基于 cnn 的疲劳检测源码-python
时间: 2023-06-06 22:02:33 浏览: 120
基于CNN的疲劳检测源码是基于人工神经网络的算法实现,用于检测人体疲劳情况。这一技术对于需要大量人工劳动的生产环境和汽车驾驶等行业,具有重要的应用价值。
在Python中,可以通过使用tensorflow等深度学习框架,实现基于CNN的疲劳检测。具体步骤如下:
1. 数据预处理:将预测的图像数据集划分为训练集和测试集,进行数据清洗和标准化处理。
2. 搭建CNN模型:通过tensorflow或其它框架来构建深度神经网络模型。包括卷积、池化、 dropout、全连接层等,还可以使用一些优化算法,如 Adam优化器。
3. 模型训练:训练CNN模型,并使用测试数据集来验证模型的效果。可以通过改变网络的参数来提高模型的准确性。
4. 疲劳检测:通过模型的预测结果,分析人体疲劳情况,判断其是否需要休息或进行调整。
总的来说,基于CNN的疲劳检测源码Python实现,需要熟练掌握深度学习和神经网络相关知识,还需要对Python编程语言有充分的掌握。只有掌握了这些技能,才能顺利实现这一重要技术,为企业的发展提供新的动力。
相关问题
python:基于cnn的人脸检测项目源码
Python是一门强大的编程语言,广泛应用于各类人工智能和数据科学项目中。其中基于CNN的人脸检测项目源码,是Python领域内的一个热门研究领域。
该人脸检测项目源码基于卷积神经网络(CNN)技术,可以准确地检测并识别图像中的人脸。具体实现流程包括以下几步:
第一步是数据预处理。从大量人脸图像中选取出合适的训练样本,对原始图像进行尺寸调整、灰度化、归一化等处理,提高识别准确率。
第二步是CNN模型训练。采用卷积神经网络的深度学习算法,并使用已有的训练集进行训练。通过不断调整网络参数,优化模型结构和训练过程,提高检测准确率。
第三步是人脸检测。测试阶段,利用训练好的CNN模型,对输入的新图像进行检测。通过卷积计算和池化操作,对图像中的特征进行提取和匹配,最终确定人脸的位置和大小。
通过以上实现步骤,基于CNN的人脸检测项目可以实现高效、可靠的人脸检测功能。此外,Python作为一门简单易学、开发效率高的编程语言,非常适合进行此类人工智能项目的开发。
基于cnn-lstm的轴承故障诊断python源码
很抱歉,根据提供的引用内容,我没有找到基于cnn-lstm的轴承故障诊断的Python源码。但是,我可以为您提供基于Tensorflow+CNN深度学习的轴承故障诊断的Python源码,您可以参考该代码进行学习和实现。
以下是基于Tensorflow+CNN深度学习的轴承故障诊断的Python源码:
```python
import tensorflow as tf
import numpy as np
import os
import time
import datetime
from tensorflow.contrib import learn
import csv
# Parameters
# ==================================================
# Data loading params
tf.flags.DEFINE_float("dev_sample_percentage", .2, "Percentage of the training data to use for validation")
tf.flags.DEFINE_string("positive_data_file", "./data/positive.csv", "Data source for the positive data.")
tf.flags.DEFINE_string("negative_data_file", "./data/negative.csv", "Data source for the negative data.")
# Model Hyperparameters
tf.flags.DEFINE_integer("embedding_dim", 128, "Dimensionality of character embedding (default: 128)")
tf.flags.DEFINE_string("filter_sizes", "3,4,5", "Comma-separated filter sizes (default: '3,4,5')")
tf.flags.DEFINE_integer("num_filters", 128, "Number of filters per filter size (default: 128)")
tf.flags.DEFINE_float("dropout_keep_prob", 0.5, "Dropout keep probability (default: 0.5)")
tf.flags.DEFINE_float("l2_reg_lambda", 0.0, "L2 regularization lambda (default: 0.0)")
# Training parameters
tf.flags.DEFINE_integer("batch_size", 64, "Batch Size (default: 64)")
tf.flags.DEFINE_integer("num_epochs", 200, "Number of training epochs (default: 200)")
tf.flags.DEFINE_integer("evaluate_every", 100, "Evaluate model on dev set after this many steps (default: 100)")
tf.flags.DEFINE_integer("checkpoint_every", 100, "Save model after this many steps (default: 100)")
tf.flags.DEFINE_integer("num_checkpoints", 5, "Number of checkpoints to store (default: 5)")
tf.flags.DEFINE_boolean("allow_soft_placement", True, "Allow device soft device placement")
tf.flags.DEFINE_boolean("log_device_placement", False, "Log placement of ops on devices")
FLAGS = tf.flags.FLAGS
FLAGS._parse_flags()
print("\nParameters:")
for attr, value in sorted(FLAGS.__flags.items()):
print("{}={}".format(attr.upper(), value))
print("")
# Data Preparation
# ==================================================
# Load data
print("Loading data...")
