def Stop_words(): stopword = [] data = [] f = open('C:/Users/Administrator/Desktop/data/stopword.txt',encoding='utf8') for line in f.readlines(): data.append(line) for i in data: output = str(i).replace('\n','')#replace用法和sub函数很接近 stopword.append(output) return stopword # 采用jieba进行词性标注,对当前文档过滤词性和停用词 def Filter_word(text): filter_word = [] stopword = Stop_words() text = jieba.posseg.cut(text) for word, flag in text: if flag.startswith('n') is False:#用于检测字符串是否以指定的子字符串开始 continue if not word in stopword and len(word) > 1: filter_word.append(word) return filter_word # 对文档集过滤词性和停用词 def Filter_words(data_path =r'C:/Users/Administrator/Desktop/data//corpus.txt'): document = [] for line in open(data_path, 'r',encoding= 'utf8') : segment = jieba.posseg.cut(line.strip()) filter_words = [] stopword = Stop_words() for word, flag in segment: if flag.startswith('n') is False: continue if not word in stopword and len(word) > 1: filter_words.append(word) document.append(filter_words) return document def tf_idf(): tf_dict = {} idf_dict = {} filter_word = Filter_word(text) for word in filter_word: if word not in tf_dict: tf_dict[word] = 1 else: tf_dict[word] += 1 for word in tf_dict: tf_dict[word] = tf_dict[word] / len(text) document = Filter_words() doc_total = len(document) for doc in document: for word in set(doc): if word not in idf_dict: idf_dict[word] = 1 else: idf_dict[word] += 1 for word in idf_dict: idf_dict[word] = math.log(doc_total / (idf_dict[word] + 1)) tf_idf_dict = {} for word in filter_word: if word not in idf_dict: idf_dict[word] = 0 tf_idf_dict[word] = tf_dict[word] * idf_dict[word] return tf_idf_dict tf_idf_dict = tf_idf() keyword = 6 print('TF-IDF模型结果:') for key, value in sorted(tf_idf_dict.items(), key=operator.itemgetter(1),reverse=True)[:keyword]: print(key, end=' ') print('\n')
时间: 2024-03-28 22:38:58 浏览: 204
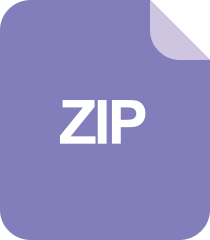
数据库项目设计 数据库项目设计包含类似的文档
这段代码实现了一个 TF-IDF 模型,用于计算文本中关键词的权重。其中,Stop_words 函数用于读取停用词表,Filter_word 函数用于对单个文档进行过滤,Filter_words 函数用于对整个文档集进行过滤。tf_idf 函数用于计算 TF-IDF 值,最后输出了权重最高的前 keyword 个关键词。
阅读全文
相关推荐
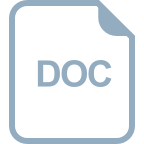
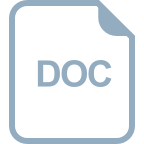















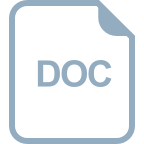