{net}-{epoch}-{type}.pth
时间: 2023-07-24 08:00:16 浏览: 53
`{net}-{epoch}-{type}.pth` 是一个文件命名模板,通常用于保存模型的参数文件。其中,"{net}"表示网络的名称或标识符,"{epoch}"表示当前保存的模型对应的训练轮数,"{type}"表示保存的模型类型或其他附加信息。
在实际应用中,这个命名模板可以根据具体需求进行修改。例如,可以将"{net}"替换为具体的网络结构名称,如"resnet"、"vgg"等;"{epoch}"可以替换为当前保存模型时的训练轮数或时间戳;"{type}"可以用于区分不同类型的模型参数文件,如"best"表示最佳模型、"latest"表示最新模型等。
通过使用这样的命名模板,可以方便地保存和管理多个模型的参数文件,并且可以根据文件名快速了解模型的相关信息。
相关问题
这段代码中加一个test loss功能 class LSTM(nn.Module): def __init__(self, input_size, hidden_size, num_layers, output_size, batch_size, device): super().__init__() self.device = device self.input_size = input_size self.hidden_size = hidden_size self.num_layers = num_layers self.output_size = output_size self.num_directions = 1 # 单向LSTM self.batch_size = batch_size self.lstm = nn.LSTM(self.input_size, self.hidden_size, self.num_layers, batch_first=True) self.linear = nn.Linear(65536, self.output_size) def forward(self, input_seq): h_0 = torch.randn(self.num_directions * self.num_layers, self.batch_size, self.hidden_size).to(self.device) c_0 = torch.randn(self.num_directions * self.num_layers, self.batch_size, self.hidden_size).to(self.device) output, _ = self.lstm(input_seq, (h_0, c_0)) pred = self.linear(output.contiguous().view(self.batch_size, -1)) return pred if __name__ == '__main__': # 加载已保存的模型参数 saved_model_path = '/content/drive/MyDrive/危急值/model/dangerous.pth' device = 'cuda:0' lstm_model = LSTM(input_size=1, hidden_size=64, num_layers=1, output_size=3, batch_size=256, device='cuda:0').to(device) state_dict = torch.load(saved_model_path) lstm_model.load_state_dict(state_dict) dataset = ECGDataset(X_train_df.to_numpy()) dataloader = DataLoader(dataset, batch_size=256, shuffle=True, num_workers=0, drop_last=True) loss_fn = nn.CrossEntropyLoss() optimizer = optim.SGD(lstm_model.parameters(), lr=1e-4) for epoch in range(200000): print(f'epoch:{epoch}') lstm_model.train() epoch_bar = tqdm(dataloader) for x, y in epoch_bar: optimizer.zero_grad() x_out = lstm_model(x.to(device).type(torch.cuda.FloatTensor)) loss = loss_fn(x_out, y.long().to(device)) loss.backward() epoch_bar.set_description(f'loss:{loss.item():.4f}') optimizer.step() if epoch % 100 == 0 or epoch == epoch - 1: torch.save(lstm_model.state_dict(), "/content/drive/MyDrive/危急值/model/dangerous.pth") print("权重成功保存一次")
以下是加入 Test Loss 功能后的代码:
```python
import torch
import torch.nn as nn
import torch.optim as optim
from torch.utils.data import DataLoader
class LSTM(nn.Module):
def __init__(self, input_size, hidden_size, num_layers, output_size, batch_size, device):
super().__init__()
self.device = device
self.input_size = input_size
self.hidden_size = hidden_size
self.num_layers = num_layers
self.output_size = output_size
self.num_directions = 1 # 单向LSTM
self.batch_size = batch_size
self.lstm = nn.LSTM(self.input_size, self.hidden_size, self.num_layers, batch_first=True)
self.linear = nn.Linear(65536, self.output_size)
def forward(self, input_seq):
h_0 = torch.randn(self.num_directions * self.num_layers, self.batch_size, self.hidden_size).to(self.device)
c_0 = torch.randn(self.num_directions * self.num_layers, self.batch_size, self.hidden_size).to(self.device)
output, _ = self.lstm(input_seq, (h_0, c_0))
pred = self.linear(output.contiguous().view(self.batch_size, -1))
return pred
