data = np.reshape(data, (num, length))
时间: 2024-06-06 08:11:22 浏览: 10
This line of code reshapes the numpy array "data" into a new shape specified by the tuple (num, length).
The new shape has "num" rows and "length" columns, where "num" and "length" are variables that should be defined previously in the code. The data in the original array is rearranged to fit this new shape, so the total number of elements in the reshaped array must be the same as the original array.
This kind of reshaping is useful when we want to work with data in a particular format, such as when we want to treat a long list of values as a matrix with a specific number of rows and columns.
相关问题
import random import numpy as np import scipy.io as scio from sklearn import preprocessing from keras.utils import np_utils def deal_data(data,length,label): data = np.reshape(data,(-1)) num = len(data)//length data = data[0:num*length] data = np.reshape(data,(num,length)) min_max_scaler = preprocessing.MinMaxScaler() data = min_max_scaler.fit_transform(np.transpose(data,[1,0])) data = np.transpose(data,[1,0]) label = np.ones((num,1))*label return np.column_stack((data,label)) 解释下每一段代码的含义
这是一个处理数据的函数,具体含义如下:
1. `import random`: 导入random模块,用于生成随机数。
2. `import numpy as np`: 导入numpy模块,并给它取一个别名np,用于进行数组操作。
3. `import scipy.io as scio`: 导入scipy模块中的io子模块,并给它取一个别名scio,用于读取.mat文件。
4. `from sklearn import preprocessing`: 从sklearn模块中导入preprocessing子模块,用于数据预处理。
5. `from keras.utils import np_utils`: 从keras.utils模块中导入np_utils子模块,用于独热编码。
6. `def deal_data(data,length,label):`:定义一个函数deal_data,该函数的参数为data、length和label。
7. `data = np.reshape(data,(-1))`: 将data数组按照一维数组进行重塑。
8. `num = len(data)//length`: 计算data数组中能够组成多少个长度为length的数组。
9. `data = data[0:num*length]`: 将data数组截取为长度为length的倍数。
10. `data = np.reshape(data,(num,length))`: 将data数组按照长度为length进行重塑。
11. `min_max_scaler = preprocessing.MinMaxScaler()`: 创建一个MinMaxScaler的实例。
12. `data = min_max_scaler.fit_transform(np.transpose(data,[1,0]))`: 将data数组进行归一化处理。
13. `data = np.transpose(data,[1,0])`: 将data数组进行转置操作。
14. `label = np.ones((num,1))*label`: 创建一个元素均为label的num行1列的数组。
15. `return np.column_stack((data,label))`: 将data和label按列方向进行合并,返回合并后的数组。
import tensorflow as tf import tensorflow_hub as hub from tensorflow.keras import layers import bert import numpy as np from transformers import BertTokenizer, BertModel # 设置BERT模型的路径和参数 bert_path = "E:\\AAA\\523\\BERT-pytorch-master\\bert1.ckpt" max_seq_length = 128 train_batch_size = 32 learning_rate = 2e-5 num_train_epochs = 3 # 加载BERT模型 def create_model(): input_word_ids = tf.keras.layers.Input(shape=(max_seq_length,), dtype=tf.int32, name="input_word_ids") input_mask = tf.keras.layers.Input(shape=(max_seq_length,), dtype=tf.int32, name="input_mask") segment_ids = tf.keras.layers.Input(shape=(max_seq_length,), dtype=tf.int32, name="segment_ids") bert_layer = hub.KerasLayer(bert_path, trainable=True) pooled_output, sequence_output = bert_layer([input_word_ids, input_mask, segment_ids]) output = layers.Dense(1, activation='sigmoid')(pooled_output) model = tf.keras.models.Model(inputs=[input_word_ids, input_mask, segment_ids], outputs=output) return model # 准备数据 def create_input_data(sentences, labels): tokenizer = bert.tokenization.FullTokenizer(vocab_file=bert_path + "trainer/vocab.small", do_lower_case=True) # tokenizer = BertTokenizer.from_pretrained('bert-base-uncased') input_ids = [] input_masks = [] segment_ids = [] for sentence in sentences: tokens = tokenizer.tokenize(sentence) tokens = ["[CLS]"] + tokens + ["[SEP]"] input_id = tokenizer.convert_tokens_to_ids(tokens) input_mask = [1] * len(input_id) segment_id = [0] * len(input_id) padding_length = max_seq_length - len(input_id) input_id += [0] * padding_length input_mask += [0] * padding_length segment_id += [0] * padding_length input_ids.append(input_id) input_masks.append(input_mask) segment_ids.append(segment_id) return np.array(input_ids), np.array(input_masks), np.array(segment_ids), np.array(labels) # 加载训练数据 train_sentences = ["Example sentence 1", "Example sentence 2", ...] train_labels = [0, 1, ...] train_input_ids, train_input_masks, train_segment_ids, train_labels = create_input_data(train_sentences, train_labels) # 构建模型 model = create_model() model.compile(optimizer=tf.keras.optimizers.Adam(lr=learning_rate), loss='binary_crossentropy', metrics=['accuracy']) # 开始微调 model.fit([train_input_ids, train_input_masks, train_segment_ids], train_labels, batch_size=train_batch_size, epochs=num_train_epochs)这段代码有什么问题吗?
