深度可分离卷积pytorch代码
时间: 2024-02-29 17:50:02 浏览: 27
深度可分离卷积是一种卷积神经网络中常用的操作,它可以有效地减少模型的参数量和计算量,从而提高模型的效率和性能。在PyTorch中,可以使用torch.nn模块中的nn.Conv2d函数来实现深度可分离卷积。
下面是一个使用PyTorch实现深度可分离卷积的示例代码:
```python
import torch
import torch.nn as nn
# 定义一个包含深度可分离卷积的网络模型
class SeparableConvNet(nn.Module):
def __init__(self, in_channels, out_channels):
super(SeparableConvNet, self).__init__()
# 深度可分离卷积由深度卷积和逐点卷积两部分组成
self.depthwise_conv = nn.Conv2d(in_channels, in_channels, kernel_size=3, padding=1, groups=in_channels)
self.pointwise_conv = nn.Conv2d(in_channels, out_channels, kernel_size=1)
def forward(self, x):
x = self.depthwise_conv(x)
x = self.pointwise_conv(x)
return x
# 创建一个输入张量
input_tensor = torch.randn(1, 3, 32, 32)
# 创建一个深度可分离卷积模型
model = SeparableConvNet(3, 64)
# 将输入张量传入模型进行前向计算
output_tensor = model(input_tensor)
print(output_tensor.shape) # 输出张量的形状
```
在上面的代码中,我们首先定义了一个名为SeparableConvNet的网络模型,其中包含了深度可分离卷积的两个部分:深度卷积和逐点卷积。然后,我们创建了一个输入张量input_tensor,并将其传入模型进行前向计算,得到输出张量output_tensor。最后,我们打印输出张量的形状。
希望以上代码对你有所帮助!
相关推荐
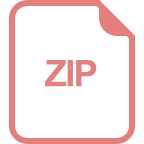
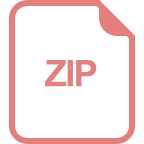
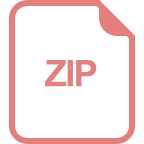














