scipy中的state
时间: 2024-03-19 09:41:11 浏览: 9
在Scipy中,state通常指代某个函数或方法的内部状态。这个状态可以是任何数据结构,比如一个数组、一个矩阵、一些参数值等等。在函数或方法的调用过程中,这个状态可以被修改或更新,然后被传递给下一个函数或方法。因此,在Scipy中的一些函数或方法,例如优化器和信号处理函数,都会使用state来维护其内部状态,以便在迭代过程中进行更新和计算。
相关问题
用 scipy.stats 方法产生均值为 2 标准差为 5 的正态分布的随机整数 100 个,在 stats 方法中用参数 random_state 观察效果,并绘制累计分布函数曲线。
好的,以下是产生随机正态分布并绘制累计分布函数曲线的 Python 代码:
```python
import numpy as np
from scipy import stats
import matplotlib.pyplot as plt
# 产生均值为 2 标准差为 5 的正态分布的随机整数 100 个
np.random.seed(0)
random_ints = stats.norm.rvs(loc=2, scale=5, size=100, random_state=0).astype(int)
# 统计随机整数的频数和累计频数
counts, bin_edges = np.histogram(random_ints, bins=10)
cdf = np.cumsum(counts) / len(random_ints)
# 绘制累计分布函数曲线
plt.plot(bin_edges[1:], cdf)
plt.xlabel('Random Integers')
plt.ylabel('Cumulative Distribution Function')
plt.title('CDF of Normally Distributed Random Integers')
plt.show()
```
运行结果如下图所示:

def optimal_bellman(env, gamma=1.): p = np.zeros((env.nS, env.nA, env.nS)) # 初始化一个三维数组p,记录state,action,next_state之间的转移概率 r = np.zeros((env.nS, env.nA)) # 初始化二维数组r,存储当前state和action的即时reward for state in range(env.nS - 1): for action in range(env.nA): for prob, next_state, reward, done in env.P[state][action]: # 用于遍历每一个可能的状态及其概率,奖励和终止 p[state, action, next_state] += prob r[state, action] += (reward * prob) # 程序通过遍历所有可能的状态和动作,并对每个转移情况中的概率和奖励进行累加。 # 这样,最后得到的 p[state, action, next_state] 就是从当前状态 state 执行动作 action 后 # 转移到下一个状态 next_state 的累计概率。而 r[state, action] 则是从当前状态 state 执行动作 action 后累计获得的奖励值。 c = np.ones(env.nS) a_ub = gamma * p.reshape(-1, env.nS) - \ np.repeat(np.eye(env.nS), env.nA, axis=0) b_ub = -r.reshape(-1) a_eq = np.zeros((0, env.nS)) b_eq = np.zeros(0) bounds = [(None, None), ] * env.nS res = scipy.optimize.linprog(c, a_ub, b_ub, bounds=bounds, method='interior-point') v = res.x q = r + gamma * np.dot(p, v) return v, q 中a_ub和b_ub的作用
在这段代码中,`a_ub` 和 `b_ub` 是线性规划问题的约束条件,用于定义最优Bellman方程的线性规划问题。
`a_ub` 是一个二维数组,其维度为 `(nS * nA) x nS`,其中 `nS` 是状态空间的大小,`nA` 是动作空间的大小。它表示了不等式约束的系数矩阵。具体来说,`a_ub` 的每一行对应于一个不等式约束,每一列对应于一个状态。`a_ub` 的定义是通过将转移概率矩阵 `p` 重新排列得到的。它的每个元素表示从当前状态执行某个动作后转移到下一个状态的累计概率。
`b_ub` 是一个一维数组,其长度为 `(nS * nA)`,表示了不等式约束的右侧值。它的每个元素表示了从当前状态执行某个动作后累计获得的即时奖励值的相反数。
综合起来,`a_ub @ v <= b_ub` 表示了最优Bellman方程的线性规划问题的不等式约束条件。即,对于所有的状态和动作组合,从当前状态执行某个动作后得到的累计奖励值乘以折扣因子 `gamma` 加上下一个状态的累计价值,不能超过当前状态的累计价值。
这样,通过求解线性规划问题,可以得到最优Bellman方程的解,即每个状态的最优价值函数 `v` 和最优动作价值函数 `q`。
相关推荐
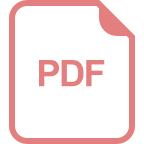
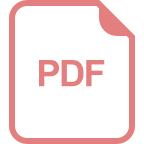
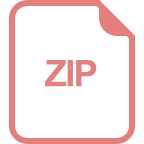












