将self attention加入到mlp的pytorch代码实现
时间: 2023-07-15 16:10:26 浏览: 165
以下是将 self-attention 加入到 MLP 中的 PyTorch 代码实现。假设输入的数据维度为 `input_size`,输出的维度为 `output_size`,self-attention 的隐藏层大小为 `hidden_size`。
```python
import torch.nn as nn
class SelfAttentionMLP(nn.Module):
def __init__(self, input_size, output_size, hidden_size):
super().__init__()
# 定义MLP的线性层
self.fc1 = nn.Linear(input_size, hidden_size)
self.fc2 = nn.Linear(hidden_size, output_size)
# 定义self-attention的线性层
self.attn_fc = nn.Linear(input_size, hidden_size)
def forward(self, x):
# MLP部分
x = nn.functional.relu(self.fc1(x))
x = self.fc2(x)
# self-attention部分
attn_weights = nn.functional.softmax(self.attn_fc(x), dim=-1)
x = torch.matmul(attn_weights.transpose(-1, -2), x)
return x
```
在上面的代码中,我们首先定义了一个 MLP,其中包含两个线性层。接下来,我们定义了一个与输入数据相同维度的线性层,用于计算 self-attention 的权重。然后,我们使用 softmax 计算这些权重,并使用矩阵乘法将它们与输入数据相乘以产生加权的输出。最后,我们返回 self-attention 的输出。
请注意,在此实现中,我们假设输入数据是一个二维张量(批次大小 x 输入大小)。如果您的输入张量具有更高的维度,则需要相应地更改代码。
阅读全文
相关推荐
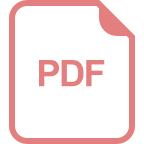
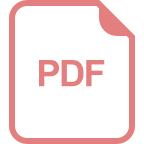
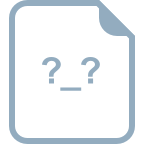




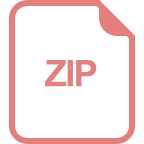
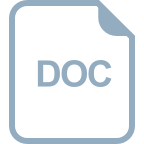
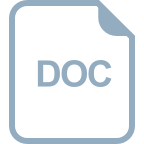
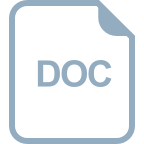
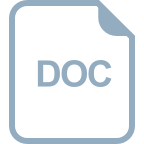
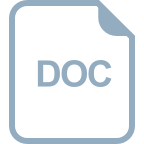






