写出遗传算法算出一元二次函数的极值并输出对应的自变量,用matlab代码写
时间: 2024-10-05 17:04:49 浏览: 40
遗传算法是一种基于生物进化原理的优化算法,常用于解决复杂非线性问题,包括寻找函数的极值。对于一元二次函数 f(x) = ax^2 + bx + c 的最小化问题,我们可以构建一个简单的遗传算法模型。
下面是一个简化的MATLAB遗传算法求解一元二次函数极值的示例代码:
```Matlab
% 定义一元二次函数
function [fval, x] = quadratic_function(x, a, b)
fval = a * x.^2 + b * x;
end
% 初始化遗传算法参数
pop_size = 50; % 种群大小
num_generations = 100; % 进化代数
a = -1; % 随机系数范围下限
b = 4; % 随机系数范围上限
c = 0; % 常数项
x_bounds = [-10 10]; % 自变量范围
% 遗传操作函数
function offspring = crossover(parent1, parent2)
r = randi(2, 1);
offspring = [(1-r).*parent1 + r.*parent2];
end
function mutated_offspring = mutation(parent, mutation_rate)
r = rand(size(parent));
mutated_offspring = parent .* (r < mutation_rate) + parent .* (r >= mutation_rate);
end
% 主遗传算法循环
a_population = linspace(a, b, pop_size)'; % 初始种群
for gen = 1:num_generations
fitness = zeros(pop_size, 1); % 计算适应度
for i = 1:pop_size
x_guess = a_population(i);
[fitness(i), _] = quadratic_function(x_guess, a, b);
end
% 选择
parents = a_population(fitness == min(fitness));
% 变异与交叉
offspring = [];
while length(offspring) < pop_size
parent1 = parents(randi(numel(parents)));
parent2 = parents(randi(numelor(parents)));
offspring = [offspring crossover(parent1, parent2)];
mutated_offspring = mutation(offspring(end), 0.1); % 有一定概率变异
offspring = [offspring mutated_offspring];
end
% 更新种群
a_population = offspring;
end
% 找到最佳解
[min_fitness, best_x] = min(fitness);
[x_min, ~] = min(quadratic_function(a_population, a, b)', 2);
disp("最小值函数值: " + num2str(min_fitness));
disp("对应自变量: " + num2str(best_x));
```
这个代码首先定义了一元二次函数,并设置遗传算法的基本参数。然后通过迭代的方式,通过选择、交叉和变异生成新的种群,逐步接近函数的极小值。最后输出找到的最佳解及其对应的自变量。
阅读全文
相关推荐
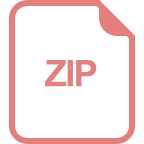
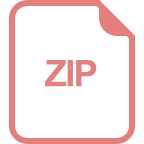
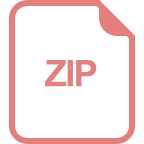















