帮我润色论文。In this paper, we propose a balancing training method to address problems in imbalanced data learning. To this end, we derive a new loss used in the balancing training phase that alleviates the influence of samples that cause an overfitted decision boundary. The proposed loss efficiently improves the performance of any type of imbalance learning methods. In experiments on multiple benchmark data sets, we demonstrate the validity of our method and reveal that the proposed loss outperforms the state-of-the-art cost-sensitive loss methods. Furthermore, since our loss is not restricted to a specific task, model, or training method, it can be easily used in combination with other recent resampling, meta-learning, and cost-sensitive learning methods for class-imbalance problems. Our code is made available at https://github.com/pseulki/IB-Loss.
时间: 2023-02-24 13:02:14 浏览: 323
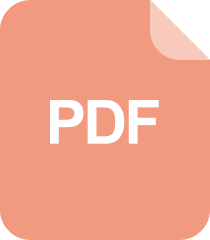
Development of Fatigue Driving Detection Method Based on Fuzzy Control Logic
在本文中,我们提出了一种平衡训练方法,以解决不平衡数据学习中的问题。为此,我们在平衡训练阶段推导出一种新的损失,以减少导致过度拟合决策边界的样本的影响。所提出的损失有效提高了任何类型的不平衡学习方法的性能。在多个基准数据集的实验中,我们证明了我们的方法的有效性,并发现所提出的损失优于最先进的成本敏感损失方法。此外,由于我们的损失不受特定任务、模型或训练方法的限制,它可以轻松与其他最近的重采样、元学习和成本敏感学习方法结合使用,以解决类不平衡问题。我们的代码可在 https://github.com/pseulki/IB-Loss 获得。
阅读全文
相关推荐
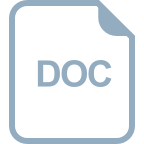
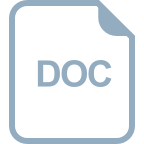










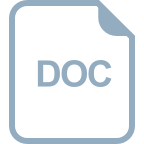
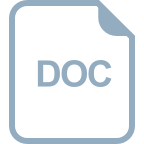