matrix.empty()
时间: 2024-10-15 16:17:51 浏览: 2
矩阵.empty()通常是在编程中用于检查矩阵数据结构是否为空的操作,它通常是某个库函数提供的方法,比如NumPy(Python)或Pandas(Python)中的功能。这个函数会返回一个布尔值,如果矩阵中没有任何元素或者所有元素都被标记为缺失值,则返回True,表示矩阵是空的;反之则返回False。
例如,在NumPy中,你可以这样做:
```python
import numpy as np
# 创建一个空矩阵
matrix = np.empty((0, 0))
if matrix.empty():
print("Matrix is empty")
else:
print("Matrix is not empty")
# 或者创建一个非空矩阵
filled_matrix = np.array([[1, 2], [3, 4]])
if filled_matrix.empty():
print("Filled matrix is empty") # 这将不会打印
else:
print("Filled matrix is not empty")
```
相关问题
优化这段代码while (!potential_tracks.empty()) { int current_track = potential_tracksv[potential_tracksv.size() - 1]; potential_tracksv.pop_back(); // std::cout<<"current_track "<<current_track<<std::endl; potential_tracks.erase(current_track); for (int j = 0; j < cost_matrix.rows; ++j) { if (cost_matrix.at<int>(j, current_track) == 1) { // std::cout<<"visited_detections#### "<<j<<std::endl; setupdate(visited_detections, j); } } visited_tracks.insert(current_track); for (auto &det : visited_detections) { std::vector<int> connected_tracks; for (int j = 1; j < cost_matrix.cols; ++j) { if (cost_matrix.at<int>(det, j) == 1) { connected_tracks.push_back(j); } } for (auto tr : connected_tracks) { if (visited_tracks.count(tr) || potential_tracks.count(tr)) { continue; } potential_tracks.insert(tr); potential_tracksv.push_back(tr); } } }
这段代码的主要问题在于访问 potential_tracksv 和 visited_detections 容器时使用了循环,这样会导致在容器较大时的性能问题。另外,在每次迭代中都要执行 potential_tracks.erase(current_track) 操作,也会对性能造成一定的影响。
为了优化这段代码,可以采用迭代器来遍历容器,避免使用循环。另外,可以使用 erase 函数的返回值来删除元素,避免每次都要执行 erase 操作。具体的优化代码如下:
```c++
while (!potential_tracks.empty()) {
int current_track = *potential_tracks.rbegin();
potential_tracks.erase(--potential_tracks.end());
visited_tracks.insert(current_track);
for (int j = 0; j < cost_matrix.rows; ++j) {
if (cost_matrix.at<int>(j, current_track) == 1) {
setupdate(visited_detections, j);
}
}
for (auto it = visited_detections.begin(); it != visited_detections.end(); ) {
std::vector<int> connected_tracks;
for (int j = 1; j < cost_matrix.cols; ++j) {
if (cost_matrix.at<int>(*it, j) == 1) {
connected_tracks.push_back(j);
}
}
for (auto tr : connected_tracks) {
if (visited_tracks.count(tr) || potential_tracks.count(tr)) {
continue;
}
it = visited_detections.erase(it);
potential_tracks.insert(tr);
}
++it;
}
}
```
在优化后的代码中,采用了迭代器来遍历 visited_detections 容器,避免了使用循环。同时,在 visited_detections.erase(it) 操作后,使用了迭代器的后置递增运算符,避免了迭代器失效的问题。此外,使用了 potential_tracks.rbegin() 和 --potential_tracks.end() 来获取 potential_tracks 容器中的最后一个元素,避免了使用 vector 容器的 pop_back 操作。最后,将 visited_tracks.insert(current_track) 的操作提到了 potential_tracks.erase(current_track) 的前面,避免了重复插入已经访问过的轨迹。
error: (-215:Assertion failed) !_src.empty() in function 'cv::cvtColor'
This error message is related to the OpenCV library and occurs when the input image or matrix is empty. The assertion failed because the function cvtColor() expects a non-empty input image or matrix.
To fix this error, you should check if the input image or matrix is empty before passing it to the cvtColor() function. You can use the empty() function provided by OpenCV to check if the image or matrix is empty.
Here is an example of how to check if an image is empty before converting it to grayscale using cvtColor():
```
cv::Mat image = cv::imread("image.jpg");
if (!image.empty()) {
cv::Mat grayImage;
cv::cvtColor(image, grayImage, cv::COLOR_BGR2GRAY);
// do something with the grayscale image
} else {
std::cout << "Error: input image is empty!" << std::endl;
}
```
In this example, we first read an image from a file and check if it is empty using the empty() function. If the image is not empty, we convert it to grayscale using cvtColor(). If the image is empty, we print an error message.
相关推荐
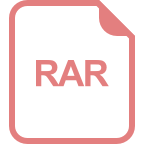
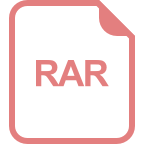
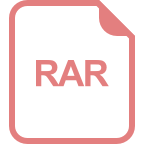











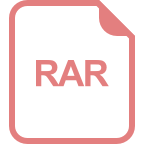
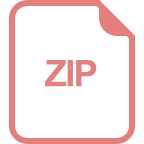