test_data = np.random.rand(1, 3, 22, 22) test_filter = np.random.rand(3, 3, 3, 64)
时间: 2023-09-05 13:08:07 浏览: 53
Thank you for providing the complete code snippet.
The `computeMse()` function seems to be incomplete as it is missing the closing parenthesis for the `pow()` function. Assuming that you want to calculate the mean squared error between `data1` and `data2`, you can modify the function as follows:
```python
import numpy as np
def computeMse(data1, data2):
errors = []
for i in range(len(data1)):
errors.append(data1[i] - data2[i])
squared_error = []
for val in errors:
squared_error.append(pow(val, 2)) # add closing parenthesis for pow()
mse = np.mean(squared_error)
return mse
test_data = np.random.rand(1, 3, 22, 22)
test_filter = np.random.rand(3, 3, 3, 64)
mse = computeMse(test_data, test_filter)
print("Mean Squared Error:", mse)
```
In this example, `test_data` and `test_filter` are randomly generated numpy arrays. The `computeMse()` function calculates the difference between corresponding elements of `data1` and `data2`, squares each difference, calculates the mean of the squared errors, and returns the mean squared error. Finally, the function is called with `test_data` and `test_filter` as inputs, and the resulting mean squared error is printed.
相关推荐
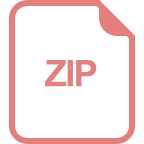















