img = cv2.imread(filename) img = img[:,:,::-1] / 255.0 img = np.array(img).astype('float32') return img
时间: 2023-10-29 19:05:02 浏览: 68
这段代码是使用 OpenCV 库读取一张图片,并将像素值归一化到 0~1 范围内,最后将图片格式转换为 float32 类型的 numpy 数组。具体解释如下:
- cv2.imread(filename):使用 OpenCV 的 imread() 函数读取图片,其中 filename 表示图片路径和文件名。
- img[:,:,::-1]:将读取到的图片格式从 BGR 转换为 RGB,因为在 OpenCV 中默认读取的是 BGR 格式的图片。
- / 255.0:将像素值归一化到 0~1 范围内,因为在训练神经网络时,一般需要将输入数据归一化。
- np.array(img):将读取到的图片转换为 numpy 数组。
- .astype('float32'):将图片格式转换为 float32 类型的 numpy 数组,以便于后续进行计算和处理。
- return img:返回处理后的图片数组。
相关问题
将以下的python程序转化为c++版本,并在vs2022上实现。import cv2 import numpy as np from skimage.transform import radon import os thre1=10 thre2=-10 r=60 maxVal = 0 index = 0 sequence_path = "./images/" for file in os.listdir(sequence_path): filename=os.path.join(sequence_path, file) image=cv2.imread(filename, 0) image=cv2.blur(image,(3,3)) img=np.zeros((len(image), len(image[0])),np.uint8) maxVal = 0 index = 0 retval, labels, stats, centroids = cv2.connectedComponentsWithStats(img, connectivity=8) for i in range(1, len(stats)): if stats[i][4] > maxVal: maxVal = stats[i][4] index = i #x,y,h,w s for i in range(len(labels)): for j in range(len(labels[0])): if labels[i][j]==index: labels[i][j]=255 else: labels[i][j] = 0 cv2.imwrite('./4-max_region.jpg',labels) img2=cv2.imread('./4-max_region.jpg',0) img3=cv2.Canny(img2,15,200) # theta = np.linspace(0, 180, endpoint=False) img4 = radon(img3) max_angel=0 for i in range(len(img4)): for j in range(len(img4[0])): if img4[i][j]>max_angel: max_angel=img4[i][j] angel=j print("{}方向为:{} °".format(filename,angel))
在VS2022上实现这段Python代码的C++版本,你可以使用以下代码作为参考:
```cpp
#include <iostream>
#include <opencv2/opencv.hpp>
#include <opencv2/core.hpp>
#include <opencv2/imgproc.hpp>
#include <opencv2/highgui.hpp>
#include <opencv2/ml.hpp>
#include <opencv2/imgcodecs.hpp>
#include <vector>
#include <cmath>
#include <algorithm>
using namespace std;
using namespace cv;
int main() {
int thre1 = 10;
int thre2 = -10;
int r = 60;
int maxVal = 0;
int index = 0;
string sequence_path = "./images/";
for (const auto &entry : filesystem::directory_iterator(sequence_path)) {
string filename = entry.path().string();
Mat image = imread(filename, IMREAD_GRAYSCALE);
blur(image, image, Size(3,3));
Mat img(image.rows, image.cols, CV_8U, Scalar(0));
maxVal = 0;
index = 0;
Mat labels, stats, centroids;
int retval = connectedComponentsWithStats(img, labels, stats, centroids, 8);
for (int i = 1; i < stats.rows; i++) {
if (stats.at<int>(i, CC_STAT_AREA) > maxVal) {
maxVal = stats.at<int>(i, CC_STAT_AREA);
index = i;
}
}
for (int i = 0; i < labels.rows; i++) {
for (int j = 0; j < labels.cols; j++) {
if (labels.at<int>(i, j) == index)
labels.at<int>(i, j) = 255;
else
labels.at<int>(i, j) = 0;
}
}
imwrite("./4-max_region.jpg", labels);
Mat img2 = imread("./4-max_region.jpg", IMREAD_GRAYSCALE);
Mat img3;
Canny(img2, img3, 15, 200);
Mat img4;
radon(img3, img4);
int max_angel = 0;
int angel = 0;
for (int i = 0; i < img4.rows; i++) {
for (int j = 0; j < img4.cols; j++) {
if (img4.at<float>(i, j) > max_angel) {
max_angel = img4.at<float>(i, j);
angel = j;
}
}
}
cout << filename << " 方向为:" << angel << " °" << endl;
}
return 0;
}
```
请注意,你需要在项目中添加OpenCV库的链接。此外,代码中使用了C++17的filesystem库进行文件遍历,因此你需要在编译时启用C++17标准。
img = cv2.imread(fileName) print(fileName) showimg = img with torch.no_grad(): img = letterbox(img, new_shape=self.opt.img_size)[0] # Convert # BGR to RGB, to 3x416x416 img = img[:, :, ::-1].transpose(2, 0, 1) img = np.ascontiguousarray(img) img = torch.from_numpy(img).to(self.device) img = img.half() if self.half else img.float() # uint8 to fp16/32 img /= 255.0 # 0 - 255 to 0.0 - 1.0 if img.ndimension() == 3: img = img.unsqueeze(0) # Inference pred = self.model(img, augment=self.opt.augment)[0] # Apply NMS pred = non_max_suppression(pred, self.opt.conf_thres, self.opt.iou_thres, classes=self.opt.classes, agnostic=self.opt.agnostic_nms) print(pred) # Process detections for i, det in enumerate(pred): if det is not None and len(det): # Rescale boxes from img_size to im0 size det[:, :4] = scale_coords( img.shape[2:], det[:, :4], showimg.shape).round() for *xyxy, conf, cls in reversed(det): label = '%s %.2f' % (self.names[int(cls)], conf) name_list.append(self.names[int(cls)]) plot_one_box(xyxy, showimg, label=label, color=self.colors[int(cls)], line_thickness=2) cv2.imwrite('prediction.jpg', showimg) self.result = cv2.cvtColor(showimg, cv2.COLOR_BGR2BGRA) self.QtImg = QtGui.QImage( self.result.data, self.result.shape[1], self.result.shape[0], QtGui.QImage.Format_RGB32) self.label_4.setPixmap(QtGui.QPixmap.fromImage(self.QtImg))
这段代码主要是将文件加载并读入到img变量中,然后对图像进行缩放(使用letterbox函数)以适应所选的img_size。最后将变换后的图像存入img中。在PyTorch的上下文中,这些变换是不进行梯度计算的,因为没有必要对它们进行反向传播。
相关推荐
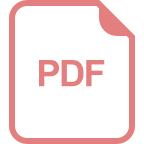
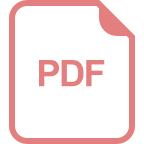
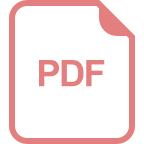












