torch.max(2)[0]
时间: 2024-01-10 14:01:52 浏览: 67
这条命令不完整,缺少输入张量参数。torch.max(input, dim=None, keepdim=False, out=None) 函数返回输入张量中指定维度上的最大值。其中,dim参数指定计算最大值的维度,keepdim参数指定是否保留维度大小为1的维度,out参数指定输出张量。如果你想要计算一个张量在第二个维度上的最大值并返回该值,则可以使用以下命令:
```
import torch
x = torch.randn(3, 4)
max_value = torch.max(x, dim=1)[0]
print(max_value)
```
输出为:
```
tensor([0.5373, 0.2681, 0.7473])
```
其中,x是一个3行4列的张量,torch.max(x, dim=1)返回一个元组,包含该张量在第二个维度上的最大值和最大值的索引。由于我们只需要最大值,因此使用[0]获取该元组的第一个元素即可。
相关问题
torch.nn.maxpool2d 和torch.nn.maxpool1d有什么区别
`torch.nn.maxpool2d` 和 `torch.nn.maxpool1d` 是 PyTorch 中用于实现最大池化操作的两个函数,它们的区别在于输入数据的维度不同。
`torch.nn.maxpool2d` 是用于二维输入数据(例如图像)的最大池化操作,它会将输入数据沿着宽度和高度方向进行池化,输出一个降低了尺寸的二维特征图。
`torch.nn.maxpool1d` 是用于一维输入数据(例如时间序列)的最大池化操作,它会将输入数据沿着一个维度(通常是时间维度)进行池化,输出一个降低了尺寸的一维特征图。
因此,这两个函数虽然都是用于最大池化操作,但是针对的输入数据不同,所以需要分别使用。
def forward(self, x1, x2): x1 = x1.to(torch.float32) x2 = x2.to(torch.float32) channel1_conv1 = self.channel1_conv1(x1).squeeze(dim=2) channel1_conv1 = torch.max(channel1_conv1, dim=1)[0].unsqueeze(dim=1) channel1_conv2 = self.channel1_conv2(x1).squeeze(dim=2) channel1_conv2 = torch.max(channel1_conv2, dim=1)[0].unsqueeze(dim=1) channel1_conv3 = self.channel1_conv3(x1).squeeze(dim=2) channel1_conv3 = torch.max(channel1_conv3, dim=1)[0].unsqueeze(dim=1) channel1_conv4 = self.channel1_conv4(x1).squeeze(dim=2) channel1_conv4 = torch.max(channel1_conv4, dim=1)[0].unsqueeze(dim=1) X1 = torch.cat([channel1_conv1, channel1_conv2, channel1_conv3, channel1_conv4], dim=1) channel2_conv1 = self.channel2_conv1(x2).squeeze(dim=2) channel2_conv1 = torch.max(channel2_conv1, dim=1)[0].unsqueeze(dim=1) channel2_conv2 = self.channel2_conv2(x2).squeeze(dim=2) channel2_conv2 = torch.max(channel2_conv2, dim=1)[0].unsqueeze(dim=1) channel2_conv3 = self.channel2_conv3(x2).squeeze(dim=2) channel2_conv3 = torch.max(channel2_conv3, dim=1)[0].unsqueeze(dim=1) channel2_conv4 = self.channel2_conv4(x2).squeeze(dim=2) channel2_conv4 = torch.max(channel2_conv4, dim=1)[0].unsqueeze(dim=1) X2 = torch.cat([channel2_conv1, channel2_conv2, channel2_conv3, channel2_conv4], dim=1) X2 = torch.cat([X1, X2], dim=1) output = self.layer3(X2)
这段代码是一个双通道的卷积神经网络的前向传播函数,输入是两个张量 x1 和 x2,经过一些卷积、池化和拼接操作后,最终输出一个张量 output。这个神经网络的具体结构是由两个通道的卷积层、池化层和全连接层构成的,其中 channel1_conv1-4 和 channel2_conv1-4 是卷积层,layer3 是全连接层。在前向传播过程中,先对两个通道的数据进行卷积和池化操作,然后将两个通道的结果拼接在一起,最后通过全连接层生成输出。需要注意的是,这个神经网络对输入数据进行了类型转换,将输入张量的数据类型转换为 float32。
阅读全文
相关推荐
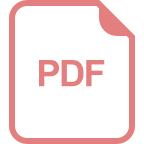
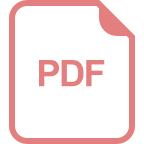
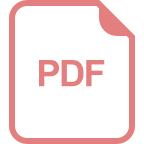













