AP聚类python算法
时间: 2023-10-11 12:08:48 浏览: 88
AP聚类(Affinity Propagation Clustering)是一种基于图论的聚类算法,可以自动决定数据集中的聚类数目。它不需要指定聚类的数量,而是通过样本自身的相似性来确定聚类的个数和每个样本所属的类别。
下面给出一个用 Python 实现 AP 聚类的例子:
```python
from sklearn.cluster import AffinityPropagation
from sklearn import metrics
from sklearn.datasets import make_blobs
# 生成样本数据
centers = [[1, 1], [-1, -1], [1, -1]]
X, labels_true = make_blobs(n_samples=300, centers=centers, cluster_std=0.5, random_state=0)
# 计算相似度矩阵
af = AffinityPropagation().fit(X)
cluster_centers_indices = af.cluster_centers_indices_
labels = af.labels_
n_clusters_ = len(cluster_centers_indices)
# 输出聚类结果
print('Estimated number of clusters: %d' % n_clusters_)
print("Homogeneity: %0.3f" % metrics.homogeneity_score(labels_true, labels))
print("Completeness: %0.3f" % metrics.completeness_score(labels_true, labels))
print("V-measure: %0.3f" % metrics.v_measure_score(labels_true, labels))
print("Adjusted Rand Index: %0.3f" % metrics.adjusted_rand_score(labels_true, labels))
print("Adjusted Mutual Information: %0.3f" % metrics.adjusted_mutual_info_score(labels_true, labels))
```
在上述代码中,我们使用了 `make_blobs()` 生成了一个三类数据集,然后使用 `AffinityPropagation()` 计算相似度矩阵并进行聚类,最后输出了聚类结果和聚类评估指标。
需要注意的是,AP 聚类算法的时间复杂度较高,因此对于大规模数据集可能不太适用。
阅读全文
相关推荐

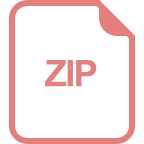
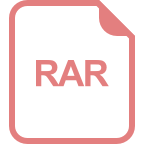
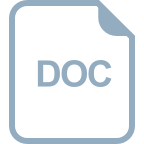


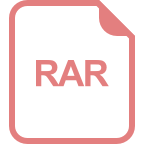
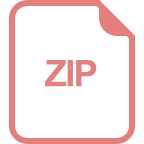
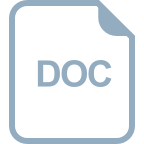
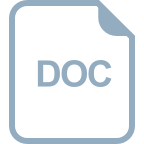



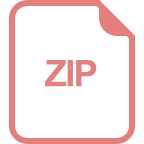
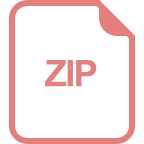