tf.Variable
时间: 2023-10-08 13:11:22 浏览: 110
A tf.Variable is a type of TensorFlow object that represents a mutable tensor. It is used to store and update values during the computation of a TensorFlow graph. Variables are typically used to hold the trainable parameters of a machine learning model, such as the weights and biases of a neural network. They can be initialized with an initial value and updated using operations such as assign and assign_add. Variables have a specific shape and data type, which is specified when they are created. They also have a name, which can be used to identify them in the TensorFlow graph.
相关问题
class PhysicsInformedNN: # Initialize the class def __init__(self, x0, u0, x1, u1, layers, dt, lb, ub, q, splitIdx1, splitIdx2, splitIdx3, otherParams): self.lb = lb self.ub = ub self.x0 = x0 self.x1 = x1 self.u0 = u0 self.u1 = u1 self.layers = layers self.dt = dt self.q = max(q, 1) self.splitIdx1 = splitIdx1 self.splitIdx2 = splitIdx2 self.splitIdx3 = splitIdx3 # Initialize NN self.weights, self.biases = self.initialize_NN(layers) self.otherParams = otherParams self.L = tf.Variable([2], dtype=tf.float32) self.RL = tf.Variable([0.039], dtype=tf.float32) self.C = tf.Variable([0.412], dtype=tf.float32) self.RC = tf.Variable([1.59], dtype=tf.float32) self.Rdson = tf.Variable([1.22], dtype=tf.float32) self.Rload1 = tf.Variable([1.22], dtype=tf.float32) self.Rload2 = tf.Variable([1.22], dtype=tf.float32) self.Rload3 = tf.Variable([1.22], dtype=tf.float32) self.vIn = tf.Variable([0.87], dtype=tf.float32) self.vF = tf.Variable([0.1], dtype=tf.float32) tmp = np.float32(np.loadtxt( 'Butcher_tableau/Butcher_IRK%d.txt' % (q), ndmin=2)) weights = np.reshape(tmp[0:q ** 2 + q], (q + 1, q)) self.IRK_alpha = weights[0:-1, :] self.IRK_beta = weights[-1:, :] self.IRK_times = tmp[q ** 2 + q:]
这段代码是一个名为PhysicsInformedNN的类的初始化方法。它接受了一些参数,包括x0、u0、x1、u1、layers、dt、lb、ub、q、splitIdx1、splitIdx2、splitIdx3和otherParams。
在初始化方法中,它保存了lb和ub的值,这是表示上下界的变量。
它还保存了x0、u0、x1和u1的值,这些值表示输入和输出的初始和目标状态。
layers表示神经网络的层数。
dt表示时间步长。
q表示IRK方法的阶数。
splitIdx1、splitIdx2和splitIdx3是用于将输入分割成多个部分的索引。
initialize_NN是一个用于初始化神经网络权重和偏置的方法。
其他参数被保存在otherParams变量中。
还有一些变量L、RL、C、RC、Rdson、Rload1、Rload2、Rload3、vIn和vF,它们是用于计算的常量。
IRK_alpha、IRK_beta和IRK_times是根据给定的q值从文件加载的常数值。
tf.placeholder与tf.Variable
`tf.placeholder` 和 `tf.Variable` 都是 TensorFlow 中的重要概念,但在使用方式、作用和特点上有所不同。
`tf.placeholder` 是一个占位符,用于在 TensorFlow 的计算图中定义输入数据的位置。它在定义计算图的时候并不需要给定具体的数值,而是在计算图运行时,通过 `feed_dict` 参数传入具体的数值。它通常用于传入训练数据和标签等变量,例如:
```
x = tf.placeholder(tf.float32, shape=[None, 784])
y = tf.placeholder(tf.float32, shape=[None, 10])
```
在这个例子中,我们定义了两个 `tf.placeholder`,`x` 和 `y`,分别用于输入训练数据和标签。其中,`shape=[None, 784]` 表示输入数据的形状是一个二维张量,第一个维度可以是任意大小,第二个维度是 784。
相比之下,`tf.Variable` 则是用于在 TensorFlow 的计算图中定义需要优化的变量。它在定义时需要给定初始值,通常是一个随机数值或者全零的数组。在 TensorFlow 的计算过程中,`tf.Variable` 的值会不断地被优化,以使得算法达到更好的结果。例如:
```
W = tf.Variable(tf.zeros([784, 10]))
b = tf.Variable(tf.zeros([10]))
```
在这个例子中,我们定义了两个 `tf.Variable`,`W` 和 `b`,分别表示权重和偏置。它们的初始值都是全零的数组。
总的来说,`tf.placeholder` 用于传入数据,`tf.Variable` 用于定义需要优化的变量。它们在 TensorFlow 中都有着重要的作用。
阅读全文
相关推荐
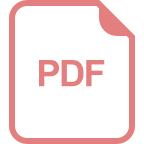
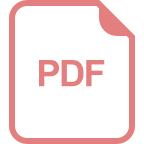











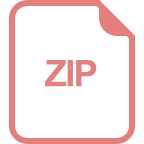
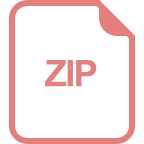
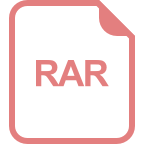