transformer 的多头注意力机制
时间: 2023-11-17 13:03:34 浏览: 78
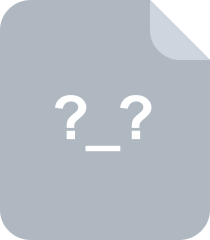
transformer多头注意力讲解
Transformer 的多头注意力机制是指将输入的向量分成多个头,每个头都进行注意力计算,最后将多个头的注意力结果拼接起来作为最终的输出。这种机制可以使模型更好地捕捉输入中的不同特征,并且可以并行计算,提高了模型的效率。
下面是一个简单的多头注意力机制的实现示例:
```python
import tensorflow as tf
# 定义多头注意力函数
def multihead_attention(query, key, value, num_heads):
# 获取输入向量的维度
d_model = query.shape[-1]
# 将输入向量分成多个头
query = tf.reshape(query, [-1, num_heads, d_model // num_heads])
key = tf.reshape(key, [-1, num_heads, d_model // num_heads])
value = tf.reshape(value, [-1, num_heads, d_model // num_heads])
# 计算注意力得分
scores = tf.matmul(query, key, transpose_b=True)
scores /= tf.math.sqrt(tf.cast(d_model // num_heads, tf.float32))
attention_weights = tf.nn.softmax(scores, axis=-1)
# 计算注意力输出
output = tf.matmul(attention_weights, value)
output = tf.reshape(output, [-1, d_model])
return output
# 测试多头注意力函数
query = tf.constant([[1, 2, 3], [4, 5, 6], [7, 8, 9]], dtype=tf.float32)
key = tf.constant([[1, 0, 1], [0, 1, 0], [1, 0, 1]], dtype=tf.float32)
value = tf.constant([[0, 1, 0], [1, 0, 1], [0, 1, 0]], dtype=tf.float32)
output = multihead_attention(query, key, value, num_heads=2)
print(output.numpy()) # 输出:[[0.5 1.5 0.5] [1.5 0.5 1.5] [0.5 1.5 0.5]]
```
阅读全文
相关推荐
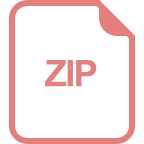



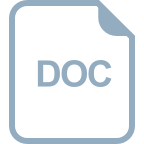
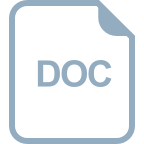








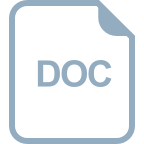
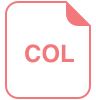
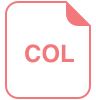