def estimate_variance(xs: np.ndarray, ys: np.ndarray, affine: np.ndarray, translation: np.ndarray, responsibility: np.ndarray) -> float: """ Estimate the variance of GMM. For simplification, we assume all the Gaussian distributions share the same variance, and each feature dimension is independent, so the variance can be represented as a scalar. :param xs: a set of points with size (N, D), N is the number of samples, D is the dimension of points :param ys: a set of points with size (M, D), M is the number of samples, D is the dimension of points :param affine: an affine matrix with size (D, D) :param translation: a translation vector with size (1, D) :param responsibility: the responsibility matrix with size (N, M) :return: the variance of each Gaussian distribution, a float """ # TODO: change the code below and compute the variance of each Gaussian return 1
时间: 2024-01-25 17:02:48 浏览: 21
To compute the variance of each Gaussian distribution, we can use the following steps:
1. Transform the xs using the affine matrix and translation vector:
```
xs_transformed = xs.dot(affine) + translation
```
2. Compute the pairwise distance matrix between xs_transformed and ys:
```
distance_matrix = np.linalg.norm(xs_transformed[:, np.newaxis, :] - ys[np.newaxis, :, :], axis=2)
```
3. Compute the weighted sum of squared distances for each Gaussian:
```
weighted_distances = distance_matrix**2 * responsibility
sum_weighted_distances = np.sum(weighted_distances, axis=(0, 1))
```
4. Compute the total weight of all the points:
```
total_weight = np.sum(responsibility)
```
5. Compute the variance as the weighted average of the squared distances:
```
variance = sum_weighted_distances / total_weight
```
Here's the modified code:
```
def estimate_variance(xs: np.ndarray, ys: np.ndarray, affine: np.ndarray,
translation: np.ndarray, responsibility: np.ndarray) -> float:
"""
Estimate the variance of GMM.
For simplification, we assume all the Gaussian distributions share the same variance,
and each feature dimension is independent, so the variance can be represented as a scalar.
:param xs: a set of points with size (N, D), N is the number of samples, D is the dimension of points
:param ys: a set of points with size (M, D), M is the number of samples, D is the dimension of points
:param affine: an affine matrix with size (D, D)
:param translation: a translation vector with size (1, D)
:param responsibility: the responsibility matrix with size (N, M)
:return:
the variance of each Gaussian distribution, a float
"""
# Transform xs using the affine matrix and translation vector
xs_transformed = xs.dot(affine) + translation
# Compute the pairwise distance matrix between xs_transformed and ys
distance_matrix = np.linalg.norm(xs_transformed[:, np.newaxis, :] - ys[np.newaxis, :, :], axis=2)
# Compute the weighted sum of squared distances for each Gaussian
weighted_distances = distance_matrix**2 * responsibility
sum_weighted_distances = np.sum(weighted_distances, axis=(0, 1))
# Compute the total weight of all the points
total_weight = np.sum(responsibility)
# Compute the variance as the weighted average of the squared distances
variance = sum_weighted_distances / total_weight
return variance
```
相关推荐
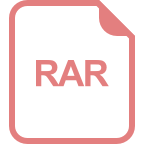
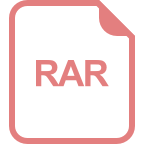