这段代码存在一些问题:
1. `bert_path` 的值不应该是 ".ckpt" 结尾的文件路径,而是BERT模型的目录路径,例如:`bert_path = "E:\\AAA\\523\\BERT-pytorch-master\\"`。
2. `vocab_file` 参数应该是BERT模型目录下的 "vocab.txt" 文件路径,而不是训练器目录下的 "vocab.small" 文件路径。例如:`tokenizer = bert.tokenization.FullTokenizer(vocab_file=bert_path + "vocab.txt", do_lower_case=True)`。
3. `create_input_data` 函数中的 `tokenizer` 对象应该是从 `BertTokenizer` 类中导入的。因此,需要将 `from transformers import BertTokenizer` 加入代码开头,并使用 `BertTokenizer.from_pretrained()` 函数来加载BERT的tokenizer。
4. `train_sentences` 和 `train_labels` 应该是训练数据集,但是在代码中缺失了这些数据的定义和加载。
5. `create_input_data` 函数返回的 `labels` 应该是一维的,而不是二维的。因此,需要修改函数 `return` 语句为:`return np.array(input_ids), np.array(input_masks), np.array(segment_ids), np.array(labels).reshape(-1)`。
6. 在 `model.fit` 中,需要指定验证集的参数 `validation_data`,以便在训练过程中评估模型的性能。
综上所述,以下是修改后的代码示例:
```
import tensorflow as tf
import tensorflow_hub as hub
from tensorflow.keras import layers
import bert
import numpy as np
from transformers import BertTokenizer, BertModel
# 设置BERT模型的路径和参数
bert_path = "E:\\AAA\\523\\BERT-pytorch-master\\"
max_seq_length = 128
train_batch_size = 32
learning_rate = 2e-5
num_train_epochs = 3
# 加载BERT模型
def create_model():
input_word_ids = tf.keras.layers.Input(shape=(max_seq_length,), dtype=tf.int32, name="input_word_ids")
input_mask = tf.keras.layers.Input(shape=(max_seq_length,), dtype=tf.int32, name="input_mask")
segment_ids = tf.keras.layers.Input(shape=(max_seq_length,), dtype=tf.int32, name="segment_ids")
bert_layer = hub.KerasLayer(bert_path, trainable=True)
pooled_output, sequence_output = bert_layer([input_word_ids, input_mask, segment_ids])
output = layers.Dense(1, activation='sigmoid')(pooled_output)
model = tf.keras.models.Model(inputs=[input_word_ids, input_mask, segment_ids], outputs=output)
return model
# 准备数据
def create_input_data(sentences, labels):
tokenizer = BertTokenizer.from_pretrained('bert-base-uncased')
input_ids = []
input_masks = []
segment_ids = []
for sentence in sentences:
tokens = tokenizer.tokenize(sentence)
tokens = ["[CLS]"] + tokens + ["[SEP]"]
input_id = tokenizer.convert_tokens_to_ids(tokens)
input_mask = [1] * len(input_id)
segment_id = [0] * len(input_id)
padding_length = max_seq_length - len(input_id)
input_id += [0] * padding_length
input_mask += [0] * padding_length
segment_id += [0] * padding_length
input_ids.append(input_id)
input_masks.append(input_mask)
segment_ids.append(segment_id)
return np.array(input_ids), np.array(input_masks), np.array(segment_ids), np.array(labels).reshape(-1)
# 加载训练数据
train_sentences = ["Example sentence 1", "Example sentence 2", ...]
train_labels = [0, 1, ...]
train_input_ids, train_input_masks, train_segment_ids, train_labels = create_input_data(train_sentences, train_labels)
# 构建模型
model = create_model()
model.compile(optimizer=tf.keras.optimizers.Adam(lr=learning_rate),
loss='binary_crossentropy',
metrics=['accuracy'])
# 开始微调
model.fit([train_input_ids, train_input_masks, train_segment_ids], train_labels,
batch_size=train_batch_size,
epochs=num_train_epochs,
validation_data=([val_input_ids, val_input_masks, val_segment_ids], val_labels))
```
相关推荐
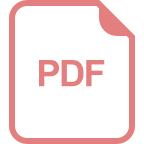
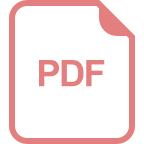
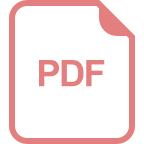












