vectors = np.stack((x.ravel(), y.ravel(), z.ravel()), axis=1) - np.stack((x.ravel(), y.ravel(), z.ravel()), axis=0) ValueError: operands could not be broadcast together with shapes (64,3) (3,64) 解决这段代码的问题
时间: 2023-11-03 19:04:17 浏览: 187
这个错误通常出现在进行数组运算时,两个数组的形状不兼容。具体来说,这里的错误信息显示,两个数组的形状分别是(64,3)和(3,64),无法进行广播计算。
要解决这个问题,需要将第二个数组的形状转置,使其与第一个数组的形状匹配。可以使用numpy库中的transpose函数或者T属性来实现。修改后的代码如下所示:
```
vectors = np.stack((x.ravel(), y.ravel(), z.ravel()), axis=1) - np.stack((x.ravel(), y.ravel(), z.ravel()), axis=0).T
```
这里,我们将第二个数组的形状转置之后,再进行减法运算,得到符合要求的向量数组。
相关问题
这段代码出现了这个问题: vectors = np.stack((x.ravel(), y.ravel(), z.ravel()), axis=1) - np.stack((x.ravel(), y.ravel(), z.ravel()), axis=0) ValueError: operands could not be broadcast together with shapes (64,3) (3,64) ,代码如下:import numpy as np # 定义坐标信息 points = np.array([[1, 2, 3], [4, 5, 6], [7, 8, 9], [10, 11, 12]]) # 生成所有点两两组合的向量 x, y, z = np.meshgrid(points[:, 0], points[:, 1], points[:, 2], indexing='ij') vectors = np.stack((x.ravel(), y.ravel(), z.ravel()), axis=1) - np.stack((x.ravel(), y.ravel(), z.ravel()), axis=0) # 判断每个向量是否平行于x-y面、x-z面或y-z面 parallel_xy = np.abs(vectors[:, 2]) < 1e-10 parallel_xz = np.abs(vectors[:, 1]) < 1e-10 parallel_yz = np.abs(vectors[:, 0]) < 1e-10 # 输出结果 print("平行于x-y面的向量:") print(vectors[parallel_xy]) print("平行于x-z面的向量:") print(vectors[parallel_xz]) print("平行于y-z面的向量:") print(vectors[parallel_yz]),帮我优化这段代码,解决运行的错误
这段代码的错误已经在之前的回答中解释过了,是由于两个数组的形状不兼容导致的。要解决这个问题,我们需要将第二个数组的形状转置,使其与第一个数组的形状匹配。可以使用numpy库中的transpose函数或者T属性来实现。
下面是修改后的代码:
```python
import numpy as np
# 定义坐标信息
points = np.array([[1, 2, 3], [4, 5, 6], [7, 8, 9], [10, 11, 12]])
# 生成所有点两两组合的向量
x, y, z = np.meshgrid(points[:, 0], points[:, 1], points[:, 2], indexing='ij')
vectors = np.stack((x.ravel(), y.ravel(), z.ravel()), axis=1) - np.stack((x.ravel(), y.ravel(), z.ravel()), axis=0).T
# 判断每个向量是否平行于x-y面、x-z面或y-z面
parallel_xy = np.abs(vectors[:, 2]) < 1e-10
parallel_xz = np.abs(vectors[:, 1]) < 1e-10
parallel_yz = np.abs(vectors[:, 0]) < 1e-10
# 输出结果
print("平行于x-y面的向量:")
print(vectors[parallel_xy])
print("平行于x-z面的向量:")
print(vectors[parallel_xz])
print("平行于y-z面的向量:")
print(vectors[parallel_yz])
```
在这个版本的代码中,我们将第二个数组的形状进行了转置,使其与第一个数组的形状匹配。这样就可以避免之前出现的形状不兼容的问题了。
import numpy as np import matplotlib.pyplot as plt # 设置模拟参数 num_boids = 50 # 粒子数 max_speed = 0.03 # 最大速度 max_force = 0.05 # 最大受力 neighborhood_radius = 0.2 # 邻域半径 separation_distance = 0.05 # 分离距离 alignment_distance = 0.1 # 对齐距离 cohesion_distance = 0.2 # 凝聚距离 # 初始化粒子位置和速度 positions = np.random.rand(num_boids, 2) velocities = np.random.rand(num_boids, 2) * max_speed # 模拟循环 for i in range(1000): # 计算邻域距离 distances = np.sqrt(np.sum(np.square(positions[:, np.newaxis, :] - positions), axis=-1)) neighbors = np.logical_and(distances > 0, distances < neighborhood_radius) # 计算三个力 separation = np.zeros_like(positions) alignment = np.zeros_like(positions) cohesion = np.zeros_like(positions) for j in range(num_boids): # 计算分离力 separation_vector = positions[j] - positions[neighbors[j]] separation_distance_mask = np.linalg.norm(separation_vector, axis=-1) < separation_distance separation_vector = separation_vector[separation_distance_mask] separation[j] = np.sum(separation_vector, axis=0) # 计算对齐力 alignment_vectors = velocities[neighbors[j]] alignment_distance_mask = np.linalg.norm(separation_vector, axis=-1) < alignment_distance alignment_vectors = alignment_vectors[alignment_distance_mask] alignment[j] = np.sum(alignment_vectors, axis=0) # 计算凝聚力 cohesion_vectors = positions[neighbors[j]] cohesion_distance_mask = np.linalg.norm(separation_vector, axis=-1) < cohesion_distance cohesion_vectors = cohesion_vectors[cohesion_distance_mask] cohesion[j] = np.sum(cohesion_vectors, axis=0) # 计算总受力 total_force = separation + alignment + cohesion total_force = np.clip(total_force, -max_force, max_force) # 更新速度和位置 velocities += total_force velocities = np.clip(velocities, -max_speed, max_speed) positions += velocities # 绘制粒子 plt.clf() plt.scatter(positions[:, 0], positions[:, 1], s=5) plt.xlim(0, 1) plt.ylim(0, 1) plt.pause(0.01)
这段代码是一个基于群体智能的仿真模型,用于模拟粒子的运动行为。该模型使用numpy和matplotlib库来实现。主要步骤包括:
1. 设置模拟参数:定义粒子数、最大速度、最大受力、邻域半径、分离距离、对齐距离、凝聚距离等参数。
2. 初始化粒子位置和速度:使用numpy的rand()函数生成随机位置和速度。
3. 模拟循环:在每个时间步长内,计算粒子的邻域距离,并根据邻域距离计算分离力、对齐力、凝聚力等三个力。最后,根据总受力更新粒子的速度和位置,并将粒子的位置绘制出来。
该模型可以用于研究粒子运动的行为和规律,也可以用于模拟群体智能算法的效果。
阅读全文
相关推荐
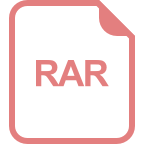
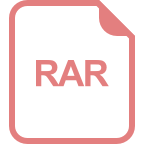













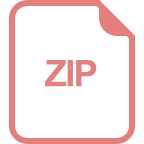