pytorch实现将self-attention机制添加到mlp中
时间: 2023-08-31 13:41:51 浏览: 337
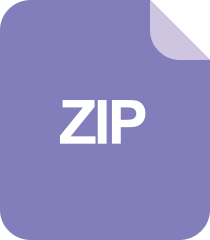
Self-Attention,深度学习意力机制,注意力模型,仔细分析了他们的设计方法和应用领域,pytorch实现
### 回答1:
要将self-attention机制添加到mlp中,您可以使用PyTorch中的torch.nn.MultiheadAttention模块。这个模块可以实现self-attention机制,并且可以直接用在多层感知机(mlp)中。
首先,您需要定义一个包含多个线性层和self-attention模块的PyTorch模型。然后,您可以将输入传递给多层感知机,并将多层感知机的输出作为self-attention模块的输入。最后,将self-attention模块的输出传递给后续的层进行处理,例如输出层。
以下是一个简单的示例代码,演示如何在PyTorch中将self-attention机制添加到mlp中:
```
import torch
import torch.nn as nn
class MLPWithSelfAttention(nn.Module):
def __init__(self, input_dim, hidden_dim, num_heads):
super(MLPWithSelfAttention, self).__init__()
# 定义多层感知机的线性层
self.fc1 = nn.Linear(input_dim, hidden_dim)
self.fc2 = nn.Linear(hidden_dim, hidden_dim)
# 定义self-attention模块
self.self_attn = nn.MultiheadAttention(hidden_dim, num_heads)
# 定义输出层
self.out = nn.Linear(hidden_dim, 1)
def forward(self, x):
# 通过多层感知机进行前向传递
x = self.fc1(x)
x = torch.relu(x)
x = self.fc2(x)
# 通过self-attention模块进行前向传递
x, _ = self.self_attn(x, x, x)
# 通过输出层进行前向传递
x = self.out(x)
return x
```
在这个例子中,MLPWithSelfAttention类定义了一个包含两个线性层、一个self-attention模块和一个输出层的多层感知机。在forward()方法中,输入首先通过两个线性层进行处理,然后将输出传递给self-attention模块进行处理。最后,self-attention模块的输出传递给输出层进行处理,并返回模型的输出。
### 回答2:
实现将self-attention机制添加到多层感知机(MLP)中需要使用PyTorch框架。Self-attention是一种在序列数据上运行的机制,它可以提取序列内元素之间的关系。以下是一个简单的示例代码,演示了如何将self-attention添加到一个具有两个隐藏层的MLP中:
首先,需要导入PyTorch库:
``` python
import torch
import torch.nn as nn
```
然后,定义一个自定义的MLP模型类,并在其中添加self-attention机制:
``` python
class MLPWithSelfAttention(nn.Module):
def __init__(self, input_dim, hidden_dim, output_dim):
super(MLPWithSelfAttention, self).__init__()
self.fc1 = nn.Linear(input_dim, hidden_dim)
self.fc2 = nn.Linear(hidden_dim, hidden_dim)
self.fc3 = nn.Linear(hidden_dim, output_dim)
self.self_attention = nn.MultiheadAttention(hidden_dim, num_heads=1)
def forward(self, x):
x = torch.relu(self.fc1(x))
x = torch.relu(self.fc2(x))
# 将隐层的输出作为query, key和value输入到self-attention中
attention_output, _ = self.self_attention(x, x, x)
x = torch.relu(attention_output)
x = self.fc3(x)
return x
```
在这个示例中,MLP模型通过三个全连接层进行前向传播,然后将隐层输出作为query、key和value输入到了self-attention中。在self-attention层之后,我们使用ReLU激活函数进行非线性处理,并最终通过全连接层输出结果。
这就是如何将self-attention机制添加到MLP中的示例代码,通过将MLP输出作为self-attention的输入,可以提取序列数据中元素之间的关系,并增强模型的表达能力。
### 回答3:
为了将self-attention机制添加到MLP中,我们可以使用PyTorch提供的功能和技巧。
首先,我们需要导入PyTorch和必要的模块。在导入阶段,我们需要引入`nn`,`MultiheadAttention`和`Sequential`等模块。
```python
import torch
import torch.nn as nn
from torch.nn import MultiheadAttention
from torch.nn import Sequential
```
然后,我们可以创建一个自定义的MLP模型,并在其中定义self-attention层。我们可以使用`Sequential`来定义MLP的结构,其中包含线性层和激活函数。
```python
class MLPWithSelfAttention(nn.Module):
def __init__(self, input_size, hidden_size, num_heads):
super(MLPWithSelfAttention, self).__init__()
self.attention = MultiheadAttention(hidden_size, num_heads)
self.mlp = Sequential(
nn.Linear(input_size, hidden_size),
nn.ReLU(),
nn.Linear(hidden_size, hidden_size),
nn.ReLU()
)
def forward(self, x):
attention_output, _ = self.attention(x, x, x)
mlp_output = self.mlp(attention_output)
return mlp_output
```
在上面的代码中,我们在MLP模型中添加了一个self-attention层,并将其命名为`attention`。然后,我们使用`Sequential`定义了MLP的结构,其中包含两个线性层和ReLU激活函数。以`attention_output`作为输入,将其输入到MLP中,得到最终的MLP输出`mlp_output`。注意,这里的self-attention输入和输出都使用相同的变量`x`。
最后,我们可以创建一个MLPWithSelfAttention的实例,并将它传递给训练环节。
```python
input_size = 100
hidden_size = 64
num_heads = 8
model = MLPWithSelfAttention(input_size, hidden_size, num_heads)
input_data = torch.randn(32, input_size)
output = model(input_data)
```
在这个例子中,我们创建了一个MLPWithSelfAttention实例,并传入输入数据,最后得到输出结果。这样,我们就成功地将self-attention机制添加到了MLP中。
阅读全文
相关推荐
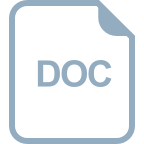
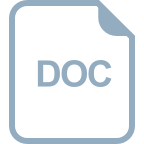





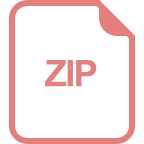
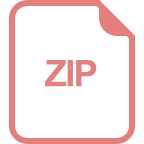
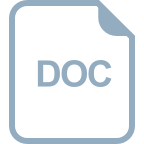
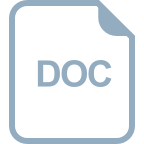
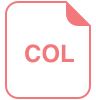





