fig = plt.figure(layout='constrained', figsize=(10, 8))的layout
时间: 2023-12-05 18:04:11 浏览: 230
参数设置为'constrained'时,会启用约束布局,这意味着所有子图将被自动调整为相同的大小和位置。每个子图将被放置在一个矩形内,该矩形的大小将由最大子图的大小决定。如果子图的大小不同,则它们将被调整为相同的大小,以适应最大子图的大小。此布局可确保所有子图具有相同的大小和位置,从而使它们更易于比较和解释。它在多个子图的情况下非常有用。
相关问题
#The fit of the methods must be done only using the real sequential data pca.fit(stock_data_reduced) pca_real = pd.DataFrame(pca.transform(stock_data_reduced)) pca_synth = pd.DataFrame(pca.transform(synth_data_reduced)) data_reduced = np.concatenate((stock_data_reduced, synth_data_reduced), axis=0) tsne_results = pd.DataFrame(tsne.fit_transform(data_reduced)) fig = plt.figure(constrained_layout=True, figsize=(20,10)) spec = gridspec.GridSpec(ncols=2, nrows=1, figure=fig)
这段代码是用于拟合主成分分析(PCA)和 t-分布随机近邻嵌入(t-SNE)的方法,并将结果可视化出来。
首先,使用`pca.fit()`方法对降维后的真实数据`stock_data_reduced`进行拟合,得到PCA模型。
接下来,使用`pca.transform()`方法将真实数据和合成数据分别转换为PCA的结果。使用`pd.DataFrame()`将转换后的结果转换为DataFrame格式,并分别保存在`pca_real`和`pca_synth`中。
然后,将真实数据和合成数据在降维后的空间中进行拼接,得到`data_reduced`。使用`tsne.fit_transform()`方法对拼接后的数据进行t-SNE降维,得到t-SNE的结果。同样地,使用`pd.DataFrame()`将转换后的结果转换为DataFrame格式,并保存在`tsne_results`中。
最后,创建一个大小为(20,10)的图形窗口,并使用`gridspec.GridSpec()`设置图形的网格布局。在这个例子中,将创建一个1行2列的网格布局。
这段代码的目的是使用PCA和t-SNE对真实数据进行降维,并将结果可视化出来。在可视化中,真实数据和合成数据在降维后的空间中被表示为不同的点集,可以用于比较它们在特征空间中的分布情况。
import numpy as np import matplotlib.pyplot as plt import matplotlib.gridspec as gridspec plt.rcParams["font.sans-serif"] = ["SimHei"] x_month = np.array(['1月', '2月', '3月', '4月', '5月', '6月']) y_sales = np.array([2150, 1050, 1560, 1480, 1530, 1490]) x_citys = np.array(['北京', '上海', '广州', '深圳', '浙江', '山东']) y_sale_count = np.array([83775, 62860, 59176, 64205, 48671, 39968]) # 创建画布和布局 fig = plt.figure(constrained_layout=True) gs = fig.add_gridspec(2, 2) ax_one = fig.add_subplot(gs[0, :]) ax_two = fig.add_subplot(gs[1, 0]) ax_thr = fig.add_subplot(gs[1, 1])优化为饼状图
这段代码导入了numpy、matplotlib.pyplot、matplotlib.gridspec模块,并将字体设置为“SimHei”。然后使用numpy创建了一个月份数组x_month和一个销售额数组y_sales,以及一个城市名数组x_citys和一个销售统计数量数组y_sale_count。
阅读全文
相关推荐
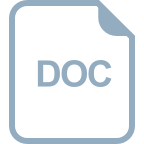
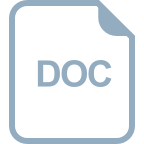
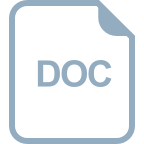






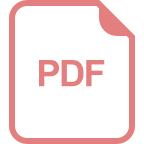




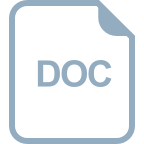
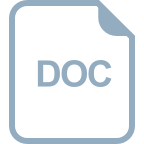