net = nn.Sequential(nn.Linear(in_features,1))
时间: 2023-07-22 12:56:07 浏览: 122
这行代码定义了一个简单的线性回归模型,该模型由一个全连接层(fully connected layer)组成,输入特征的维度为 `in_features`,输出特征的维度为 1,即模型输出一个标量。具体来说,`nn.Sequential` 表示将多个神经网络层按顺序连接起来形成一个神经网络模型。在这里,我们只有一个全连接层,即 `nn.Linear(in_features, 1)`,它表示将输入特征的维度映射到输出特征的维度,同时进行线性变换。因此,这个模型实现的是一个简单的线性回归模型,用于预测一个连续的目标值。
线性回归模型是一种常用的回归模型,它通过线性变换将输入特征映射到输出特征,用于预测一个连续的目标值。线性回归模型的参数可以通过最小化训练数据的均方误差(mean squared error,MSE)来进行优化,常用的优化算法包括随机梯度下降(stochastic gradient descent,SGD)和其它的优化算法(如 Adam、Adagrad、RMSProp 等)。
相关问题
self.linear_relu_stack = nn.Sequential( nn.Linear(in_features=28 * 28, out_features=512), nn.ReLU(), nn.Linear(in_features=512, out_features=512), nn.ReLU(), nn.Linear(in_features=512, out_features=10), )
这是一个典型的神经网络模型,包含了三个线性层和两个激活函数。首先,输入层是一个具有28 * 28个特征的向量,经过第一个线性层,将输入特征映射到一个具有512个神经元的隐藏层,并通过ReLU激活函数进行非线性变换。然后,第二个线性层将隐藏层的输出映射到另一个512个神经元的隐藏层,并再次通过ReLU激活函数进行非线性变换。最后,第三个线性层将最后一个隐藏层的输出映射到一个具有10个神经元的输出层,表示模型的预测结果。
self.fc1 = nn.Sequential( nn.Linear(in_features=12*7*7, out_features=196), nn.ReLU(), ) # 补充第二个全连接层... self.fc2 = nn.Sequential( nn.Linear(in_features=196, out_features=84), nn.ReLU(), ) # 补充第三个全连接层... self.fc2 = nn.Sequential( nn.Linear(in_features=84, out_features=n_classes), nn.ReLU(), )有什么错误吗
这段代码有一个错误,就是最后一个全连接层的定义重复了,应该将其改为`self.fc3`,而不是`self.fc2`。正确的代码如下:
```
self.fc1 = nn.Sequential(
nn.Linear(in_features=12*7*7, out_features=196),
nn.ReLU(),
)
# 补充第二个全连接层...
self.fc2 = nn.Sequential(
nn.Linear(in_features=196, out_features=84),
nn.ReLU(),
)
# 补充第三个全连接层...
self.fc3 = nn.Sequential(
nn.Linear(in_features=84, out_features=n_classes),
nn.ReLU(),
)
```
其中,`n_classes`表示分类的类别数。在最后一个全连接层中,一般不使用激活函数,因为输出结果需要经过softmax进行归一化处理。因此,可以将nn.ReLU()改为nn.Identity(),即不使用任何激活函数。
阅读全文
相关推荐
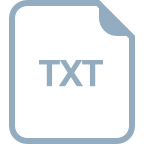
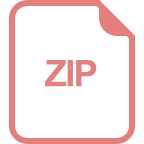














