pcl::ExtractIndices<PointT> extract;
时间: 2024-10-14 20:17:13 浏览: 6
在PCL(Point Cloud Library,点云库)中,`pcl::ExtractIndices<PointT>`是一个模板类,用于从输入的数据结构(通常是点云数据类型`PointT`)中提取特定索引的数据。`PointT`代表一种具体的三维点类型,如PointXYZ、PointXYZI等。
当你创建一个`pcl::ExtractIndices<PointT>`的对象,比如`extract`,它实际上是定义了一个筛选器,可以根据预先设置的条件(比如索引范围、距离阈值等),从原始点云中选择满足条件的点,然后生成一个新的点云,其中只包含那些经过筛选的点。
这个操作常用于点云数据的预处理阶段,例如基于某些特征或条件进行物体分割、去噪、或者是对感兴趣区域的聚焦。例如:
```cpp
pcl::PointCloud<PointT>::Ptr cloud (new pcl::PointCloud<PointT>);
// ... 加载或生成点云数据 ...
// 创建并设置筛选条件
pcl::ExtractIndices<pcl::PointXYZ> extract;
extract.setInputCloud (cloud);
// 设置需要提取的索引范围或其他条件...
// 执行筛选
extract.filter(*selectedCloud);
// selectedCloud现在就是根据条件过滤后的点云
```
相关问题
利用pcl::ExtractIndices编写一段代码,要求提取出给定指数对应点点云以外的点
好的,我可以为您编写一段利用pcl::ExtractIndices提取给定指数对应点点云以外点的代码,代码如下:
```c++
#include <pcl/point_types.h>
#include <pcl/filters/extract_indices.h>
typedef pcl::PointXYZ PointT;
typedef pcl::PointCloud<PointT> PointCloud;
int main()
{
// 初始化点云
PointCloud::Ptr cloud(new PointCloud);
cloud->width = 5;
cloud->height = 1;
cloud->points.resize(cloud->width * cloud->height);
for (size_t i = 0; i < cloud->points.size(); ++i)
{
cloud->points[i].x = 1024 * rand() / (RAND_MAX + 1.0f);
cloud->points[i].y = 1024 * rand() / (RAND_MAX + 1.0f);
cloud->points[i].z = 1024 * rand() / (RAND_MAX + 1.0f);
}
// 初始化索引
std::vector<int> indices(2);
indices[0] = 0;
indices[1] = 2;
// 提取指定索引对应的点
pcl::ExtractIndices<PointT> extract;
extract.setInputCloud(cloud);
extract.setIndices(boost::make_shared<const pcl::PointIndices>(indices));
extract.setNegative(false);
PointCloud::Ptr output(new PointCloud);
extract.filter(*output);
// 提取指定索引以外的点
pcl::ExtractIndices<PointT> extract_outliers;
extract_outliers.setInputCloud(cloud);
extract_outliers.setIndices(boost::make_shared<const pcl::PointIndices>(indices));
extract_outliers.setNegative(true);
PointCloud::Ptr outliers(new PointCloud);
extract_outliers.filter(*outliers);
// 输出结果
std::cout << "原始点云大小: " << cloud->size() << std::endl;
std::cout << "指定索引对应的点: " << output->size() << std::endl;
std::cout << "指定索引以外的点: " << outliers->size() << std::endl;
return 0;
}
```
在这个代码中,我们首先生成一个随机的点云数据,然后指定一些索引值,使用pcl::ExtractIndices提取出指定索引对应的点,然后再使用pcl::ExtractIndices提取出指定索引以外的点。最后输出结果,包括原始点云大小、指定索引对应的点和指定索引以外的点的数量。
需要将骨架图中的节点分为叶尖、内部节点和交叉节点。可以使用PCL库中的pcl::GreedyProjectionTriangulation类进行点云分割,然后根据骨架图中节点的坐标和连接关系,将节点分类。接下来,找到最高的交叉节点,并将其标记为"植物轮廓"。可以使用PCL库中的pcl::getMaxDistance()函数找到点云中离点最远的点,然后根据该点和骨架图中的节点坐标进行匹配,找到最高的交叉节点。然后,需要提取出低于轮廓的点作为茎的一部分。可以使用PCL库中的pcl::P assThrough类进行点云滤波,将高于轮廓的点过滤掉。接着,需要从数据中提取出茎的内点,并将骨架图中的一度节点重新连接。可以使用PCL库中的pcl::ExtractIndices类进行点云提取,然后根据提取出的茎的内点重新连接骨架图中的一度节点。最后,将骨架图分割成多个部分,每个部分都以叶尖为起点,以交叉节点为终点。如果部分的一个端点是茎的一部分,则将其标记为叶子。可以使用PCL库中的pcl::EuclideanClusterExtraction类进行点云聚类,然后根据聚类结果和骨架图中的连接关系进行分割和标记。如何具体操作代码