x_text, y = data_helpers.load_data_and_labels(FLAGS.positive_data_file, FLAGS.negative_data_file)
# Build vocabulary
max_document_length = max([len(x.split(" ")) for x in x_text])
vocab_processor = learn.preprocessing.VocabularyProcessor(max_document_length)
x = np.array(list(vocab_processor.fit_transform(x_text)))
# Randomly shuffle data
np.random.seed(10)
shuffle_indices = np.random.permutation(np.arange(len(y)))
x_shuffled = x[shuffle_indices]
y_shuffled = y[shuffle_indices]
# Split train/test set
# TODO: This is very crude, should use cross-validation
dev_sample_index = -1 * int(FLAGS.dev_sample_percentage * float(len(y)))
x_train, x_dev = x_shuffled[:dev_sample_index], x_shuffled[dev_sample_index:]
y_train, y_dev = y_shuffled[:dev_sample_index], y_shuffled[dev_sample_index:]
print("Vocabulary Size: {:d}".format(len(vocab_processor.vocabulary_)))
print("Train/Dev split: {:d}/{:d}".format(len(y_train), len(y_dev)))
# Training
# ==================================================
with tf.Graph().as_default():
session_conf = tf.ConfigProto(
allow_soft_placement=FLAGS.allow_soft_placement,
log_device_placement=FLAGS.log_device_placement)
sess = tf.Session(config=session_conf)
with sess.as_default():
cnn = TextCNN(
sequence_length=x_train.shape[1],
num_classes=y_train.shape[1],
vocab_size=len(vocab_processor.vocabulary_),
embedding_size=FLAGS.embedding_dim,
filter_sizes=list(map(int, FLAGS.filter_sizes.split(","))),
num_filters=FLAGS.num_filters,
l2_reg_lambda=FLAGS.l2_reg_lambda)
# Define Training procedure
global_step = tf.Variable(0, name="global_step", trainable=False)
optimizer = tf.train.AdamOptimizer(1e-3)
grads_and_vars = optimizer.compute_gradients(cnn.loss)
train_op = optimizer.apply_gradients(grads_and_vars, global_step=global_step)
# Keep track of gradient values and sparsity (optional)
grad_summaries = []
for g, v in grads_and_vars:
if g is not None:
grad_hist_summary = tf.summary.histogram("{}/grad/hist".format(v.name), g)
sparsity_summary = tf.summary.scalar("{}/grad/sparsity".format(v.name), tf.nn.zero_fraction(g))
grad_summaries.append(grad_hist_summary)
grad_summaries.append(sparsity_summary)
grad_summaries_merged = tf.summary.merge(grad_summaries)
# Output directory for models and summaries
timestamp = str(int(time.time()))
out_dir = os.path.abspath(os.path.join(os.path.curdir, "runs", timestamp))
print("Writing to {}\n".format(out_dir))
# Summaries for loss and accuracy
loss_summary = tf.summary.scalar("loss", cnn.loss)
acc_summary = tf.summary.scalar("accuracy", cnn.accuracy)
# Train Summaries
train_summary_op = tf.summary.merge([loss_summary, acc_summary, grad_summaries_merged])
train_summary_dir = os.path.join(out_dir, "summaries", "train")
train_summary_writer = tf.summary.FileWriter(train_summary_dir, sess.graph)
# Dev summaries
dev_summary_op = tf.summary.merge([loss_summary, acc_summary])
dev_summary_dir = os.path.join(out_dir, "summaries", "dev")
dev_summary_writer = tf.summary.FileWriter(dev_summary_dir, sess.graph)
# Checkpoint directory. Tensorflow assumes this directory already exists so we need to create it
checkpoint_dir = os.path.abspath(os.path.join(out_dir, "checkpoints"))
checkpoint_prefix = os.path.join(checkpoint_dir, "model")
if not os.path.exists(checkpoint_dir):
os.makedirs(checkpoint_dir)
saver = tf.train.Saver(tf.global_variables(), max_to_keep=FLAGS.num_checkpoints)
# Write vocabulary
vocab_processor.save(os.path.join(out_dir, "vocab"))
# Initialize all variables
sess.run(tf.global_variables_initializer())
def train_step(x_batch, y_batch):
"""
A single training step
"""
feed_dict = {
cnn.input_x: x_batch,
cnn.input_y: y_batch,
cnn.dropout_keep_prob: FLAGS.dropout_keep_prob
}
_, step, summaries, loss, accuracy = sess.run(
[train_op, global_step, train_summary_op, cnn.loss, cnn.accuracy],
feed_dict)
time_str = datetime.datetime.now().isoformat()
print("{}: step {}, loss {:g}, acc {:g}".format(time_str, step, loss, accuracy))
train_summary_writer.add_summary(summaries, step)
def dev_step(x_batch, y_batch, writer=None):
"""
Evaluates model on a dev set
"""
feed_dict = {
cnn.input_x: x_batch,
cnn.input_y: y_batch,
cnn.dropout_keep_prob: 1.0
}
step, summaries, loss, accuracy = sess.run(
[global_step, dev_summary_op, cnn.loss, cnn.accuracy],
feed_dict)
time_str = datetime.datetime.now().isoformat()
print("{}: step {}, loss {:g}, acc {:g}".format(time_str, step, loss, accuracy))
if writer:
writer.add_summary(summaries, step)
# Generate batches
batches = data_helpers.batch_iter(
list(zip(x_train, y_train)), FLAGS.batch_size, FLAGS.num_epochs)
# Training loop. For each batch...
for batch in batches:
x_batch, y_batch = zip(*batch)
train_step(x_batch, y_batch)
current_step = tf.train.global_step(sess, global_step)
if current_step % FLAGS.evaluate_every == 0:
print("\nEvaluation:")
dev_step(x_dev, y_dev, writer=dev_summary_writer)
print("")
if current_step % FLAGS.checkpoint_every == 0:
path = saver.save(sess, checkpoint_prefix, global_step=current_step)
print("Saved model checkpoint to {}\n".format(path))
--相关问题--:
相关推荐
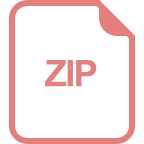












