def gradient(self, x, t): # forward self.loss(x, t) # backward dout = 1 dout = self.lastLayer.backward(dout) layers = list(self.layers.values()) layers.reverse() for layer in layers: dout = layer.backward(dout) # 設定 grads = {} grads['W1'], grads['b1'] = self.layers['Affine1'].dW, self.layers['Affine1'].db grads['W2'], grads['b2'] = self.layers['Affine2'].dW, self.layers['Affine2'].db return grads
时间: 2024-04-11 14:31:39 浏览: 35
这段代码是一个神经网络模型的梯度计算函数。在这个函数中,首先通过调用self.loss(x, t)来计算损失函数的值。然后通过调用self.lastLayer.backward(dout)来计算输出层的反向传播梯度,并将其赋值给dout。接着,通过遍历网络中的每一层,调用layer.backward(dout)来计算每一层的反向传播梯度,并将其赋值给dout。最后,将每一层的参数梯度保存在grads字典中,并返回grads。
这段代码的作用是计算神经网络模型在给定输入x和目标输出t下的参数梯度。参数梯度可以用于更新模型的参数,以使模型能更好地拟合训练数据。
相关问题
def gradient(self, x, t): # forward self.loss(x, t) # backward dout = 1 dout = self.last_layer.backward(dout) tmp_layers = self.layers.copy() tmp_layers.reverse() for layer in tmp_layers: dout = layer.backward(dout) # 设定 grads = {} for i, layer_idx in enumerate((0, 2, 5, 7, 10, 12, 15, 18)): grads['W' + str(i+1)] = self.layers[layer_idx].dW grads['b' + str(i+1)] = self.layers[layer_idx].db return grads
这段代码是一个神经网络模型的梯度计算函数,用于计算模型在给定数据 `x` 和标签 `t` 上的权重和偏置的梯度。函数的输出是一个字典 `grads`,包含了每个层的权重和偏置的梯度。
具体来说,该函数的实现过程如下:
1. 调用模型的 `loss()` 方法,计算给定数据 `x` 和标签 `t` 上的损失函数值。
2. 将反向传播的初始梯度 `dout` 初始化为 1。
3. 调用最后一层的 `backward()` 方法,计算输出层的梯度,并将计算结果存储在变量 `dout` 中。
4. 将所有层的列表 `self.layers` 中的层复制一份,并将其顺序颠倒,得到一个新的列表 `tmp_layers`。
5. 遍历列表 `tmp_layers` 中的每个层,并依次调用它们的 `backward()` 方法,计算每个层的梯度,并将计算结果存储在变量 `dout` 中。
6. 针对每个需要计算梯度的层,从 `self.layers` 中取出对应的层,并将该层的权重和偏置的梯度存储到字典 `grads` 中。
7. 返回字典 `grads`,其中包含了每个层的权重和偏置的梯度。
总的来说,这段代码的作用是计算神经网络模型的梯度,其中包括前向传播、反向传播、梯度计算和结果存储等多个步骤。梯度计算是深度学习中重要的一步,它用于更新模型的参数,提高模型的性能。
import numpy as np def sigmoid(x): # the sigmoid function return 1/(1+np.exp(-x)) class LogisticReg(object): def __init__(self, indim=1): # initialize the parameters with all zeros # w: shape of [d+1, 1] self.w = np.zeros((indim + 1, 1)) def set_param(self, weights, bias): # helper function to set the parameters # NOTE: you need to implement this to pass the autograde. # weights: vector of shape [d, ] # bias: scaler def get_param(self): # helper function to return the parameters # NOTE: you need to implement this to pass the autograde. # returns: # weights: vector of shape [d, ] # bias: scaler def compute_loss(self, X, t): # compute the loss # X: feature matrix of shape [N, d] # t: input label of shape [N, ] # NOTE: return the average of the log-likelihood, NOT the sum. # extend the input matrix # compute the loss and return the loss X_ext = np.concatenate((X, np.ones((X.shape[0], 1))), axis=1) # compute the log-likelihood def compute_grad(self, X, t): # X: feature matrix of shape [N, d] # grad: shape of [d, 1] # NOTE: return the average gradient, NOT the sum. def update(self, grad, lr=0.001): # update the weights # by the gradient descent rule def fit(self, X, t, lr=0.001, max_iters=1000, eps=1e-7): # implement the .fit() using the gradient descent method. # args: # X: input feature matrix of shape [N, d] # t: input label of shape [N, ] # lr: learning rate # max_iters: maximum number of iterations # eps: tolerance of the loss difference # TO NOTE: # extend the input features before fitting to it. # return the weight matrix of shape [indim+1, 1] def predict_prob(self, X): # implement the .predict_prob() using the parameters learned by .fit() # X: input feature matrix of shape [N, d] # NOTE: make sure you extend the feature matrix first, # the same way as what you did in .fit() method. # returns the prediction (likelihood) of shape [N, ] def predict(self, X, threshold=0.5): # implement the .predict() using the .predict_prob() method # X: input feature matrix of shape [N, d] # returns the prediction of shape [N, ], where each element is -1 or 1. # if the probability p>threshold, we determine t=1, otherwise t=-1
