c45决策树算法python
时间: 2024-09-29 16:14:46 浏览: 45
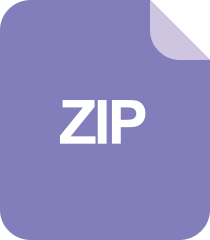
C45决策树算法 C45决策树算法
C4.5决策树算法是一种基于信息增益的分类算法,由Ross Quinlan在ID3基础上发展而来。它通过计算属性对数据集划分的信息增益或信息增益比,选择最优属性作为当前节点的分裂依据,递归地构建决策树。
在Python中,你可以使用scikit-learn库来实现C4.5决策树。以下是一个简单的例子:
```python
from sklearn.tree import DecisionTreeClassifier
import pandas as pd
# 假设你已经有了一个DataFrame 'data',其中包含特征列和目标列
X = data.iloc[:, :-1] # 特征
y = data.iloc[:, -1] # 目标变量
# 创建并训练C4.5决策树模型
clf = DecisionTreeClassifier(criterion='gini', splitter='best') # 'gini' 是信息熵的一种变体
clf.fit(X, y)
# 对新的数据进行预测
new_data = ... # 新的数据点
prediction = clf.predict(new_data)
```
阅读全文
相关推荐
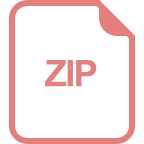
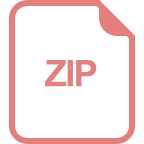



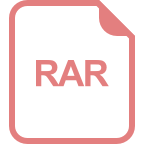
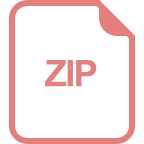
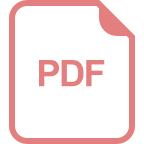
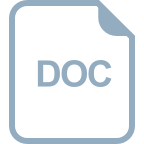
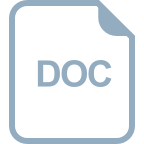



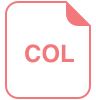
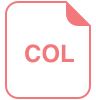

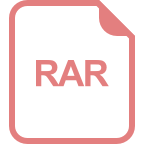
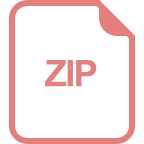