R中:Standardize the matrix A such that columns of A are centered to have mean 0 and scaled to have standard deviation 1.
时间: 2024-02-04 16:03:40 浏览: 121
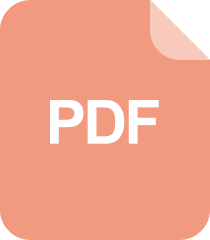
中心化和规范化1
可以使用R语言中的scale()函数来标准化矩阵A。代码如下:
```
scaled_A <- scale(A, center = TRUE, scale = TRUE)
```
其中,center = TRUE表示要对每列进行中心化处理,即让每列的均值为0。scale = TRUE表示要对每列进行标准化处理,即让每列的标准差为1。处理后的结果保存在scaled_A中。
阅读全文
相关推荐
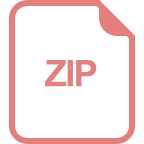
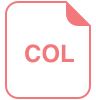
















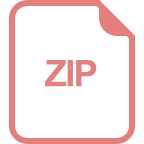