帮我编写一个测试推理的python脚本
时间: 2024-10-23 08:05:32 浏览: 21
当然可以,以下是一个简单的Python脚本,用于测试基础的逻辑推理。这个例子会定义几个函数,然后通过调用它们来进行一些简单的推理判断:
```python
def is_even(number):
"""
判断一个数是否为偶数
"""
return number % 2 == 0
def is_prime(n):
"""
判断一个数是否为质数
"""
if n < 2:
return False
for i in range(2, int(n**0.5) + 1):
if n % i == 0:
return False
return True
def test_inference():
"""
测试推理功能
"""
assert is_even(4), "4应该是个偶数"
assert not is_even(5), "5不是偶数"
# 推理一个数字是否既是偶数又是质数
assert not (is_even(7) and is_prime(7)), "7既非偶数也非质数"
print("所有测试通过")
if __name__ == "__main__":
test_inference()
相关问题
帮我编写一个测试GPU 推理的python脚本
在Python中,我们可以使用PyTorch或TensorFlow等深度学习库来进行GPU推理的测试。这里是一个简单的例子,我们将演示如何使用PyTorch:
```python
# 首先确保已经安装了torch和cuda相关的库
import torch
from torch import nn, cuda
# 检查是否支持CUDA
if not torch.cuda.is_available():
print("当前系统不支持CUDA")
else:
print("已检测到可用的CUDA设备")
# 定义一个简单的模型,假设这是一个卷积神经网络
class SimpleModel(nn.Module):
def __init__(self):
super(SimpleModel, self).__init__()
self.conv = nn.Conv2d(1, 1, kernel_size=3)
def forward(self, x):
return self.conv(x)
# 创建模型并移至GPU
model = SimpleModel().to('cuda')
# 准备一个随机数据张量(假定输入是4D Tensor)
x = torch.randn((1, 1, 8, 8)).to('cuda')
# 执行前向传播
output = model(x)
print("Output on GPU:", output.shape)
# 计算时间(为了演示性能差异)
start_time = torch.cuda.Event(enable_timing=True)
end_time = torch.cuda.Event(enable_timing=True)
start_time.record()
output = model(x)
end_time.record()
# 等待事件计时结束
torch.cuda.synchronize()
time_taken = start_time.elapsed_time(end_time) / 1e3
print(f"GPU推理时间 (ms): {time_taken:.6f}")
帮我编写一个测试CPU和GPU推理对比的python脚本
在Python中,我们可以使用一些库如`tensorflow`, `pytorch`, 或者`cupy`来进行CPU和GPU性能的比较。这里是一个基本的示例,我们将使用TensorFlow来展示如何在CPU和GPU上运行简单的矩阵乘法:
```python
import tensorflow as tf
import time
# 创建一个简单的矩阵
matrix = tf.random.uniform((5000, 5000), dtype=tf.float32)
def run_on_cpu():
with tf.device('/cpu:0'):
start_time = time.time()
cpu_result = tf.matmul(matrix, matrix)
print(f"CPU Time: {time.time() - start_time} seconds")
def run_on_gpu():
if tf.config.list_physical_devices('GPU'):
with tf.device('/gpu:0'):
start_time = time.time()
gpu_result = tf.matmul(matrix, matrix)
print(f"GPU Time: {time.time() - start_time} seconds")
# 如果有GPU,对比两者的速度
print("Speedup (GPU/CPU):", (start_time - run_on_cpu().timestamp) / (time.time() - start_time))
run_on_cpu()
run_on_gpu()
#
阅读全文
相关推荐
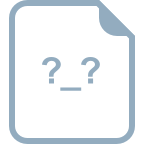
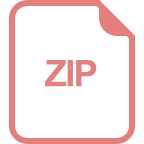
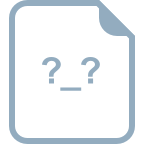



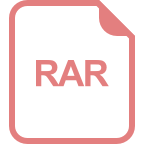
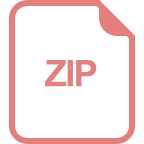
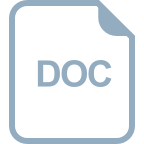
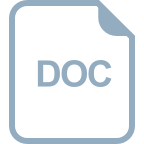
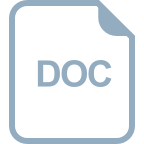
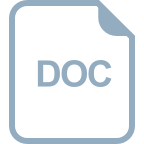
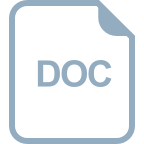
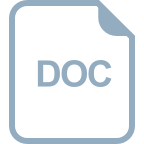
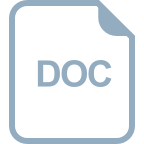

