用tensorflow写一个程序:强化学习
时间: 2023-06-30 14:03:09 浏览: 182
好的,以下是一个简单的强化学习程序,使用了 TensorFlow 和 OpenAI Gym 模块:
```python
import tensorflow as tf
import numpy as np
import gym
# 创建 CartPole 游戏环境
env = gym.make('CartPole-v0')
# 定义神经网络模型
model = tf.keras.models.Sequential([
tf.keras.layers.Dense(24, activation='relu', input_shape=(4,)),
tf.keras.layers.Dense(24, activation='relu'),
tf.keras.layers.Dense(2, activation='linear')
])
# 定义优化器和损失函数
optimizer = tf.keras.optimizers.Adam()
loss_fn = tf.keras.losses.MeanSquaredError()
# 定义超参数
gamma = 0.99 # 折扣因子
epsilon = 1.0 # ε-贪心策略中的初始 ε 值
epsilon_min = 0.01 # ε-贪心策略中的最小 ε 值
epsilon_decay = 0.995 # ε-贪心策略中的衰减值
batch_size = 32 # 每个批次的样本数量
memory = [] # 记忆池
# 定义动作选择函数
def choose_action(state):
if np.random.rand() < epsilon:
return env.action_space.sample()
else:
Q_values = model.predict(state[np.newaxis])
return np.argmax(Q_values[0])
# 定义经验回放函数
def replay(batch_size):
batch = np.random.choice(len(memory), batch_size, replace=False)
for index in batch:
state, action, reward, next_state, done = memory[index]
target = model.predict(state[np.newaxis])
if done:
target[0][action] = reward
else:
Q_future = np.max(model.predict(next_state[np.newaxis])[0])
target[0][action] = reward + Q_future * gamma
model.fit(state[np.newaxis], target, epochs=1, verbose=0)
# 训练模型
for episode in range(1000):
state = env.reset()
done = False
total_reward = 0
while not done:
action = choose_action(state)
next_state, reward, done, _ = env.step(action)
memory.append((state, action, reward, next_state, done))
state = next_state
total_reward += reward
if len(memory) > batch_size:
replay(batch_size)
epsilon = max(epsilon_min, epsilon * epsilon_decay)
print("Episode {}: Score = {}, ε = {:.2f}".format(episode, total_reward, epsilon))
```
这个程序使用了深度 Q 学习算法,训练一个神经网络模型来学习在 CartPole 游戏中如何选择动作。它通过与环境交互来收集数据,然后使用经验回放方法来训练模型。在训练过程中,ε-贪心策略用于平衡探索和利用之间的权衡。最终,模型可以在游戏中取得高分数。
阅读全文
相关推荐






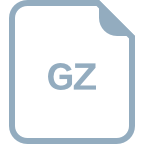








