hidden_features) for _ in range(num_heads)]
for i, attention in enumerate(self.attentions):
self.add_module('attention_{}'.format(i), attention)
self.out_att = GraphAttentionLayer(hidden_features*num_heads, out_features)
def forward(self, x, adj):
x = F.dropout(x, p=0.6, training=self.training)
x = torch.cat([att(x, adj) for att in self.attentions], dim=2)
x = F.dropout(x, p=0.6, training=self.training)
x = F.elu(self.out_att(x, adj))
return F.log_softmax(x, dim=2)
```
这是一个简化的GAT模型实现,包括GraphAttentionLayer和GAT两个类。你可以根据自己的需求和数据的特点进行适当的修改和扩展。
这段代码中,GraphAttentionLayer类定义了一个注意力层,GAT类则定义了整个GAT模型。在forward方法中,通过多头注意力机制和注意力权重的计算,将节点特征进行聚合和更新,最后输出预测结果。
请注意,这只是一个简单的示例代码,实际应用中你可能需要根据具体场景进行调整和改进。希望对你有所帮助!如果你还有其他问题,请随时提问。
相关推荐
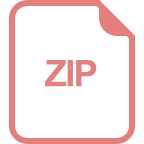
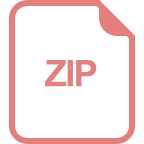









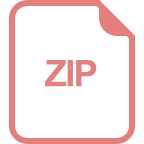
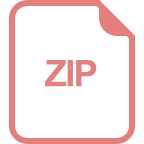
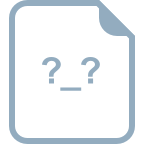
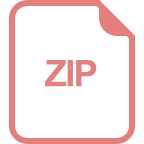