我需要一个源语言为python,利用PCA对人脸数据集进行降维的代码
时间: 2024-04-30 21:22:19 浏览: 191
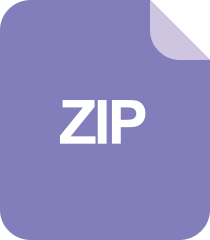
基于python的PCA人脸识别算法+源代码+文档说明
以下是一个源语言为Python的利用PCA对人脸数据集进行降维的代码:
```python
import numpy as np
import matplotlib.pyplot as plt
from sklearn.decomposition import PCA
from sklearn.datasets import fetch_lfw_people
# 加载人脸数据集
lfw_people = fetch_lfw_people(min_faces_per_person=70, resize=0.4)
X = lfw_people.data
n_samples, n_features = X.shape
# 显示原始图像
def plot_gallery(images, titles, h, w, n_row=3, n_col=4):
plt.figure(figsize=(1.8 * n_col, 2.4 * n_row))
plt.subplots_adjust(bottom=0, left=0.01, right=0.99, top=0.90, hspace=0.35)
for i in range(n_row * n_col):
plt.subplot(n_row, n_col, i + 1)
plt.imshow(images[i].reshape((h, w)), cmap=plt.cm.gray)
plt.title(titles[i], size=12)
plt.xticks(())
plt.yticks(())
plot_gallery(X, lfw_people.target_names, 50, 37)
# 进行PCA降维
n_components = 150
pca = PCA(n_components=n_components, svd_solver='randomized', whiten=True)
pca.fit(X)
# 显示降维后的图像
eigenfaces = pca.components_.reshape((n_components, 50, 37))
plot_gallery(eigenfaces, range(n_components), 50, 37)
# 显示解释方差比
plt.figure()
plt.plot(np.cumsum(pca.explained_variance_ratio_))
plt.xlabel('Number of components')
plt.ylabel('Cumulative explained variance')
plt.show()
```
该代码使用了`sklearn`库中的`PCA`类进行降维。在代码中,首先加载了人脸数据集,然后使用`plot_gallery`函数显示了原始图像。接着,使用`PCA`类进行降维,并使用`plot_gallery`函数显示了降维后的图像。最后,使用`plt.plot`函数显示了解释方差比。
阅读全文
相关推荐
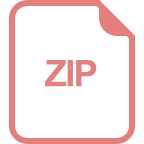
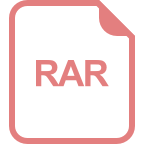
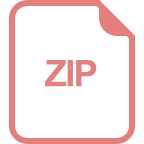
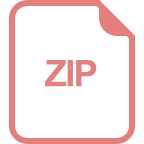
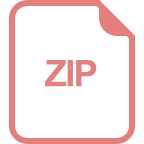
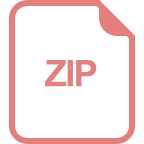
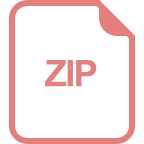
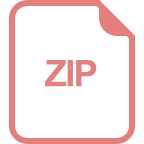
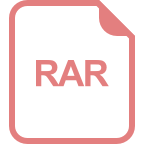
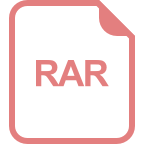
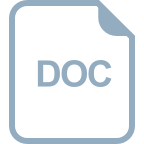
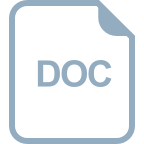
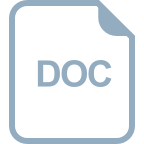
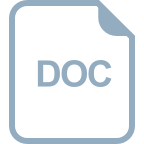
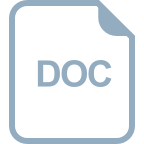
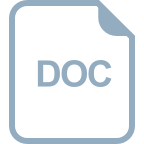