生成一段cifar_net.pth图像识别模型的代码
时间: 2024-02-11 20:09:26 浏览: 171
好的,下面是生成一个基于CIFAR-10数据集的图像识别模型并保存为cifar_net.pth文件的示例代码:
```python
import torch
import torch.nn as nn
import torch.optim as optim
import torchvision.transforms as transforms
import torchvision.datasets as datasets
# Define the neural network architecture
class Net(nn.Module):
def __init__(self):
super(Net, self).__init__()
self.conv1 = nn.Conv2d(3, 6, 5)
self.pool = nn.MaxPool2d(2, 2)
self.conv2 = nn.Conv2d(6, 16, 5)
self.fc1 = nn.Linear(16 * 5 * 5, 120)
self.fc2 = nn.Linear(120, 84)
self.fc3 = nn.Linear(84, 10)
def forward(self, x):
x = self.pool(nn.functional.relu(self.conv1(x)))
x = self.pool(nn.functional.relu(self.conv2(x)))
x = x.view(-1, 16 * 5 * 5)
x = nn.functional.relu(self.fc1(x))
x = nn.functional.relu(self.fc2(x))
x = self.fc3(x)
return x
# Load the CIFAR-10 dataset
transform = transforms.Compose(
[transforms.ToTensor(),
transforms.Normalize((0.5, 0.5, 0.5), (0.5, 0.5, 0.5))]
)
trainset = datasets.CIFAR10(root='./data', train=True,
download=True, transform=transform)
trainloader = torch.utils.data.DataLoader(trainset, batch_size=4,
shuffle=True, num_workers=2)
testset = datasets.CIFAR10(root='./data', train=False,
download=True, transform=transform)
testloader = torch.utils.data.DataLoader(testset, batch_size=4,
shuffle=False, num_workers=2)
# Initialize the neural network and the optimizer
net = Net()
criterion = nn.CrossEntropyLoss()
optimizer = optim.SGD(net.parameters(), lr=0.001, momentum=0.9)
# Train the neural network
for epoch in range(2):
running_loss = 0.0
for i, data in enumerate(trainloader, 0):
inputs, labels = data
optimizer.zero_grad()
outputs = net(inputs)
loss = criterion(outputs, labels)
loss.backward()
optimizer.step()
running_loss += loss.item()
if i % 2000 == 1999:
print('[%d, %5d] loss: %.3f' %
(epoch + 1, i + 1, running_loss / 2000))
running_loss = 0.0
print('Finished Training')
# Test the neural network
correct = 0
total = 0
with torch.no_grad():
for data in testloader:
images, labels = data
outputs = net(images)
_, predicted = torch.max(outputs.data, 1)
total += labels.size(0)
correct += (predicted == labels).sum().item()
print('Accuracy of the network on the 10000 test images: %d %%' % (
100 * correct / total))
# Save the trained model weights to a .pth file
PATH = './cifar_net.pth'
torch.save(net.state_dict(), PATH)
```
这段代码定义了一个包含两个卷积层和三个全连接层的神经网络,使用CIFAR-10数据集进行训练,并在测试集上进行了准确率测试。最后,将训练好的神经网络权重保存到cifar_net.pth文件中。
阅读全文
相关推荐
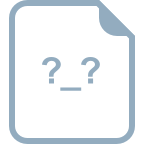
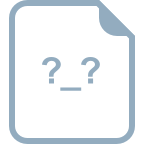

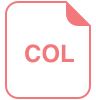
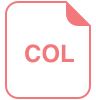
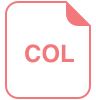
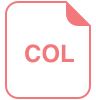
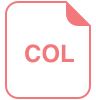
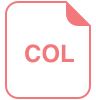
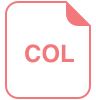
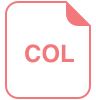
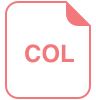
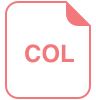
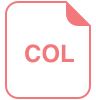
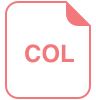
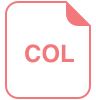
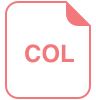