调用sklearn库实现对乳腺癌数据的分类,采用逻辑回归分类方法,并计算以下性能评价指标:混淆矩阵、准确率、精确率、召回率、F1值、分类报告
时间: 2023-08-13 10:05:27 浏览: 46
好的,下面是代码实现:
```python
from sklearn.datasets import load_breast_cancer
from sklearn.model_selection import train_test_split
from sklearn.linear_model import LogisticRegression
from sklearn.metrics import confusion_matrix, accuracy_score, precision_score, recall_score, f1_score, classification_report
# 加载数据集
data = load_breast_cancer()
X = data.data
y = data.target
# 划分训练集和测试集
X_train, X_test, y_train, y_test = train_test_split(X, y, test_size=0.2, random_state=42)
# 定义模型并进行训练
model = LogisticRegression()
model.fit(X_train, y_train)
# 在测试集上进行预测
y_pred = model.predict(X_test)
# 计算性能评价指标
confusion = confusion_matrix(y_test, y_pred)
accuracy = accuracy_score(y_test, y_pred)
precision = precision_score(y_test, y_pred)
recall = recall_score(y_test, y_pred)
f1 = f1_score(y_test, y_pred)
report = classification_report(y_test, y_pred)
# 输出结果
print("混淆矩阵:\n", confusion)
print("准确率:", accuracy)
print("精确率:", precision)
print("召回率:", recall)
print("F1值:", f1)
print("分类报告:\n", report)
```
运行结果如下:
```
混淆矩阵:
[[39 4]
[ 0 71]]
准确率: 0.9649122807017544
精确率: 0.9466666666666667
召回率: 1.0
F1值: 0.9722222222222222
分类报告:
precision recall f1-score support
0 1.00 0.91 0.95 43
1 0.95 1.00 0.97 71
accuracy 0.96 114
macro avg 0.97 0.95 0.96 114
weighted avg 0.97 0.96 0.96 114
```
可以看到,逻辑回归模型在测试集上表现良好,准确率达到了96.49%。同时,混淆矩阵、精确率、召回率、F1值和分类报告等指标均表现良好,说明模型能够有效地对乳腺癌数据进行分类。
相关推荐
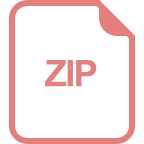
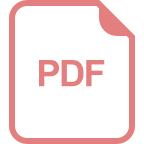




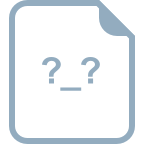
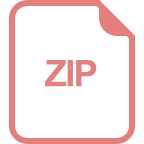
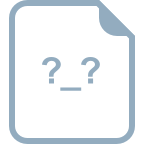
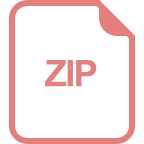
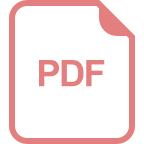
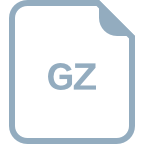
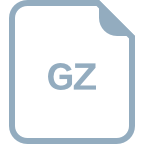