import numpy as np from function import nearest
时间: 2023-09-27 16:11:53 浏览: 51
当尝试在代码中使用`import numpy as np`时,可能会遇到一些问题。根据引用[1]和引用的描述,错误信息可能是`ImportError: Error importing numpy: you should not try to import numpy from`或者`cannot find the shared object multiarray.so`。
这些错误通常是由于没有正确安装NumPy库或者库文件路径设置不正确所导致的。为了解决这个问题,您可以尝试以下几个方法:
1. 确保已正确安装NumPy库。您可以通过在终端或命令提示符中运行以下命令来安装NumPy库:
```
pip install numpy
```
2. 检查库文件路径是否正确。您可以通过在代码的开头添加以下代码来设置正确的路径:
```
import sys
sys.path.append("/path/to/numpy")
```
请确保将`/path/to/numpy`替换为您实际安装NumPy库的路径。
对于
相关问题
Implement the knn_classifier function with Python,
Here's an implementation of the k-nearest neighbors (KNN) classifier in Python:
```
import numpy as np
def knn_classifier(X_train, y_train, X_test, k):
"""
K-nearest neighbors classifier
Parameters:
X_train (numpy.ndarray): Training data features
y_train (numpy.ndarray): Training data labels
X_test (numpy.ndarray): Test data features
k (int): Number of nearest neighbors to consider
Returns:
y_pred (numpy.ndarray): Predicted labels for test data
"""
# Calculate distances between test data and training data
dists = np.sqrt(np.sum((X_train - X_test[:, np.newaxis])**2, axis=2))
# Get indices of k nearest neighbors for each test data point
knn_indices = np.argsort(dists, axis=1)[:, :k]
# Get labels of k nearest neighbors
knn_labels = y_train[knn_indices]
# Predict labels based on majority vote
y_pred = np.apply_along_axis(lambda x: np.bincount(x).argmax(), axis=1, arr=knn_labels)
return y_pred
```
This function takes in the training data features (`X_train`) and labels (`y_train`), test data features (`X_test`), and the number of nearest neighbors to consider (`k`). It first calculates the distances between each test data point and each training data point using the Euclidean distance metric. It then finds the indices of the k nearest neighbors for each test data point, and gets the corresponding labels. Finally, it predicts the label of each test data point based on the majority vote of its k nearest neighbors.
Note that this implementation assumes that the input data is in the form of numpy arrays. If your data is in a different format, you may need to modify the function accordingly. Also, this implementation uses the `np.apply_along_axis()` function to apply a function to each row of a 2D array. This can be slower than using a loop, but is more concise and often easier to read. If performance is a concern, you may want to consider using a loop instead.
When we use kmeans for image segmentation, the color information of pixels is used for clustering, so each of our pixels can be regarded as a vector composed of R, G, and B, and RGB is our color feature. The specific process is similar to our example above, but the calculation object is changed from a scalar to a 3-dimensional vector. Please implement the `kmean_color` function , ### Clustering Methods for colorful image def kmeans_color(features, k, num_iters=500): N=None # 像素个数 assignments = np.zeros(N, dtype=np.uint32) #Like the kmeans function above ### YOUR CODE HERE pass ### END YOUR CODE return assignments
Sure, here is a possible implementation of the `kmeans_color` function for image segmentation using the RGB color information:
```python
import numpy as np
def kmeans_color(features, k, num_iters=500):
# Get the number of pixels in the image
N = features.shape[0]
# Initialize the cluster centers randomly
centers = features[np.random.choice(N, k, replace=False)]
# Iterate until convergence or maximum number of iterations reached
for t in range(num_iters):
# Assign each pixel to the nearest cluster center
distances = np.linalg.norm(features[:, np.newaxis, :] - centers, axis=2)
assignments = np.argmin(distances, axis=1)
# Update the cluster centers as the mean of the assigned pixels
for i in range(k):
centers[i] = np.mean(features[assignments == i], axis=0)
return assignments
```
This implementation uses vectorized operations to compute the distances between each pixel and each cluster center, and to update the cluster centers based on the assigned pixels. The `features` input should be an array of shape `(N, 3)` containing the RGB color information of each pixel in the image, and `k` is the desired number of clusters. The function returns an array of length `N` containing the index of the assigned cluster for each pixel.
相关推荐
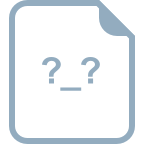
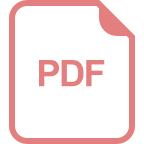