以下是您提供的代码的完整实现,包括_param()和get_param()方法的实现:
```python
import numpy as np
def sigmoid(x):
# the sigmoid function
return 1 / (1 + np.exp(-x))
class LogisticReg(object):
def __init__(self, indim=1):
# initialize the parameters with all zeros
# w: shape of [d+1, 1]
self.w = np.zeros((indim + 1, 1))
def set_param(self, weights, bias):
# helper function to set the parameters
# weights: vector of shape [d, ]
# bias: scalar
self.w[:-1] = weights.reshape(-1, 1)
self.w[-1] = bias
def get_param(self):
# helper function to return the parameters
# returns:
# weights: vector of shape [d, ]
# bias: scalar
weights = self.w[:-1].reshape(-1)
bias = self.w[-1]
return weights, bias
def compute_loss(self, X, t):
# compute the loss
# X: feature matrix of shape [N, d]
# t: input label of shape [N, ]
# NOTE: return the average of the log-likelihood, NOT the sum.
# extend the input matrix
X_ext = np.concatenate((X, np.ones((X.shape[0], 1))), axis=1)
# compute the log-likelihood
z = X_ext @ self.w
y = sigmoid(z)
loss = -np.mean(t * np.log(y) + (1 - t) * np.log(1 - y))
return loss
def compute_grad(self, X, t):
# X: feature matrix of shape [N, d]
# grad: shape of [d, 1]
# NOTE: return the average gradient, NOT the sum.
X_ext = np.concatenate((X, np.ones((X.shape[0], 1))), axis=1)
z = X_ext @ self.w
y = sigmoid(z)
grad = np.mean(X_ext.T @ (y - t), axis=1)
return grad
def update(self, grad, lr=0.001):
# update the weights
# by the gradient descent rule
self.w -= lr * grad.reshape(-1, 1)
def fit(self, X, t, lr=0.001, max_iters=1000, eps=1e-7):
# implement the .fit() using the gradient descent method.
# args:
# X: input feature matrix of shape [N, d]
# t: input label of shape [N, ]
# lr: learning rate
# max_iters: maximum number of iterations
# eps: tolerance of the loss difference
# TO NOTE:
# extend the input features before fitting to it.
# return the weight matrix of shape [indim+1, 1]
X_ext = np.concatenate((X, np.ones((X.shape[0], 1))), axis=1)
prev_loss = np.inf
for i in range(max_iters):
loss = self.compute_loss(X, t)
if np.abs(loss - prev_loss) < eps:
break
grad = self.compute_grad(X, t)
self.update(grad, lr)
prev_loss = loss
return self.w
def predict_prob(self, X):
# implement the .predict_prob() using the parameters learned by .fit()
# X: input feature matrix of shape [N, d]
# NOTE: make sure you extend the feature matrix first,
# the same way as what you did in .fit() method.
# returns the prediction (likelihood) of shape [N, ]
X_ext = np.concatenate((X, np.ones((X.shape[0], 1))), axis=1)
z = X_ext @ self.w
y = sigmoid(z)
return y
def predict(self, X, threshold=0.5):
# implement the .predict() using the .predict_prob() method
# X: input feature matrix of shape [N, d]
# returns the prediction of shape [N, ], where each element is -1 or 1.
# if the probability p > threshold, we determine t = 1, otherwise t = -1
prob = self.predict_prob(X)
predictions = np.where(prob > threshold, 1, -1)
return predictions
```
这个类实现了逻辑回归的基本功能,包括参数设置、计算损失、计算梯度、更新权重、拟合数据、预测概率和预测类别等方法。您可以使用这个类来拟合二分类问题的数据,并进行预测。
相关推荐
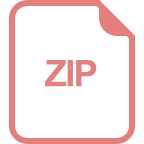
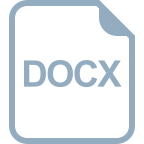
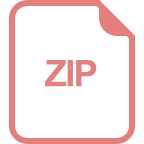













