执行代码train_source_dataset = torch.stack([train_source_dataset[i][0].repeat(3, 1, 1) for i in range(len(train_source_dataset))]),后进行data_loader,会导致data_loader返回的值与不执行该代码有何区别
时间: 2023-06-15 15:07:16 浏览: 138
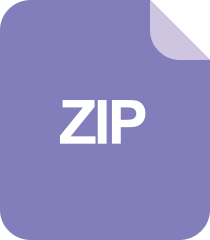
Python库 | nowcasting_dataset-2.0.39-py3-none-any.whl
执行 `train_source_dataset = torch.stack([train_source_dataset[i][0].repeat(3, 1, 1) for i in range(len(train_source_dataset))])` 会将 `train_source_dataset` 中的每一张单通道图片在通道维度上复制成三个通道,变成了三通道图片。这样做的目的是为了将单通道的图像数据转换为3通道的图像数据,使其适配某些需要输入3通道图像的模型,例如VGG等。
如果不执行这个语句,那么 `train_source_dataset` 会保持原来的单通道数据, `data_loader` 返回的tensor也会是单通道的。如果模型需要输入三通道的数据,那么就需要在 `data_loader` 中对单通道图像进行转换,使其适配模型。
阅读全文
相关推荐
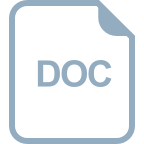
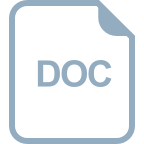















