loss_recon = nn.MSELoss()(real_images[:, :, :, 1:128], fake_images[:, :, :, 1:128])
时间: 2024-05-21 14:12:38 浏览: 16
我的回答是,loss_recon 是一个用来衡量真实图片和生成图片之间的均方误差的损失函数。其中 real_images[:, :, :, 1:128] 和 fake_images[:, :, :, 1:128] 是对应真实图片和生成图片的通道数为 1~128 的部分。这个损失函数可以用来优化生成器的训练,使得生成的图片更加接近真实图片。
相关问题
def forward(self, x): z = self._encoder(x) z = self._pre_vq_conv(z) loss, quantized, perplexity, _ = self._vq_vae(z) x_recon = self._decoder(quantized)
这段代码是一个神经网络模型的前向传递函数,用于输入数据 `x`,并输出模型的预测结果。
首先,数据 `x` 通过模型的 `_encoder` 层进行编码,然后通过 `_pre_vq_conv` 层进行卷积操作。
接下来,经过 `_vq_vae` 层进行向量量化(vector quantization)和自编码器(autoencoder)操作,并计算损失、量化后的结果、困惑度(perplexity)等参数。
最后,量化后的结果 `quantized` 通过 `_decoder` 层进行解码,得到重建后的结果 `x_recon`。
总的来说,这段代码实现了一个基于向量量化和自编码器的神经网络模型,用于对输入数据进行编码、解码和重建。
def train(images, labels, epoch, training_mode): with tf.GradientTape() as tape: if training_mode == 'full': predictions = bc_model(images) elif training_mode == 'latent': z, _, _ = cmvae_model.encode(images) predictions = bc_model(z) elif training_mode == 'reg': z = reg_model(images) predictions = bc_model(z) recon_loss = tf.reduce_mean(compute_loss(labels, predictions)) gradients = tape.gradient(recon_loss, bc_model.trainable_variables) optimizer.apply_gradients(zip(gradients, bc_model.trainable_variables)) train_loss_rec_v(recon_loss)
这段代码实现了一个模型的训练过程,训练的是一个基于条件变分自编码器(CMVAE)的卷积神经网络(CNN),可以在完全训练模式、潜变量训练模式和正则化训练模式下进行。这里的`bc_model`是一个卷积神经网络模型,`cmvae_model`是一个条件变分自编码器模型,`reg_model`是一个正则化模型。`compute_loss`是一个计算损失函数的函数。
在训练过程中,首先根据训练模式(`training_mode`)选择不同的模型进行预测(`predictions = bc_model(images)`),然后计算预测结果与标签数据的损失(`recon_loss = tf.reduce_mean(compute_loss(labels, predictions))`)。接着,使用`tf.GradientTape`记录损失函数对模型参数的梯度,然后使用优化器(`optimizer`)对模型参数进行更新,使得模型能够更好地拟合数据。最后,将损失函数值记录在一个变量中(`train_loss_rec_v(recon_loss)`),以便进行可视化和监控训练过程中的损失变化。
相关推荐
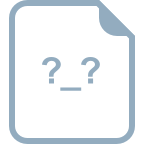