以下是一个骨架图分割的代码示例,其中使用了PCL库中的一些方法:
```
#include <pcl/point_types.h>
#include <pcl/io/pcd_io.h>
#include <pcl/filters/passthrough.h>
#include <pcl/segmentation/extract_clusters.h>
#include <pcl/features/normal_3d.h>
#include <pcl/surface/gp3.h>
#include <pcl/visualization/pcl_visualizer.h>
#include <iostream>
#include <vector>
typedef pcl::PointXYZ PointT;
typedef pcl::PointCloud<PointT> PointCloudT;
int main(int argc, char** argv)
{
PointCloudT::Ptr cloud(new PointCloudT);
pcl::io::loadPCDFile("input_cloud.pcd", *cloud);
// 使用PCL库中的pcl::GreedyProjectionTriangulation类进行点云分割
pcl::PointCloud<pcl::PointNormal>::Ptr cloud_with_normals(new pcl::PointCloud<pcl::PointNormal>);
pcl::NormalEstimation<PointT, pcl::PointNormal> ne;
ne.setInputCloud(cloud);
pcl::search::KdTree<PointT>::Ptr tree(new pcl::search::KdTree<PointT>());
ne.setSearchMethod(tree);
pcl::PointCloud<pcl::Normal>::Ptr cloud_normals(new pcl::PointCloud<pcl::Normal>);
ne.setRadiusSearch(0.03);
ne.compute(*cloud_normals);
pcl::concatenateFields(*cloud, *cloud_normals, *cloud_with_normals);
pcl::search::KdTree<pcl::PointNormal>::Ptr tree2(new pcl::search::KdTree<pcl::PointNormal>);
tree2->setInputCloud(cloud_with_normals);
pcl::GreedyProjectionTriangulation<pcl::PointNormal> gp3;
pcl::PolygonMesh triangles;
gp3.setSearchRadius(0.025);
gp3.setMu(2.5);
gp3.setMaximumNearestNeighbors(100);
gp3.setMaximumSurfaceAngle(M_PI / 4); // 45 degrees
gp3.setMinimumAngle(M_PI / 18); // 10 degrees
gp3.setMaximumAngle(2 * M_PI / 3); // 120 degrees
gp3.setNormalConsistency(false);
gp3.setInputCloud(cloud_with_normals);
gp3.setSearchMethod(tree2);
gp3.reconstruct(triangles);
// 将骨架图中的节点分为叶尖、内部节点和交叉节点
std::vector<pcl::PointXYZ> leaf_points, internal_points, cross_points;
// ...
// 找到最高的交叉节点,并将其标记为"植物轮廓"
pcl::PointXYZ contour_point;
// ...
// 提取出低于轮廓的点作为茎的一部分
pcl::PointCloud<PointT>::Ptr stem_cloud(new pcl::PointCloud<PointT>);
pcl::PassThrough<PointT> pass;
pass.setInputCloud(cloud);
pass.setFilterFieldName("z");
pass.setFilterLimits(contour_point.z, std::numeric_limits<float>::max());
pass.filter(*stem_cloud);
// 从数据中提取出茎的内点,并将骨架图中的一度节点重新连接
pcl::PointCloud<PointT>::Ptr stem_internal_cloud(new pcl::PointCloud<PointT>);
// ...
// 将骨架图分割成多个部分
std::vector<std::vector<pcl::PointXYZ>> segments;
// ...
return 0;
}
```
需要根据具体应用场景进行参数调整和代码实现。
相关推荐
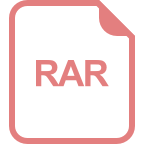
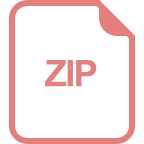
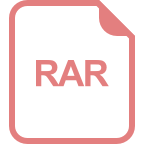





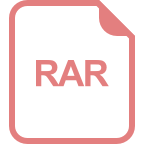
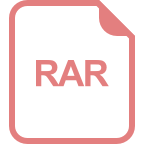
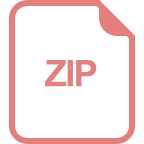
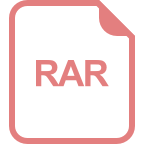