lags=9 ncols=3 nrows=int(np.ceil(lags/ncols)) fig, axes = plt.subplots(ncols=ncols, nrows=nrows, figsize=(4*ncols, 4*nrows))
时间: 2023-05-20 16:07:08 浏览: 72
这是一段 Python 代码,用于创建一个包含多个子图的图形。其中,lags、ncols 和 nrows 都是变量,分别表示子图的数量、列数和行数。fig 和 axes 是 matplotlib 库中的对象,用于创建和管理图形和子图。这段代码的作用是创建一个包含 ncols 列、nrows 行的子图矩阵,每个子图的大小为 4*ncols x 4*nrows。
相关问题
fig = sm.graphics.tsa.plot_acf(residuals, lags=30, ax=ax[0])之前需要什么代码
在使用 `fig = sm.graphics.tsa.plot_acf(residuals, lags=30, ax=ax[0])` 之前,需要先进行如下步骤:
1. 导入 `statsmodels` 库:`import statsmodels.api as sm`
2. 计算时间序列的残差:`residuals = model.resid`
3. 创建一个图像对象:`fig, ax = plt.subplots(1, 2, figsize=(15, 5))`
这里假设你已经导入了 `matplotlib` 库,可以使用 `plt.subplots()` 函数创建一个包含两个子图的图像对象。其中,第一个子图用于绘制自相关图,第二个子图用于绘制偏自相关图。如果你只需要绘制自相关图,可以将 `ax=ax[0]` 省略。完整的代码示例如下:
```
import statsmodels.api as sm
import matplotlib.pyplot as plt
# 计算时间序列的残差
residuals = model.resid
# 创建一个图像对象
fig, ax = plt.subplots(1, 2, figsize=(15, 5))
# 绘制自相关图
sm.graphics.tsa.plot_acf(residuals, lags=30, ax=ax[0])
# 绘制偏自相关图
sm.graphics.tsa.plot_pacf(residuals, lags=30, ax=ax[1])
# 显示图像
plt.show()
```
plot_acf(ycz,lags=20,color='c'
plot_acf()函数是Matplotlib库中的一个函数,用于绘制时序数据的自相关图。该函数的参数说明如下:
- ycz:一个一维时序数据数组,用于绘制自相关图。
- lags:一个整数值,表示要绘制的滞后阶数,即自相关图中显示的横轴范围,默认值为20。
- color:一个字符串,表示自相关图的颜色,默认为‘c’(青色)。
以下是一个示例代码,展示如何使用plot_acf()函数绘制自相关图:
```python
import matplotlib.pyplot as plt
from statsmodels.graphics.tsaplots import plot_acf
import pandas as pd
# 读取时序数据
data = pd.read_csv('data.csv', index_col=0, parse_dates=True)
ycz = data['ycz']
# 绘制自相关图
fig, ax = plt.subplots(figsize=(8, 4))
plot_acf(ycz, lags=20, color='c', ax=ax)
# 显示图形
plt.show()
```
在上面的代码中,我们首先使用pandas库读取了一个名为“data.csv”的时序数据文件,并从中选择了名为“ycz”的一维数据数组。然后,我们创建了一个大小为8x4英寸的Figure对象和一个Axes对象,并使用plot_acf()函数在Axes对象上绘制了自相关图。最后,我们使用plt.show()函数显示图形。
相关推荐
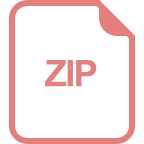
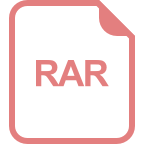
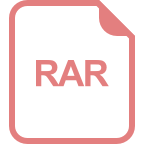












