img = imread('4.png'); [M , N] = size(img); img_result = zeros(M, N); muban_size = 3; expand_size = floor(muban_size / 2) muban = 1 / (muban_size * muban_size) .* ones(muban_size, muban_size); expand_img = double(wextend('2D','zpd', img, expand_size)); for i=1:M for j=1:N ave = sum( sum( expand_img(i:i+muban_size-1,j:j+muban_size-1) .* muban)); img_result(i,j) = ave; end end img_result = uint8(img_result); subplot(1 ,2, 1); title('原图像') imshow(img) subplot(1 ,2, 2); imshow(img_result) da = ['模板大小为' num2str(muban_size) ',变化后的图像']; title(da)
时间: 2024-04-04 21:29:12 浏览: 14
这段代码是用MATLAB实现的图像均值滤波。首先用imread函数读取名为“4.png”的图像,并获取其大小。然后定义一个模板大小muban_size,这里为3,再计算出模板的扩展大小expand_size(向下取整)。接着定义一个大小为muban_size × muban_size的模板muban,其每个元素的值都为1 / (muban_size × muban_size)。然后用wextend函数将图像进行扩展,将边界进行填充,使得可以进行卷积。接下来用两个for循环遍历原图像的每一个像素,并计算其周围muban_size × muban_size个像素的均值。最后将得到的结果存入img_result中,并将其显示出来。最后的标题显示了模板大小和变化后的图像。
相关问题
import cv2 import numpy as np # 读取图像 img = cv2.imread('hd.png') # 转换为灰度图像 gray = cv2.cvtColor(img, cv2.COLOR_BGR2GRAY) 修改这段代码将 最大轮廓的白的像素点如何将白的像素点变成黑的并保证其他区域不变py代码# 二值化处理 ret, thresh = cv2.threshold(gray, 127, 255, cv2.THRESH_BINARY) # 查找轮廓 _,contours, hierarchy = cv2.findContours(thresh, cv2.RETR_EXTERNAL, cv2.CHAIN_APPROX_SIMPLE) # 找到最大轮廓 max_contour = max(contours, key=cv2.contourArea) # 创建掩码 mask = np.zeros_like(gray) cv2.drawContours(mask, [max_contour], 0, 255, -1) # 应用掩码 result = np.zeros_like(img) result[mask == 255] = img[mask == 255] # 显示结果 cv2.imshow('Result', result) cv2.imwrite('zuida.jpg', result) cv2.waitKey(0) cv2.destroyAllWindows()
假设这个图像是一个二值化的黑白图像,黑色像素点值为0,白色像素点值为255。可以使用以下代码将白色像素点变成黑色像素点:
```python
import cv2
import numpy as np
# 读取图像并转换为灰度图像
img = cv2.imread('hd.png')
gray = cv2.cvtColor(img, cv2.COLOR_BGR2GRAY)
# 二值化处理
ret, thresh = cv2.threshold(gray, 127, 255, cv2.THRESH_BINARY)
# 查找轮廓
_, contours, hierarchy = cv2.findContours(thresh, cv2.RETR_EXTERNAL, cv2.CHAIN_APPROX_SIMPLE)
# 找到最大轮廓
max_contour = max(contours, key=cv2.contourArea)
# 创建掩码
mask = np.zeros_like(gray)
cv2.drawContours(mask, [max_contour], 0, 255, -1)
# 将白色像素点变成黑色像素点
result = gray.copy()
result[mask == 255] = 0
# 显示结果
cv2.imshow('Result', result)
cv2.imwrite('zuida.jpg', result)
cv2.waitKey(0)
cv2.destroyAllWindows()
```
这段代码的作用是找到图像中最大的轮廓,然后创建一个掩膜,在掩膜上绘制最大的轮廓,最后将白色像素点变成黑色像素点。其他区域不变。
import cv2 import numpy as np import os # 提取图像的HOG特征 def get_hog_features(image): hog = cv2.HOGDescriptor() hog_features = hog.compute(image) return hog_features # 加载训练数据集 train_data = [r"I:\18Breakageratecalculation\SVM run\detection_cut\whole\train128"] train_labels = [r"I:\18Breakageratecalculation\SVM run\detection_cut\whole\train128\labels.txt"] num_samples = 681 for i in range(num_samples): img = cv2.imread(str(i).zfill(3)+'.jpg') hog_features = get_hog_features(image) hsv_image = cv2.cvtColor(image, cv2.COLOR_BGR2HSV) color_hist = cv2.calcHist([hsv_image], [0, 1], None, [180, 256], [0, 180, 0, 256]) color_features = cv2.normalize(color_hist, color_hist).flatten() train_data.append(hog_features) train_labels.append(labels[i]) # 训练SVM模型 svm = cv2.ml.SVM_create() svm.setType(cv2.ml.SVM_C_SVC) svm.setKernel(cv2.ml.SVM_LINEAR) svm.train(np.array(train_data), cv2.ml.ROW_SAMPLE, np.array(train_labels)) # 对测试图像进行分类 test_image = cv2.imread('I:\18Breakageratecalculation\mask-slic use\maskSLIC-master\result\split\result2\maskslic2_roi.png', 0) test_features = get_hog_features(test_image) result = svm.predict(test_features.reshape(1,-1)) # 显示分割结果 result_image = np.zeros(test_image.shape, np.uint8) for i in range(test_image.shape[0]): for j in range(test_image.shape[1]): if result[i,j] == 1: result_image[i,j] = 255 cv2.imshow('I:\18Breakageratecalculation\mask-slic use\maskSLIC-master\result\split\result2\Result.png', result_image) cv2.waitKey(0) cv2.destroyAllWindows()
这段代码似乎存在一些问题。以下是一些可能需要修改的地方:
1. 在加载训练数据时,`train_data`和`train_labels`应该是列表类型,而不是字符串类型。您可以使用`os.listdir()`函数来获取文件夹中的所有图像文件名,并使用`cv2.imread()`函数读取图像文件。
2. 在获取HOG特征时,您应该使用`img`而不是`image`变量。
3. 在提取颜色直方图特征时,您应该使用`test_image`而不是`image`变量。
4. 在将特征添加到`train_data`列表中时,您应该将`hog_features`和`color_features`链接在一起。
5. 在使用`svm.predict()`函数对测试图像进行分类时,您应该使用`svm.predict(test_features)`而不是`svm.predict(test_features.reshape(1,-1))`。
6. 在显示分割结果时,您应该使用`cv2.imshow()`函数的第一个参数是窗口的名称,而不是图像文件的路径。
以下是修改后的示例代码:
```python
import cv2
import numpy as np
import os
# 提取图像的HOG特征
def get_hog_features(img):
hog = cv2.HOGDescriptor()
hog_features = hog.compute(img)
return hog_features.flatten()
# 加载训练数据集
train_folder = r"I:\18Breakageratecalculation\SVM run\detection_cut\whole\train128"
train_data = []
train_labels = []
for filename in os.listdir(train_folder):
if filename.endswith('.jpg'):
img = cv2.imread(os.path.join(train_folder, filename), 0)
hog_features = get_hog_features(img)
hsv_image = cv2.cvtColor(img, cv2.COLOR_BGR2HSV)
color_hist = cv2.calcHist([hsv_image], [0, 1], None, [180, 256], [0, 180, 0, 256])
color_features = cv2.normalize(color_hist, color_hist).flatten()
features = np.concatenate((hog_features, color_features))
train_data.append(features)
with open(os.path.join(train_folder, 'labels.txt'), 'r') as f:
label = int(f.readline().strip())
train_labels.append(label)
# 训练SVM模型
svm = cv2.ml.SVM_create()
svm.setType(cv2.ml.SVM_C_SVC)
svm.setKernel(cv2.ml.SVM_LINEAR)
svm.train(np.array(train_data), cv2.ml.ROW_SAMPLE, np.array(train_labels))
# 对测试图像进行分类
test_image = cv2.imread('I:\18Breakageratecalculation\mask-slic use\maskSLIC-master\result\split\result2\maskslic2_roi.png', 0)
test_features = get_hog_features(test_image)
hsv_test_image = cv2.cvtColor(test_image, cv2.COLOR_BGR2HSV)
color_hist = cv2.calcHist([hsv_test_image], [0, 1], None, [180, 256], [0, 180, 0, 256])
color_features = cv2.normalize(color_hist, color_hist).flatten()
test_features = np.concatenate((test_features, color_features))
result = svm.predict(test_features)
# 显示分割结果
result_image = np.zeros(test_image.shape, np.uint8)
for i in range(test_image.shape[0]):
for j in range(test_image.shape[1]):
if result[i,j] == 1:
result_image[i,j] = 255
cv2.imshow('Result', result_image)
cv2.waitKey(0)
cv2.destroyAllWindows()
```
请注意,以上代码仅作为参考。您可能需要根据自己的情况进行微调。
相关推荐
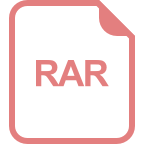
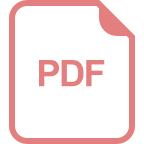
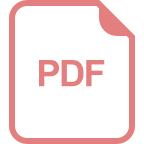












