from sklearn.ensemble import AdaBoostRegressor from sklearn.tree import DecisionTreeRegressor from sklearn.linear_model import LinearRegression from sklearn.ensemble import RandomForestRegressor from sklearn.model_selection import train_test_split import numpy as np import pandas as pd import matplotlib.pyplot as plt from sklearn.metrics import mean_squared_error as MSE from sklearn.metrics import mean_absolute_error as MAE # 从CSV文件中读取数据 data = pd.read_excel('battery.xlsx') # 分离X和y X = data.iloc[:, :-1].values y = data.iloc[:, -1].values X_train, X_test, y_train, y_test = train_test_split(X, y, test_size=0.2, random_state=0) # 定义基础模型 linear_model = LinearRegression() decision_tree_model = DecisionTreeRegressor(max_depth=5) random_forest_model = RandomForestRegressor(n_estimators=100, max_depth=30, random_state=42) base_model = [linear_model, decision_tree_model, random_forest_model] # 定义AdaBoost回归器 ada_boost = AdaBoostRegressor(base_estimator=DecisionTreeRegressor(max_depth=5), n_estimators=100, learning_rate=0.1, random_state=42) # 训练模型 ada_boost.fit(X_train, y_train) # 预测并计算均方误差 y_pred = ada_boost.predict(X_test) print("MAE:", MAE(y_pred, y_test)) print("MSE:", MSE(y_pred, y_test)) print("RMSE:", np.sqrt(MSE(y_pred, y_test))) print("训练集R^2:", ada_boost.score(X_train, y_train)) print("测试集R^2:", ada_boost.score(X_test, y_test)) # 评估预测结果 plt.figure() plt.plot(range(len(y_pred)), y_pred, 'b', label = 'predict') plt.plot(range(len(y_pred)), y_test, 'r', label = 'test') plt.legend(loc = 'upper right') plt.ylabel("SOH") plt.show() 请告诉我这个代码是什么意思
时间: 2024-02-23 15:57:34 浏览: 146
这段代码是一个使用AdaBoost回归器进行电池SOH(State of Health)预测的示例代码。首先,通过pandas库读取一个名为'battery.xlsx'的Excel文件中的数据,并将其分为X和y两个部分。然后,将数据集拆分为训练集和测试集,其中测试集占总数据集的20%。接着,定义了3个基础模型:线性回归模型、决策树回归模型和随机森林回归模型,并将它们存储在一个列表中作为基础模型。然后,使用DecisionTreeRegressor作为基础模型,定义了一个AdaBoost回归器,其中n_estimators指定了基础模型的数量,learning_rate指定了每个基础模型在集成中的权重。接着,使用训练集对AdaBoost回归器进行训练,并使用测试集进行预测和评估。最后,通过Matplotlib绘制了预测结果和测试结果的比较图。
相关问题
from sklearn.tree import DecisionTreeClassifier from sklearn.linear_model import LogisticRegression from sklearn.svm import SVC from sklearn.ensemble import VotingClassifier seed = 7 # your implementation here
好的,这段代码是用来实现集成学习中的投票分类器(Voting Classifier)的。首先,我们导入了四个不同的分类器:决策树分类器(DecisionTreeClassifier)、逻辑回归分类器(LogisticRegression)、支持向量机分类器(SVC)和集成分类器(VotingClassifier)。
接下来,我们定义了一个种子数(seed),用于控制随机数生成器的行为,以确保我们的结果是可重复的。
然后,我们可以使用这些分类器来构建一个投票分类器,即使用集成学习的思想将多个分类器的预测结果进行投票来决定最终的预测结果。具体来说,我们可以使用 VotingClassifier 类来构建投票分类器,然后将这些分类器传递给它的 estimators 参数。
例如,下面的代码实例化了一个投票分类器,其中包含了上述四个分类器:
```
voting_clf = VotingClassifier(estimators=[('dt', DecisionTreeClassifier()),
('lr', LogisticRegression()),
('svc', SVC()),
('vc', VotingClassifier(estimators=[('dt', DecisionTreeClassifier()),
('lr', LogisticRegression()),
('svc', SVC())],
voting='hard'))],
voting='hard')
```
在上面的代码中,我们首先定义了一个 VotingClassifier 对象,其中包含了四个分类器。其中第一个参数 estimators 是一个列表,其中的每个元素都是一个二元组,包含了分类器的名称和分类器本身。注意,最后一个分类器是另一个投票分类器,用于实现递归投票的效果。
最后,我们可以使用 fit 方法来拟合投票分类器,使用 predict 方法来进行预测。
在Python中想运行from sklearn.model_selection import train_test_split from sklearn.decomposition import PCA import pandas as pd from sklearn import svm import numpy as np import math import matplotlib.pyplot as plt import matplotlib as mpl from matplotlib import colors from sklearn.model_selection import train_test_split from sklearn import datasets from matplotlib.colors import ListedColormap from sklearn.svm import SVC from sklearn.preprocessing import StandardScaler from sklearn.model_selection import StratifiedShuffleSplit,StratifiedKFold from sklearn.model_selection import GridSearchCV from sklearn.model_selection import GridSearchCV, LeaveOneOut, cross_val_predict from sklearn.model_selection import KFold from sklearn.linear_model import LogisticRegression from sklearn.naive_bayes import GaussianNB from sklearn.neighbors import KNeighborsClassifier from sklearn import svm from sklearn.tree import DecisionTreeClassifier from sklearn.ensemble import RandomForestClassifier from sklearn.metrics import roc_auc_score from sklearn.metrics import roc_auc_score import math import datetime import multiprocessing as mp from sklearn.ensemble import StackingClassifier from sklearn.pipeline import make_pipeline from sklearn.svm import LinearSVC import random怎么操作
要在Python中运行这些代码,你需要确保已经安装了相应的包。大多数包都可以使用`pip`进行安装。
首先,在命令行中运行以下命令来安装所需的包:
```bash
pip install scikit-learn pandas matplotlib numpy
```
然后,在你的Python脚本中导入所需的库:
```python
from sklearn.model_selection import train_test_split
from sklearn.decomposition import PCA
import pandas as pd
from sklearn import svm
import numpy as np
import math
import matplotlib.pyplot as plt
import matplotlib as mpl
from matplotlib import colors
from sklearn.model_selection import train_test_split
from sklearn import datasets
from matplotlib.colors import ListedColormap
from sklearn.svm import SVC
from sklearn.preprocessing import StandardScaler
from sklearn.model_selection import StratifiedShuffleSplit, StratifiedKFold
from sklearn.model_selection import GridSearchCV
from sklearn.model_selection import GridSearchCV, LeaveOneOut, cross_val_predict
from sklearn.model_selection import KFold
from sklearn.linear_model import LogisticRegression
from sklearn.naive_bayes import GaussianNB
from sklearn.neighbors import KNeighborsClassifier
from sklearn.tree import DecisionTreeClassifier
from sklearn.ensemble import RandomForestClassifier
from sklearn.metrics import roc_auc_score
import datetime
import multiprocessing as mp
from sklearn.ensemble import StackingClassifier
from sklearn.pipeline import make_pipeline
from sklearn.svm import LinearSVC
import random
```
请确保在运行这些代码之前,已经安装了所需的Python库。如果遇到任何问题,请确保已正确安装这些库,并且版本与代码兼容。
阅读全文
相关推荐
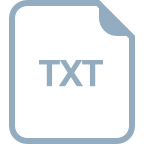
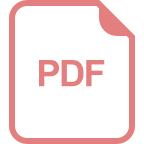
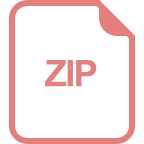













