# 设置输入层节点数、隐层节点数 in_nodes=784 h1_nodes=100 h2_nodes=100 h3_nodes=50 # 定义输入、输出、prob的placeholder x=tf.placeholder(tf.float32,[None,in_nodes]) y_=tf.placeholder(tf.float32,[None,10]) prob=tf.placeholder(tf.float32) # 设置第一隐层 w1=weight(in_nodes, h1_nodes, 0.1, 0.005) b1=tf.Variable(tf.zeros([h1_nodes])) h1=tf.nn.relu(tf.matmul(x,w1)+b1) # 设置第二隐层 w2=weight(h1_nodes, h2_nodes, 0.1, 0.0) b2=tf.Variable(tf.zeros([h2_nodes])) h2=tf.nn.relu(tf.matmul(h1,w2)+b2) h2_drop=tf.nn.dropout(h2, prob) # 设置第三隐层 w3=weight(h2_nodes, h3_nodes, 0.1, 0.0) b3=tf.Variable(tf.zeros([h3_nodes])) h3=tf.nn.relu(tf.matmul(h2_drop,w3)+b3) h3_drop=tf.nn.dropout(h3, prob) # 设置softmax输出层 w4=weight(h3_nodes, 10, 0.1, 0.0) b4=tf.Variable(tf.zeros([10])) y=tf.nn.softmax(tf.matmul(h3_drop,w4)+b4)
时间: 2024-03-20 07:42:56 浏览: 116
将上述代码改写成 TensorFlow 2.x 版本的代码如下:
```python
import tensorflow as tf
def weight(shape, stddev, wd):
initial = tf.random.truncated_normal(shape, stddev=stddev)
var = tf.Variable(initial)
if wd is not None:
weight_decay = tf.multiply(tf.nn.l2_loss(var), wd, name='weight_loss')
tf.add_to_collection('losses', weight_decay)
return var
class MyModel(tf.keras.Model):
def __init__(self, in_nodes=784, h1_nodes=100, h2_nodes=100, h3_nodes=50):
super(MyModel, self).__init__()
self.w1 = weight([in_nodes, h1_nodes], 0.1, 0.005)
self.b1 = tf.Variable(tf.zeros([h1_nodes]))
self.w2 = weight([h1_nodes, h2_nodes], 0.1, 0.0)
self.b2 = tf.Variable(tf.zeros([h2_nodes]))
self.w3 = weight([h2_nodes, h3_nodes], 0.1, 0.0)
self.b3 = tf.Variable(tf.zeros([h3_nodes]))
self.w4 = weight([h3_nodes, 10], 0.1, 0.0)
self.b4 = tf.Variable(tf.zeros([10]))
def call(self, inputs, prob):
x = inputs
y_ = tf.cast(inputs, tf.float32)
h1 = tf.nn.relu(tf.matmul(x, self.w1) + self.b1)
h2 = tf.nn.relu(tf.matmul(h1, self.w2) + self.b2)
h2_drop = tf.nn.dropout(h2, rate=prob)
h3 = tf.nn.relu(tf.matmul(h2_drop, self.w3) + self.b3)
h3_drop = tf.nn.dropout(h3, rate=prob)
y = tf.nn.softmax(tf.matmul(h3_drop, self.w4) + self.b4)
return y
model = MyModel()
x = tf.keras.Input(shape=(None, 784))
prob = tf.keras.Input(shape=())
y = model(x, prob)
y_ = tf.keras.Input(shape=(None, 10))
# 定义损失函数
cross_entropy = tf.reduce_mean(-tf.reduce_sum(y_ * tf.math.log(y), reduction_indices=[1]))
tf.add_to_collection('losses', cross_entropy)
loss = tf.add_n(tf.get_collection('losses'))
# 定义优化器
train_step = tf.train.AdamOptimizer(1e-4).minimize(loss)
# 训练模型
with tf.Session() as sess:
sess.run(tf.global_variables_initializer())
for i in range(1000):
batch_xs, batch_ys = mnist.train.next_batch(100)
sess.run(train_step, feed_dict={x: batch_xs, y_: batch_ys, prob: 0.5})
```
在 TensorFlow 2.x 中,可以使用 `tf.reduce_mean` 和 `tf.reduce_sum` 函数来计算张量的平均值和总和;使用 `tf.math.log` 函数来计算张量的自然对数。此外,可以使用 `tf.train.AdamOptimizer` 来定义优化器,使用 `model.trainable_variables` 来获取所有可训练的变量。
阅读全文
相关推荐
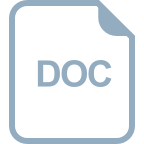
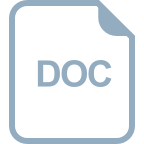
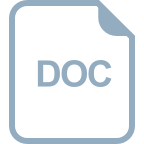