if __name__ == '__main__':
# 加载已保存的模型参数
saved_model_path = '/content/drive/MyDrive/危急值/model/dangerous.pth'
device = 'cuda:0'
lstm_model = LSTM(input_size=1, hidden_size=64, num_layers=1, output_size=3, batch_size=256, device=device).to(device)
state_dict = torch.load(saved_model_path)
lstm_model.load_state_dict(state_dict)
# 加载测试数据集
test_dataset = ECGDataset(X_test_df.to_numpy())
test_loader = DataLoader(test_dataset, batch_size=256, shuffle=False, num_workers=0, drop_last=False)
# 定义损失函数和优化器
loss_fn = nn.CrossEntropyLoss()
optimizer = optim.SGD(lstm_model.parameters(), lr=1e-4)
# 开始训练
for epoch in range(200000):
print(f'epoch:{epoch}')
lstm_model.train()
epoch_bar = tqdm(dataloader)
for x, y in epoch_bar:
optimizer.zero_grad()
x_out = lstm_model(x.to(device).type(torch.cuda.FloatTensor))
loss = loss_fn(x_out, y.long().to(device))
loss.backward()
epoch_bar.set_description(f'loss:{loss.item():.4f}')
optimizer.step()
# 计算测试集上的平均损失
test_loss = 0.0
lstm_model.eval()
with torch.no_grad():
for x, y in test_loader:
x_out = lstm_model(x.to(device).type(torch.cuda.FloatTensor))
loss = loss_fn(x_out, y.long().to(device))
test_loss += loss.item() * x.size(0)
test_loss /= len(test_dataset)
print(f'Test Loss: {test_loss:.4f}')
if epoch % 100 == 0 or epoch == epoch - 1:
torch.save(lstm_model.state_dict(), "/content/drive/MyDrive/危急值/model/dangerous.pth")
print("权重成功保存一次")
```
在这个代码中,我们首先加载了测试数据集 `test_dataset` 和测试数据加载器 `test_loader`。在每个 epoch 完成后,我们计算测试集上的平均损失值。我们使用 `lstm_model.eval()` 将模型设为评估模式,并利用 `torch.no_grad()` 避免计算梯度,以加速计算。最后,我们输出测试集上的平均损失值。
import numpy as np import pandas as pd import matplotlib as plt from model import MLP import torch.optim as optim import torch import torch.nn as nn import argparse parser = argparse.ArgumentParser() parser.add_argument('--batchSize', type=int, default=4, help='input batch size') parser.add_argument('--nEpochs', type=int, default=100, help='number of epochs to train for') parser.add_argument('--LR', type=float, default=0.001, help='learning rate for net') opt = parser.parse_args() # 数据集预处理 df = pd.read_csv("C:/Users/shen/Desktop/housing.csv", delim_whitespace=True) # Turn into Numpy arrays arr = df.to_numpy(dtype='float') # Split the features and the labels X = arr[:, :-1] y = np.expand_dims(arr[:, -1], 1) ones = np.ones((X.shape[0], 1)) X_new = np.hstack((ones, X)) # Split the dataset into train set and test set indices = np.random.permutation(X_new.shape[0]) train_indices, test_indices = indices[:int(0.9*X_new.shape[0])], indices[int(0.9*X_new.shape[0]):] X_train, X_test = X_new[train_indices, :], X_new[test_indices, :] y_train, y_test = y[train_indices, :], y[test_indices, :] model = MLP() device = torch.device("cuda:0" if torch.cuda.is_available() else "cpu") model.to(device) model.train() optim_model = optim.Adam(model.parameters(), lr=opt.LR)#Adam优化器 # for epoch in range(opt.nEpochs): # # START CODE HERE # # END CODE HERE # torch.save(model.state_dict(),"C:/Users/shen/Desktop/checkpoint/net_weight.pth")帮我解释这里面每行代码的意思
这段代码主要是在导入一些Python的库(如numpy,pandas,matplotlib等),以及导入一个叫做MLP的模型。同时还使用了一些PyTorch的库(如optim,nn等)。接下来使用argparse库创建了一个命令行参数解析器,可以通过命令行来指定一些参数(比如batchSize,nEpochs和LR)。最后通过parse_args()函数解析这些参数。
相关推荐
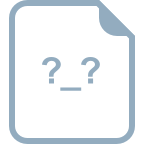
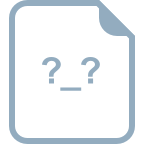












