read.csv("D:\R\Regression-model-master\练习数据 data\student_data.csv")有什么错误
时间: 2023-12-02 07:05:29 浏览: 79
这个命令可能会报错,因为在Windows系统中,文件路径中的反斜杠 `\` 会被解释为转义字符。可以使用双反斜杠 `\\` 或者正斜杠 `/` 来代替,例如:
```
read.csv("D:/R/Regression-model-master/练习数据 data/student_data.csv")
```
或者:
```
read.csv("D:\\R\\Regression-model-master\\练习数据 data\\student_data.csv")
```
相关问题
import pandas as pd import matplotlib.pyplot as plt from sklearn.linear_model import LinearRegression data = pd.read_csv('/home/w123/Documents/fatigue_detecting-master/TXT-data/5.14/2/Eye aspect ratio.txt') y = data.iloc[:, :-1].values.reshape(-1, 1) X = data.iloc[:, -1].values.reshape(-1, 1) regressor = LinearRegression() regressor.fit(X, y) y_pred = regressor.predict(X) print("Regression Function: y = {:.2f} + {:.2f}x".format(regressor.intercept_[0], regressor.coef_[0][0])) plt.scatter(X, y, color='blue') plt.plot(X, y_pred, color='red') plt.title('Linear Regression') plt.xlabel('Independent Variable') plt.ylabel('Dependent Variable') plt.legend(['Regression Line', 'Observations']) # 画第二个图 data2 = pd.read_csv('/home/w123/Documents/fatigue_detecting-master/TXT-data/5.14/2/Another file.txt') y2 = data2.iloc[:, :-1].values.reshape(-1, 1) X2 = data2.iloc[:, -1].values.reshape(-1, 1) regressor2 = LinearRegression() regressor2.fit(X2, y2) y_pred2 = regressor2.predict(X2) print("Regression Function: y = {:.2f} + {:.2f}x".format(regressor2.intercept_[0], regressor2.coef_[0][0])) plt.scatter(X2, y2, color='green') plt.plot(X2, y_pred2, color='orange') plt.legend(['Regression Line 2', 'Observations 2']) plt.show()再加一个文件
你需要将第三个文件的路径传递给程序,然后对其进行类似的处理。以下是修改后的代码:
```
import pandas as pd
import matplotlib.pyplot as plt
from sklearn.linear_model import LinearRegression
# 读取第一个文件
data = pd.read_csv('/home/w123/Documents/fatigue_detecting-master/TXT-data/5.14/2/Eye aspect ratio.txt')
y = data.iloc[:, :-1].values.reshape(-1, 1)
X = data.iloc[:, -1].values.reshape(-1, 1)
regressor = LinearRegression()
regressor.fit(X, y)
y_pred = regressor.predict(X)
print("Regression Function 1: y = {:.2f} + {:.2f}x".format(regressor.intercept_[0], regressor.coef_[0][0]))
# 画第一个图
plt.scatter(X, y, color='blue')
plt.plot(X, y_pred, color='red')
# 读取第二个文件
data2 = pd.read_csv('/home/w123/Documents/fatigue_detecting-master/TXT-data/5.14/2/Another file.txt')
y2 = data2.iloc[:, :-1].values.reshape(-1, 1)
X2 = data2.iloc[:, -1].values.reshape(-1, 1)
regressor2 = LinearRegression()
regressor2.fit(X2, y2)
y_pred2 = regressor2.predict(X2)
print("Regression Function 2: y = {:.2f} + {:.2f}x".format(regressor2.intercept_[0], regressor2.coef_[0][0]))
# 画第二个图
plt.scatter(X2, y2, color='green')
plt.plot(X2, y_pred2, color='orange')
# 读取第三个文件
data3 = pd.read_csv('/path/to/third/file.txt')
y3 = data3.iloc[:, :-1].values.reshape(-1, 1)
X3 = data3.iloc[:, -1].values.reshape(-1, 1)
regressor3 = LinearRegression()
regressor3.fit(X3, y3)
y_pred3 = regressor3.predict(X3)
print("Regression Function 3: y = {:.2f} + {:.2f}x".format(regressor3.intercept_[0], regressor3.coef_[0][0]))
# 画第三个图
plt.scatter(X3, y3, color='purple')
plt.plot(X3, y_pred3, color='yellow')
plt.title('Linear Regression')
plt.xlabel('Independent Variable')
plt.ylabel('Dependent Variable')
plt.legend(['Regression Line 1', 'Observations 1', 'Regression Line 2', 'Observations 2', 'Regression Line 3', 'Observations 3'])
plt.show()
```
请将`/path/to/third/file.txt`替换为实际的第三个文件路径。
import pandas as pdfrom sklearn.model_selection import train_test_splitfrom sklearn.preprocessing import PolynomialFeaturesfrom sklearn.linear_model import LinearRegression# 读取数据data = pd.read_csv('data.csv')# 分离自变量和因变量X = data.iloc[:, :-1].valuesy = data.iloc[:, -1].values# 数据集划分为训练集和测试集X_train, X_test, y_train, y_test = train_test_split(X, y, test_size=0.2)# 使用多项式回归模型poly_reg = PolynomialFeatures(degree=2)X_poly = poly_reg.fit_transform(X_train)# 训练模型regressor = LinearRegression()regressor.fit(X_poly, y_train)# 预测结果y_pred = regressor.predict(poly_reg.transform(X_test))最后如何绘制图
可以使用Matplotlib库来绘制散点图和回归线。以下是一个简单的示例代码:
```
import matplotlib.pyplot as plt
# 绘制训练集散点图
plt.scatter(X_train, y_train, color='blue')
# 绘制回归线
x = np.linspace(X_train.min(), X_train.max(), 100)
y = regressor.predict(poly_reg.fit_transform(x.reshape(-1, 1)))
plt.plot(x, y, color='red')
# 设置图形标题和坐标轴标签
plt.title('Polynomial Regression')
plt.xlabel('X')
plt.ylabel('Y')
# 显示图形
plt.show()
```
注意,这只是一个示例代码,具体绘图方式可以根据实际需求进行调整。
阅读全文
相关推荐
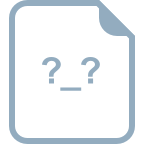
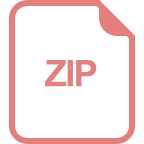













