将多头self attention加入到mlp的pytorch代码实现
时间: 2023-07-15 17:10:19 浏览: 101
以下是一个示例代码,展示了如何将多头self attention加入到mlp中。
```
import torch.nn as nn
import torch.nn.functional as F
class MultiHeadedAttention(nn.Module):
def __init__(self, input_dim, num_heads):
super(MultiHeadedAttention, self).__init__()
self.input_dim = input_dim
self.num_heads = num_heads
# query, key, value 的线性变换
self.query_linear = nn.Linear(input_dim, input_dim)
self.key_linear = nn.Linear(input_dim, input_dim)
self.value_linear = nn.Linear(input_dim, input_dim)
# 多头注意力的输出线性变换
self.output_linear = nn.Linear(input_dim, input_dim)
def forward(self, inputs):
batch_size = inputs.size(0)
# 线性变换
query = self.query_linear(inputs)
key = self.key_linear(inputs)
value = self.value_linear(inputs)
# 将输入向量拆分为多个头
query = query.view(batch_size * self.num_heads, -1, self.input_dim // self.num_heads)
key = key.view(batch_size * self.num_heads, -1, self.input_dim // self.num_heads)
value = value.view(batch_size * self.num_heads, -1, self.input_dim // self.num_heads)
# 计算注意力权重
attention_weights = torch.bmm(query, key.transpose(1, 2))
attention_weights = F.softmax(attention_weights, dim=2)
# 加权平均值
attention_output = torch.bmm(attention_weights, value)
# 合并多个头
attention_output = attention_output.view(batch_size, -1, self.input_dim)
# 输出线性变换
attention_output = self.output_linear(attention_output)
return attention_output
class MLP(nn.Module):
def __init__(self, input_dim, hidden_dim, output_dim, num_heads):
super(MLP, self).__init__()
self.input_dim = input_dim
self.hidden_dim = hidden_dim
self.output_dim = output_dim
self.num_heads = num_heads
# 输入层
self.input_layer = nn.Linear(input_dim, hidden_dim)
# 多头自注意力层
self.attention_layer = MultiHeadedAttention(hidden_dim, num_heads)
# 输出层
self.output_layer = nn.Linear(hidden_dim, output_dim)
def forward(self, inputs):
# 输入层
hidden = F.relu(self.input_layer(inputs))
# 多头自注意力层
attention_output = self.attention_layer(hidden)
# 输出层
output = self.output_layer(attention_output)
return output
```
这里定义了一个名为MultiHeadedAttention的自注意力层,它将输入向量拆分成多个头,并计算每个头的注意力权重,然后将这些头的加权平均值合并,最后输出经过线性变换的注意力输出。
然后,定义了一个名为MLP的多层感知机模型,它由一个输入层、一个多头自注意力层和一个输出层组成。在前向传递过程中,输入向量首先通过输入层,然后通过多头自注意力层,最后通过输出层。
在构建模型对象时,我们需要指定输入维度、隐藏层维度、输出维度和头的数量。例如,我们可以这样实例化一个MLP对象:
```
mlp = MLP(input_dim=100, hidden_dim=200, output_dim=10, num_heads=4)
```
这将创建一个输入维度为100、隐藏层维度为200、输出维度为10、头数为4的MLP模型。
阅读全文
相关推荐
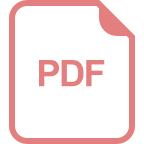
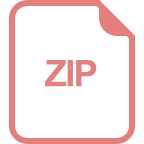
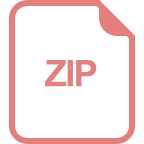















