data=Testing_data(:,Label_training==i);
时间: 2024-05-18 07:12:18 浏览: 14
这段代码的作用是从Testing_data矩阵中选择标签为i的数据,并将其存储到变量data中。其中,Label_training是一个列向量,表示Testing_data中每个样本所属的类别标签,用==运算符可以得到一个逻辑向量,表示Label_training中哪些元素等于i。将逻辑向量作为下标,可以从Testing_data中选择对应的列,即标签为i的所有数据。最终得到的data矩阵的列数就是标签为i的数据的个数,行数与Testing_data保持一致。这个过程通常用于数据的子集选择和分割,便于后续的统计和机器学习任务。
相关问题
def jpeg_to_array (category_type, img_root_path,data_type): data_path = os.path.join(img_root_path,category_type.lower()) print('Loading ' + data_type +' images for category type: '+category_type) for img in os.listdir(data_path): img_path = os.path.join(data_path,img) if img_path.endswith('.jpg'): if(data_type == 'Training'): X_train.append(hog_data_extractor(img_path)) label_train.append(str(category_type)) if(data_type =='Testing'): X_test.append(hog_data_extractor(img_path)) label_test.append(np.array(str(category_type)))
这段代码实现了将JPEG格式的图像转换为数组的功能,并且将其用于机器学习中的训练和测试数据。其中,category_type表示分类类型,img_root_path表示图像所在文件夹的路径,data_type表示数据类型,可能是训练数据或测试数据。在函数中,通过os模块读取文件夹中的所有图像,并对图像进行处理,提取出HOG特征,并将其添加到对应的训练或测试数据集中。同时,还将图像的标签信息添加到对应的标签数据集中。最后,将训练和测试数据集和标签数据集返回。
import numpy as np import tensorflow as tf from tensorflow import keras import matplotlib.pyplot as plt ## Let us define a plt function for simplicity def plt_loss(x,training_metric,testing_metric,ax,colors = ['b']): ax.plot(x,training_metric,'b',label = 'Train') ax.plot(x,testing_metric,'k',label = 'Test') ax.set_xlabel('Epochs') ax.set_ylabel('Accuarcy')# ax.set_ylabel('Categorical Crossentropy Loss') plt.legend() plt.grid() plt.show() tf.keras.utils.set_random_seed(1) ## We import the Minist Dataset using Keras.datasets (train_data, train_labels), (test_data, test_labels) = keras.datasets.mnist.load_data() ## We first vectorize the image (28*28) into a vector (784) train_data = train_data.reshape(train_data.shape[0],train_data.shape[1]train_data.shape[2]) # 60000784 test_data = test_data.reshape(test_data.shape[0],test_data.shape[1]test_data.shape[2]) # 10000784 ## We next change label number to a 10 dimensional vector, e.g., 1->[0,1,0,0,0,0,0,0,0,0] train_labels = keras.utils.to_categorical(train_labels,10) test_labels = keras.utils.to_categorical(test_labels,10) ## start to build a MLP model N_batch_size = 5000 N_epochs = 100 lr = 0.01 ## we build a three layer model, 784 -> 64 -> 10 MLP_4 = keras.models.Sequential([ keras.layers.Dense(128, input_shape=(784,),activation='relu'), keras.layers.Dense(64,activation='relu'), keras.layers.Dense(10,activation='softmax') ]) MLP_4.compile( optimizer=keras.optimizers.Adam(lr), loss= 'categorical_crossentropy', metrics = ['accuracy'] ) History = MLP_4.fit(train_data[:10000],train_labels[:10000], batch_size = N_batch_size, epochs = N_epochs,validation_data=(test_data,test_labels), shuffle=False) train_acc = History.history['accuracy'] test_acc = History.history['val_accuracy']在该模型中加入early stopping,使用monitor='loss', patience = 2设置代码
Sure, here is the updated code with early stopping:
```
import numpy as np
import tensorflow as tf
from tensorflow import keras
import matplotlib.pyplot as plt
## Let us define a plt function for simplicity
def plt_loss(x,training_metric,testing_metric,ax,colors = ['b']):
ax.plot(x,training_metric,'b',label = 'Train')
ax.plot(x,testing_metric,'k',label = 'Test')
ax.set_xlabel('Epochs')
ax.set_ylabel('Accuarcy')# ax.set_ylabel('Categorical Crossentropy Loss')
plt.legend()
plt.grid()
plt.show()
tf.keras.utils.set_random_seed(1)
## We import the Minist Dataset using Keras.datasets
(train_data, train_labels), (test_data, test_labels) = keras.datasets.mnist.load_data()
## We first vectorize the image (28*28) into a vector (784)
train_data = train_data.reshape(train_data.shape[0],train_data.shape[1]*train_data.shape[2]) # 60000*784
test_data = test_data.reshape(test_data.shape[0],test_data.shape[1]*test_data.shape[2]) # 10000*784
## We next change label number to a 10 dimensional vector, e.g., 1->[0,1,0,0,0,0,0,0,0,0]
train_labels = keras.utils.to_categorical(train_labels,10)
test_labels = keras.utils.to_categorical(test_labels,10)
## start to build a MLP model
N_batch_size = 5000
N_epochs = 100
lr = 0.01
## we build a three layer model, 784 -> 64 -> 10
MLP_4 = keras.models.Sequential([
keras.layers.Dense(128, input_shape=(784,),activation='relu'),
keras.layers.Dense(64,activation='relu'),
keras.layers.Dense(10,activation='softmax')
])
MLP_4.compile(
optimizer=keras.optimizers.Adam(lr),
loss= 'categorical_crossentropy',
metrics = ['accuracy']
)
## Add early stopping
early_stop = tf.keras.callbacks.EarlyStopping(monitor='loss', patience=2)
History = MLP_4.fit(train_data[:10000],train_labels[:10000],
batch_size = N_batch_size,
epochs = N_epochs,
validation_data=(test_data,test_labels),
shuffle=False,
callbacks=[early_stop])
train_acc = History.history['accuracy']
test_acc = History.history['val_accuracy']
```
In this code, we added the early stopping callback by creating an instance of `tf.keras.callbacks.EarlyStopping` and passing it to the `callbacks` argument of the `fit` method. We set the `monitor` parameter to `'loss'` and the `patience` parameter to `2`. This means that training will stop if the loss does not improve after 2 epochs.
相关推荐













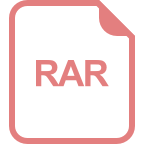