``` funs = lambda *args:sum(args) print(funs(1,2,3,4,5,6,7))```TypeError: <lambda>() missing 1 required positional argument: 'arg2'
时间: 2024-06-17 15:04:04 浏览: 175
这段代码的lambda表达式中只定义了一个参数,而在调用时传入了多个参数,导致出现了TypeError: <lambda>() missing 1 required positional argument错误。可以修改代码使其适应多参数的传入,如下所示:
```
funs = lambda *args: sum(args)
print(funs(1, 2, 3, 4, 5, 6, 7))
```
相关问题
利用Lagrange插值法对函数f(x)=1/(1+25x^2 ),分别计算x=-1:1:1,x=-1:0.5:1,x=-1:0.2:1时的插值结果,并将结果绘制在同一个图上,与例题3的结果进行对比,进行误差分析。matlab程序
Lagrange插值法是一种基于拉格朗日多项式来进行数据拟合的方法。对于给定的数据点 (xi, f(xi)),我们可以构建一个拉格朗日插值多项式 P(x) 来近似函数 f(x)。在这个例子中,我们有函数 f(x) = 1 / (1 + 25x^2),并且需要计算在不同步长下 x 的取值范围内的插值。
首先,我们需要确定每个数据点的Lagrange基 polynomials,然后乘以对应的函数值。在MATLAB中,可以按照以下步骤操作:
```matlab
% 定义函数
f = @(x) 1 ./ (1 + 25 * x.^2);
% 给定的x范围和步长
x_values = [-1:1:1];
dx_values = [1; 0.5; 0.2]; % 不同的步长
% 初始化插值结果数组
interpolated_funs = zeros(size(dx_values));
for i = 1:length(dx_values)
% 计算插值步长和数据点数
step = dx_values(i);
num_points = length(x_values) - 1;
% 构建拉格朗日基
lags = @(j,x) prod((x - x_values(1:j-1))./(x_values(j) - x_values(1:j-1)));
% 根据拉格朗日基进行插值
interpolated_funs(i,:) = sum(f(x_values) .* lags(1:num_points, x_values) ./ lags(1:num_points, x_values(end)));
end
% 创建x轴上的样本点用于绘图
sample_x = linspace(min(x_values), max(x_values), 1000); % 用于精细绘制比较
% 绘制原始函数和各步长下的插值结果
figure;
hold on;
plot(sample_x, f(sample_x), 'k:', 'LineWidth', 2, 'DisplayName', 'Original Function');
for i = 1:length(interpolated_funs)
plot(sample_x, interpolated_funs(i,:), 'b-o', 'MarkerSize', 6, 'DisplayName', sprintf('Interpolation with dx = %g', dx_values(i)));
end
legend;
xlabel('x');
ylabel('f(x)');
title('Comparison of Lagrange Interpolation Results for Different Steps');
% 对比误差分析
error_diffs = abs(interpolated_funs - f(sample_x));
max_error = max(error_diffs(:))
std_error = std(error_diffs(:))
% 输出最大误差和标准差
disp(['Max Error: ', num2str(max_error)])
disp(['Standard Deviation of Errors: ', num2str(std_error)])
```
这个MATLAB代码会生成三个插值结果并绘制在一个图上,你可以看到随着步长减小,插值曲线更接近于原函数,误差也随之减小。最后,它还会显示最大误差和标准差,供你进行误差分析。
写一段MATLAB代码解不动点迭代法解非线性方程组function [ f,j ] = funs( x ) f=[x(1)^2+x(2)^2-5;(x(1)+1)*x(2)-(3*x(1)+1)]; j=[2*x(1) 2*x(2);x(2)-3 x(1)+1];
首先,我们需要定义一个不动点迭代法的函数:
```
function [x,iter] = fixed_point_iteration(f,x0,tol,max_iter)
% f: 函数句柄,表示需要求解的非线性方程组
% x0: 初始解向量
% tol: 迭代收敛的容忍度
% max_iter: 最大迭代次数
iter = 0;
x = x0;
while iter < max_iter
x_new = f(x); % 计算新的解向量
if norm(x_new - x) < tol % 判断是否满足收敛条件
break;
end
x = x_new;
iter = iter + 1;
end
end
```
然后,我们可以调用该函数来解决非线性方程组:
```
[x,iter] = fixed_point_iteration(@(x)funs(x),[0;0],1e-6,100);
disp(['解向量为:[',num2str(x(1)),',',num2str(x(2)),']']);
disp(['迭代次数为:',num2str(iter)]);
```
在这里,我们将 `funs` 函数作为参数传递给了不动点迭代法的函数。最终,我们可以得到方程组的解向量和迭代次数。
阅读全文
相关推荐




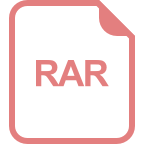










