torch中怎么将array(['结构紧凑', '承载能力强', '加工机器人', '0.1'], dtype='<U20')这样的numpy数据embedding
时间: 2023-08-06 16:26:26 浏览: 45
在PyTorch中,可以使用`torch.nn.Embedding`模块来进行embedding操作。但是,`torch.nn.Embedding`模块需要输入的是整数类型的Tensor,而不是字符串或浮点数类型的Tensor。所以,在将字符串或浮点数类型的Tensor进行embedding之前,需要先将其转换为整数类型的Tensor。
以下是一个示例代码,用于将字符串类型的Tensor转换为整数类型的Tensor,并进行embedding操作:
```python
import numpy as np
import torch
# 定义一个字符串类型的Tensor
str_tensor = torch.tensor(['结构紧凑', '承载能力强', '加工机器人', '0.1'])
# 将字符串类型的Tensor转换为整数类型的Tensor
word_to_ix = {}
for word in str_tensor:
if word not in word_to_ix:
word_to_ix[word] = len(word_to_ix)
ix_to_word = {ix: word for word, ix in word_to_ix.items()}
int_tensor = torch.tensor([word_to_ix[word] for word in str_tensor], dtype=torch.long)
# 定义embedding层
embedding_layer = torch.nn.Embedding(len(word_to_ix), 10)
# 进行embedding操作
embedded_tensor = embedding_layer(int_tensor)
print(embedded_tensor)
```
在上面的示例代码中,我们首先将字符串类型的Tensor转换为整数类型的Tensor,其中`word_to_ix`字典用于将每个字符串映射到一个整数,`ix_to_word`字典用于将整数映射回原来的字符串。然后,我们定义了一个embedding层,其中`len(word_to_ix)`表示embedding层的输入大小,`10`表示embedding层的输出大小。最后,我们使用`embedding_layer`对整数类型的Tensor进行embedding操作,得到嵌入后的Tensor。
需要注意的是,上面示例代码中,我们使用了固定的embedding层输入和输出大小。在实际应用中,这些大小需要根据数据集的特点进行调整。
相关推荐
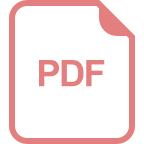
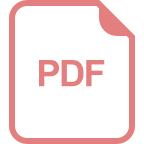
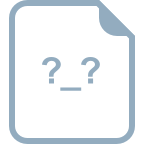














